A Continuous Random Variable May Assume What Value
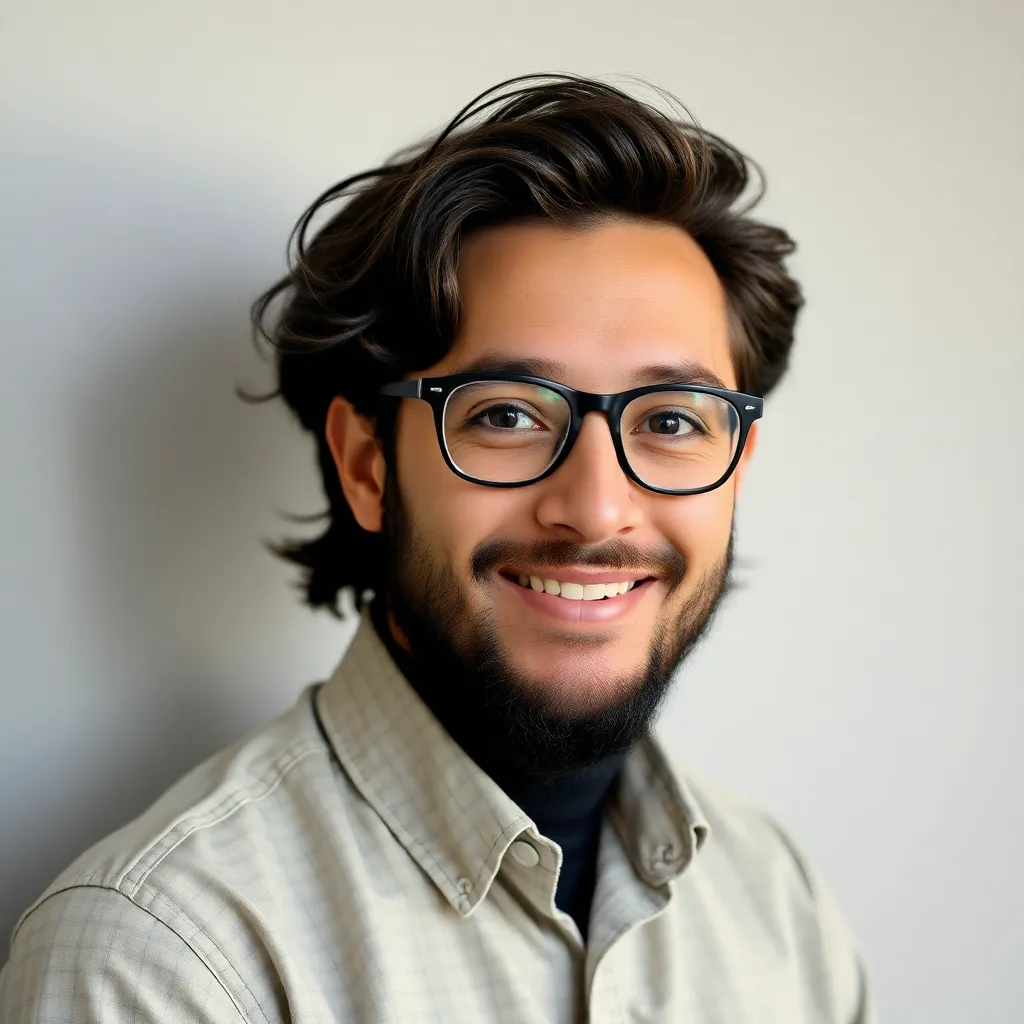
Kalali
Apr 13, 2025 · 6 min read
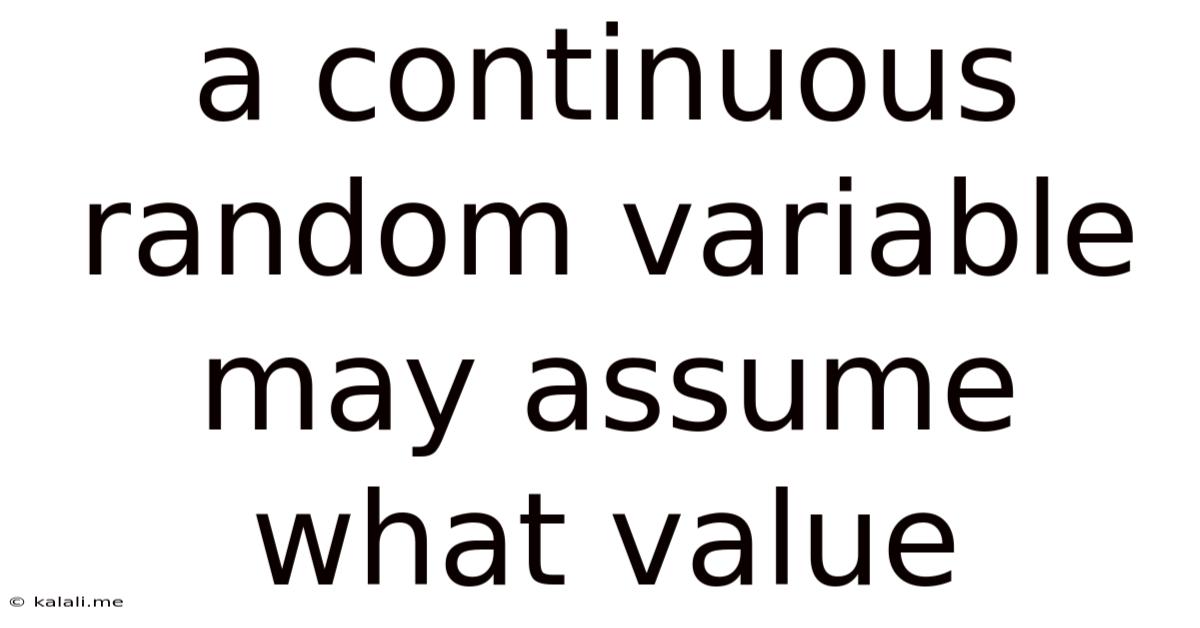
Table of Contents
A Continuous Random Variable: Exploring the Universe of Possible Values
A continuous random variable is a cornerstone of probability and statistics, representing quantities that can take on any value within a given range. Unlike discrete random variables, which can only assume specific, separate values (like the number of heads in three coin flips), continuous variables can be anywhere within an interval. This seemingly simple difference opens up a vast landscape of possibilities and complexities. Understanding what values a continuous random variable may assume requires delving into its underlying probability density function (PDF) and cumulative distribution function (CDF). This article explores these concepts, illustrating them with examples and demonstrating their practical applications.
What Defines a Continuous Random Variable?
The key characteristic of a continuous random variable is its ability to assume any value within a specified range. This range can be bounded (e.g., the height of a student in a class, typically between 4 feet and 7 feet) or unbounded (e.g., the speed of a molecule, theoretically ranging from zero to infinity). The probability of a continuous random variable taking on any single specific value is always zero. This may seem counterintuitive at first, but it’s a direct consequence of the infinite number of possible values within even a small interval. Instead of considering individual point probabilities, we examine the probability that the variable falls within a range of values.
The Probability Density Function (PDF): Unveiling the Distribution
The behavior of a continuous random variable is fully described by its probability density function, denoted as f(x). The PDF isn't a probability itself; rather, it represents the relative likelihood of the variable taking on a value near x. The crucial property of the PDF is that the integral of f(x) over a given interval gives the probability that the variable falls within that interval. Mathematically:
P(a ≤ X ≤ b) = ∫<sub>a</sub><sup>b</sup> f(x) dx
where:
- P(a ≤ X ≤ b) is the probability that the random variable X lies between a and b.
- f(x) is the probability density function.
- ∫<sub>a</sub><sup>b</sup> denotes the definite integral from a to b.
This integral represents the area under the curve of the PDF between points a and b. The total area under the entire PDF curve must always equal 1, reflecting the certainty that the variable will take on some value within its range.
The Cumulative Distribution Function (CDF): Accumulating Probabilities
The cumulative distribution function (CDF), denoted as F(x), provides a different, yet equally crucial perspective. The CDF represents the probability that the random variable X is less than or equal to a particular value x:
F(x) = P(X ≤ x) = ∫<sub>-∞</sub><sup>x</sup> f(t) dt
The CDF provides the accumulated probability up to a given point. It’s a non-decreasing function, ranging from 0 to 1. Knowing the CDF allows for easy calculation of probabilities for various intervals:
P(a ≤ X ≤ b) = F(b) - F(a)
This simply states that the probability of X being between a and b is the difference between the accumulated probabilities up to b and up to a.
Examples of Continuous Random Variables and Their PDFs:
Several common distributions describe continuous random variables, each with its unique PDF and properties:
-
Uniform Distribution: This represents a variable with equal probability of taking on any value within a specified interval [a, b]. Its PDF is a constant within this interval and zero elsewhere:
f(x) = 1/(b-a) for a ≤ x ≤ b, and 0 otherwise.
-
Exponential Distribution: Often used to model waiting times or the lifespan of components, its PDF is:
f(x) = λe<sup>-λx</sup> for x ≥ 0, and 0 otherwise, where λ is a rate parameter.
-
Normal Distribution (Gaussian Distribution): This ubiquitous distribution describes many natural phenomena, characterized by its bell-shaped curve. Its PDF is more complex:
f(x) = (1/√(2πσ²)) * e<sup>-((x-μ)²/(2σ²))</sup>, where μ is the mean and σ is the standard deviation.
-
Gamma Distribution: A versatile distribution with applications in various fields, including reliability analysis and queuing theory. Its PDF depends on two parameters, shape (k) and scale (θ).
-
Beta Distribution: Often used to model probabilities or proportions, defined on the interval [0, 1]. Its PDF is described by two shape parameters, α and β.
Illustrative Examples:
Let's consider some practical examples to solidify our understanding:
Example 1: The Height of Students
Suppose the heights of students in a college follow a normal distribution with a mean of 68 inches and a standard deviation of 3 inches. To find the probability that a randomly selected student is taller than 71 inches, we would use the normal distribution's CDF. We would find the area under the curve to the right of 71 inches. This calculation typically involves using statistical tables or software.
Example 2: Time Until Equipment Failure
Assume the time until a machine fails follows an exponential distribution with a failure rate (λ) of 0.1 per year. To determine the probability that the machine will operate for more than 5 years, we’d calculate the integral of the exponential PDF from 5 to infinity. This probability represents the reliability of the machine over that period.
Example 3: Modeling Rainfall
The amount of rainfall in a specific region during a month could be modeled using a Gamma distribution. The shape and scale parameters would be estimated from historical rainfall data. We can then use the CDF to determine probabilities for various rainfall amounts, aiding in water resource management and agricultural planning.
Addressing the "May Assume" Question:
Returning to the central question of what values a continuous random variable may assume, the answer is: any value within its defined range, with a probability of zero for any single point. The crucial aspect is that the probability is distributed across this range, as described by the PDF. The PDF provides the relative likelihood of the variable being near any given value, while the CDF gives the cumulative probability up to a certain point. The range itself is determined by the specific distribution and its parameters. For unbounded distributions like the exponential distribution, the range extends infinitely. For bounded distributions like the uniform distribution, the range is clearly defined by its lower and upper bounds. Understanding these concepts is essential for correctly interpreting and utilizing continuous random variables in diverse applications.
Beyond the Basics: Applications and Advanced Concepts
The applications of continuous random variables are extensive and pervasive across numerous fields:
- Engineering: Reliability analysis, queuing theory, signal processing.
- Physics: Modeling physical phenomena, statistical mechanics.
- Finance: Option pricing, risk management, portfolio optimization.
- Medicine: Clinical trials, epidemiological studies.
- Environmental Science: Modeling weather patterns, pollution levels.
Advanced concepts related to continuous random variables include:
- Joint distributions: Describing the relationship between multiple continuous random variables.
- Conditional distributions: Examining the probability of one variable given the value of another.
- Moment generating functions: A powerful tool for calculating moments (mean, variance, etc.) of a distribution.
- Central Limit Theorem: A fundamental result stating that the sum of many independent random variables tends towards a normal distribution.
Mastering the concepts of continuous random variables, including their PDFs and CDFs, is fundamental for anyone working with probabilistic models and statistical analysis. The ability to understand and apply these concepts unlocks a deeper understanding of the world around us, offering powerful tools for modeling, prediction, and decision-making in countless fields. The seemingly simple question of what values a continuous random variable "may assume" leads to a rich tapestry of mathematical concepts and practical applications.
Latest Posts
Latest Posts
-
How Do I Send An Evite Reminder
Jul 15, 2025
-
When Performing A Self Rescue When Should You Swim To Shore
Jul 15, 2025
-
How Many Decaliters Are In A Liter
Jul 15, 2025
-
What Note Sits In The Middle Of The Grand Staff
Jul 15, 2025
-
Did Lynette Shave Her Head In Real Life
Jul 15, 2025
Related Post
Thank you for visiting our website which covers about A Continuous Random Variable May Assume What Value . We hope the information provided has been useful to you. Feel free to contact us if you have any questions or need further assistance. See you next time and don't miss to bookmark.