Asymptotic Distribution Of Likelihood Ratio Test Statistic
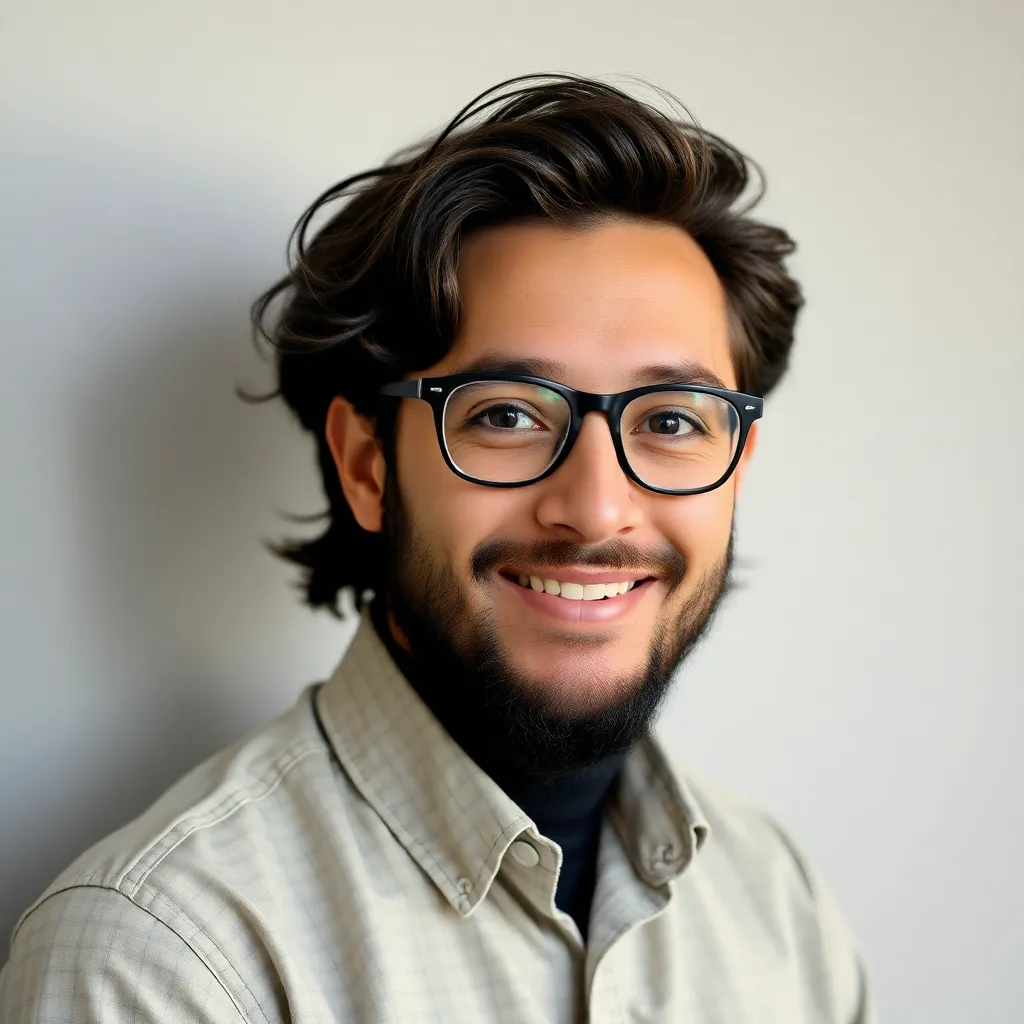
Kalali
May 23, 2025 · 3 min read
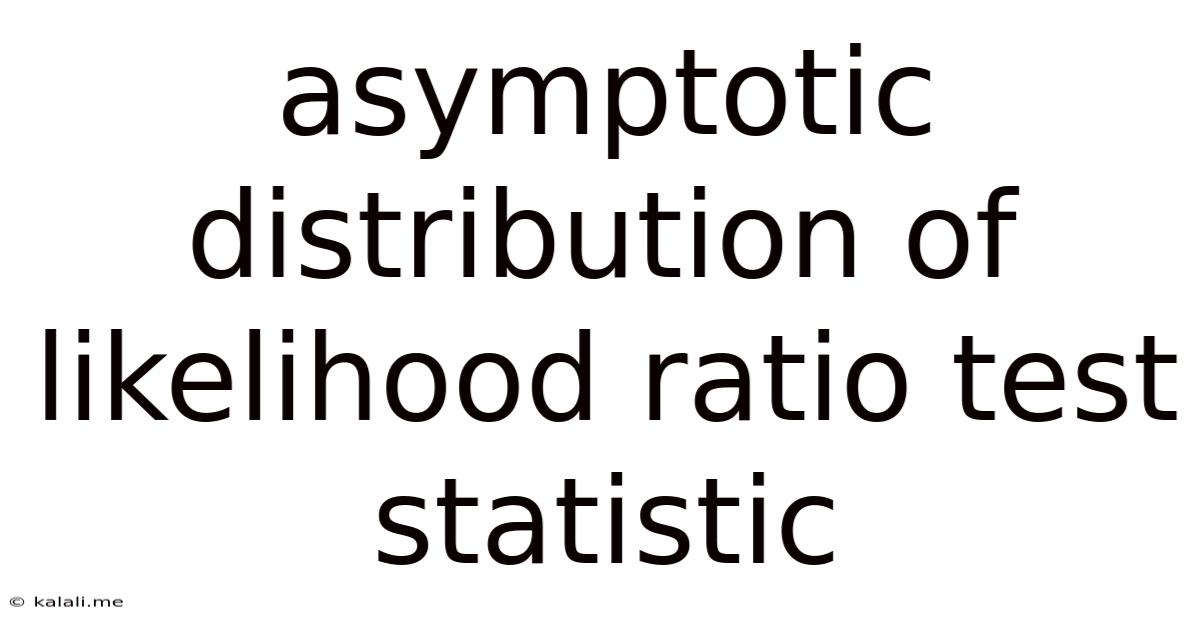
Table of Contents
Asymptotic Distribution of the Likelihood Ratio Test Statistic
This article explores the asymptotic distribution of the likelihood ratio test (LRT) statistic, a crucial concept in statistical hypothesis testing. Understanding its asymptotic behavior allows us to determine the significance of test results, even when the exact distribution is unknown or computationally intractable. We'll delve into the theoretical underpinnings and practical applications of this powerful tool.
The likelihood ratio test compares the likelihood of observed data under different statistical models. Specifically, it assesses the plausibility of a null hypothesis (H₀) against an alternative hypothesis (H₁). The test statistic is constructed as the ratio of the maximized likelihood under the null hypothesis to the maximized likelihood under the alternative hypothesis. This ratio, often denoted as λ, is always between 0 and 1. A small value of λ suggests strong evidence against the null hypothesis.
The Wilks' Theorem: Cornerstone of Asymptotic Inference
The key to understanding the LRT's behavior lies in Wilks' Theorem. This fundamental theorem states that under certain regularity conditions, the statistic -2 log λ asymptotically follows a chi-squared (χ²) distribution as the sample size (n) approaches infinity. The degrees of freedom (df) of this chi-squared distribution are equal to the difference in the number of parameters between the alternative and null hypotheses.
This is a remarkably powerful result. It means that regardless of the specific form of the likelihood function, the asymptotic distribution of the LRT statistic is known. This allows us to calculate p-values and make inferences about the hypotheses being tested, even in complex scenarios where the exact distribution of the test statistic is unavailable.
Regularity Conditions for Wilks' Theorem
Wilks' Theorem holds under several crucial regularity conditions. These conditions ensure the validity of the asymptotic approximation:
- Identifiability: The parameters under both the null and alternative hypotheses must be identifiable.
- Differentiability: The likelihood function must be twice continuously differentiable with respect to the parameters.
- Non-singularity: The Fisher information matrix must be non-singular under both hypotheses. This ensures that the parameters are estimable.
- Convergence: The maximum likelihood estimators must converge in probability to their true values.
Violation of these conditions can affect the accuracy of the chi-squared approximation, particularly for small sample sizes. In such cases, alternative approaches or simulations might be necessary.
Applications of the Asymptotic Distribution
The asymptotic distribution of the LRT statistic finds widespread application across various statistical domains, including:
- Model Selection: Choosing between nested statistical models (e.g., comparing a linear regression model to a more complex polynomial model).
- Goodness-of-Fit Tests: Assessing how well a statistical model fits the observed data.
- Testing for Differences in Means: Comparing means across multiple groups (a generalization of the t-test).
- Hypothesis Testing in Generalized Linear Models: Analyzing data with non-normal responses.
Limitations and Considerations
While the asymptotic chi-squared distribution provides a convenient approximation, it's crucial to acknowledge its limitations:
- Sample Size: The approximation improves with increasing sample size. For small samples, the approximation might be inaccurate.
- Regularity Conditions: As mentioned earlier, violating the regularity conditions can invalidate the approximation.
- Nesting: Wilks' Theorem is primarily applicable to nested models. For non-nested models, alternative testing procedures may be required.
Conclusion
The asymptotic distribution of the likelihood ratio test statistic, underpinned by Wilks' Theorem, is a cornerstone of modern statistical inference. Understanding its properties and limitations is essential for correctly interpreting the results of hypothesis tests, particularly when dealing with complex models or situations where the exact distribution is intractable. However, remembering the necessary regularity conditions and considering potential limitations, especially with small sample sizes, is vital for accurate and reliable statistical analysis.
Latest Posts
Latest Posts
-
How To Get Oil Off Driveway
May 24, 2025
-
How Long Can Tuna Salad Last In The Fridge
May 24, 2025
-
How To Get More Dwellers In Fallout Shelter
May 24, 2025
-
How To Install A Tankless Water Heater
May 24, 2025
-
No One Knows The Day Or The Hour
May 24, 2025
Related Post
Thank you for visiting our website which covers about Asymptotic Distribution Of Likelihood Ratio Test Statistic . We hope the information provided has been useful to you. Feel free to contact us if you have any questions or need further assistance. See you next time and don't miss to bookmark.