Average Treatment Effect On The Treated
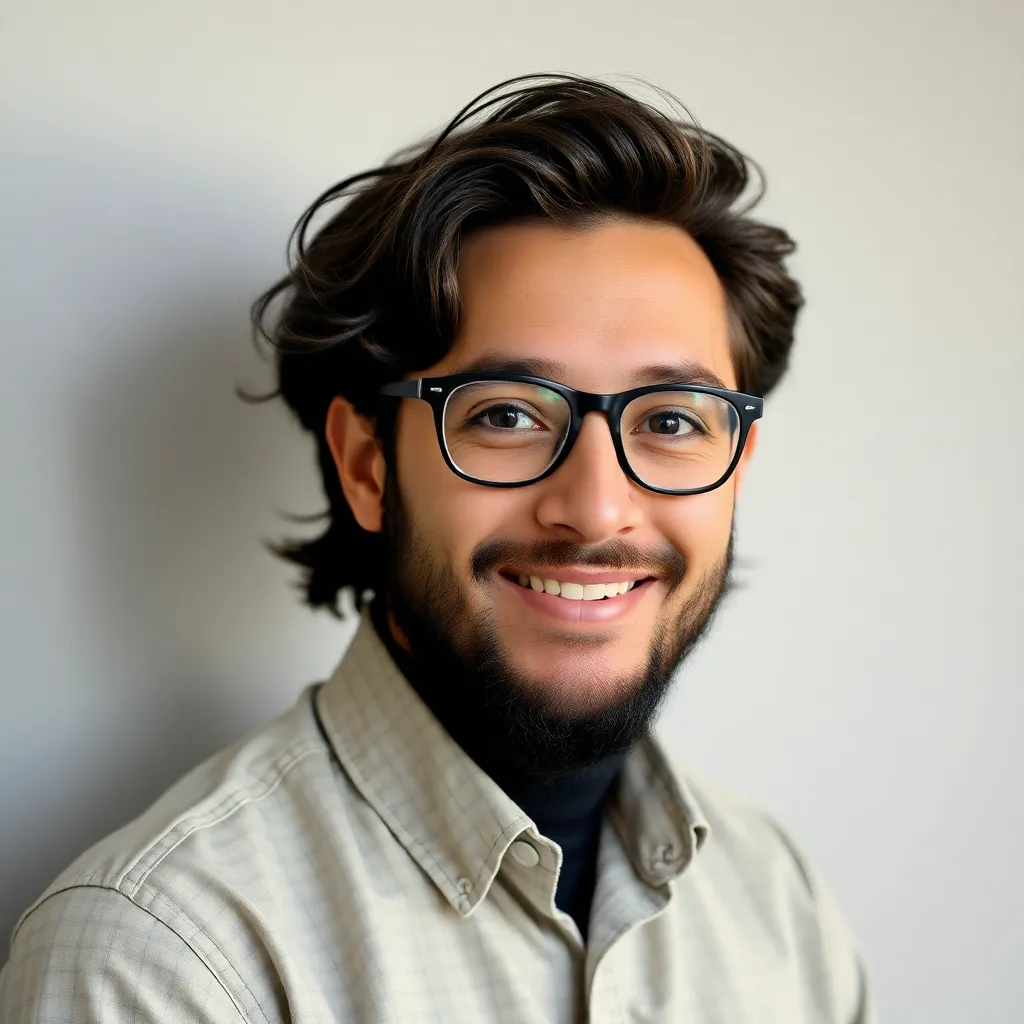
Kalali
May 19, 2025 · 4 min read
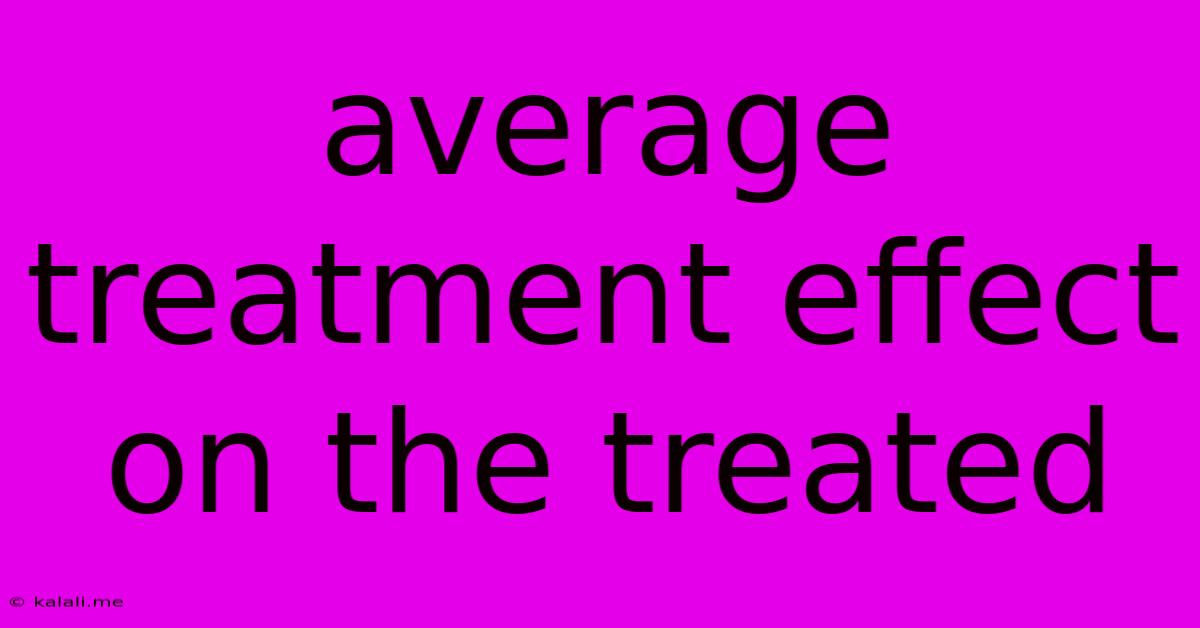
Table of Contents
Understanding the Average Treatment Effect on the Treated (ATT)
Meta Description: Learn about the Average Treatment Effect on the Treated (ATT), a crucial causal inference metric. This guide explains ATT's calculation, interpretation, and application in various fields, clarifying its differences from ATE.
Estimating the causal impact of an intervention is a fundamental goal in many fields, from healthcare to economics. While the Average Treatment Effect (ATE) measures the overall average treatment effect across the entire population, the Average Treatment Effect on the Treated (ATT) focuses specifically on the individuals who actually received the treatment. This distinction is crucial, particularly when dealing with situations where treatment assignment isn't random. This article will delve into the intricacies of ATT, exploring its calculation, interpretation, and its advantages over ATE in specific scenarios.
What is the Average Treatment Effect on the Treated (ATT)?
The ATT measures the average difference in outcomes between those who received the treatment and those who would have received the treatment had they been assigned to the treatment group. This "would have" component highlights the inherent counterfactual nature of causal inference. We're trying to determine what would have happened to the treated group if they hadn't received the treatment. This is where statistical techniques, such as regression discontinuity design or propensity score matching, become essential to estimate this counterfactual outcome.
In simpler terms, ATT answers the question: "What was the average impact of the treatment on those who actually received it?"
Calculating ATT
Calculating ATT typically involves comparing the observed outcomes of the treated group with an estimated outcome had they not received the treatment. This estimation often relies on a control group, but the selection of the control group must be carefully considered to mitigate selection bias. Common methods include:
- Regression Adjustment: This involves regressing the outcome variable on treatment status and other relevant covariates. The ATT is then estimated from the regression coefficients.
- Propensity Score Matching: This technique matches treated individuals with control individuals based on their propensity score (the probability of receiving treatment given their observed characteristics). The ATT is then estimated by comparing the outcomes of the matched pairs.
- Instrumental Variables: When random assignment isn't feasible, instrumental variables can be used to identify the causal effect. An instrument is a variable that affects treatment assignment but doesn't directly influence the outcome, except through its effect on treatment.
ATT vs. ATE: Key Differences
While both ATT and ATE aim to estimate the causal effect of a treatment, they differ in their scope:
- ATE (Average Treatment Effect): Measures the average treatment effect across the entire population, including those who received and did not receive the treatment.
- ATT (Average Treatment Effect on the Treated): Measures the average treatment effect specifically on those who received the treatment.
The choice between ATT and ATE depends on the research question. If the goal is to understand the overall impact of a treatment on a population, ATE is appropriate. However, if the interest lies in evaluating the effectiveness of a treatment program on those who actually participated, ATT is the more relevant metric. For example, evaluating the effectiveness of a job training program would likely focus on ATT, as the primary concern is the impact on those who completed the program.
Interpreting and Applying ATT
The interpretation of ATT is straightforward: it represents the average causal effect of the treatment on the treated individuals. A positive ATT indicates that the treatment had a positive average effect on those who received it, while a negative ATT suggests a negative effect.
ATT finds application across diverse fields:
- Public Health: Evaluating the effectiveness of a new vaccine or medication.
- Economics: Assessing the impact of a job training program or a policy intervention.
- Education: Measuring the effect of a new teaching method on student outcomes.
- Marketing: Determining the effectiveness of an advertising campaign on sales.
Conclusion
The Average Treatment Effect on the Treated (ATT) is a powerful tool for causal inference, providing valuable insights into the impact of interventions on those who actually receive them. Understanding its calculation, interpretation, and differences from ATE is crucial for researchers across various disciplines aiming to draw reliable causal conclusions from observational data. Choosing between ATT and ATE hinges on the specific research question and the characteristics of the data at hand. Remember that careful consideration of potential biases and the selection of appropriate statistical methods are paramount for accurate estimation of ATT.
Latest Posts
Latest Posts
-
What Did Voldemort Look Like Before He Died
May 19, 2025
-
Call Us The Moment You Finish The Job
May 19, 2025
-
Can You Drink The Water In Spain
May 19, 2025
-
Is Dying Your Hair Black Haram
May 19, 2025
-
How Long Will Keg Beer Last
May 19, 2025
Related Post
Thank you for visiting our website which covers about Average Treatment Effect On The Treated . We hope the information provided has been useful to you. Feel free to contact us if you have any questions or need further assistance. See you next time and don't miss to bookmark.