Complete Statistics How To Prove Parameter Space Contain Open Set
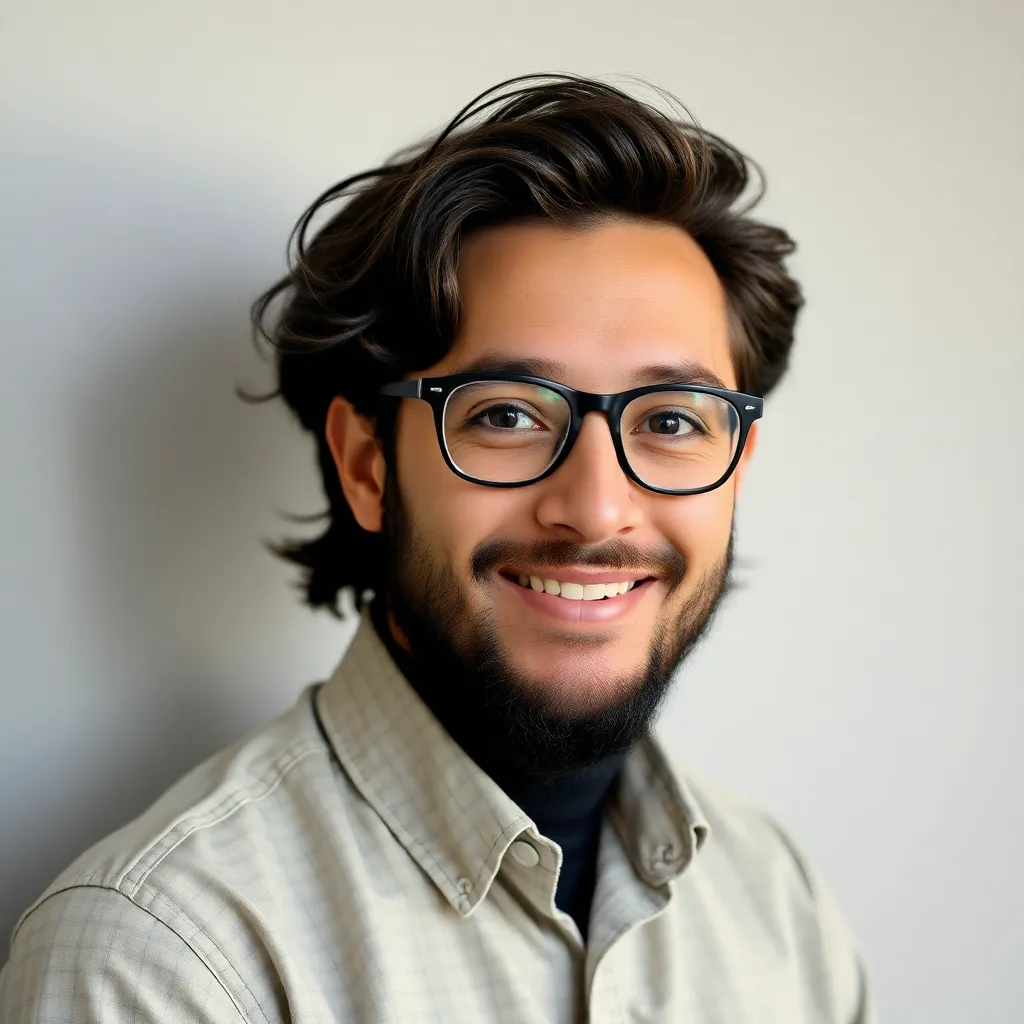
Kalali
May 24, 2025 · 4 min read
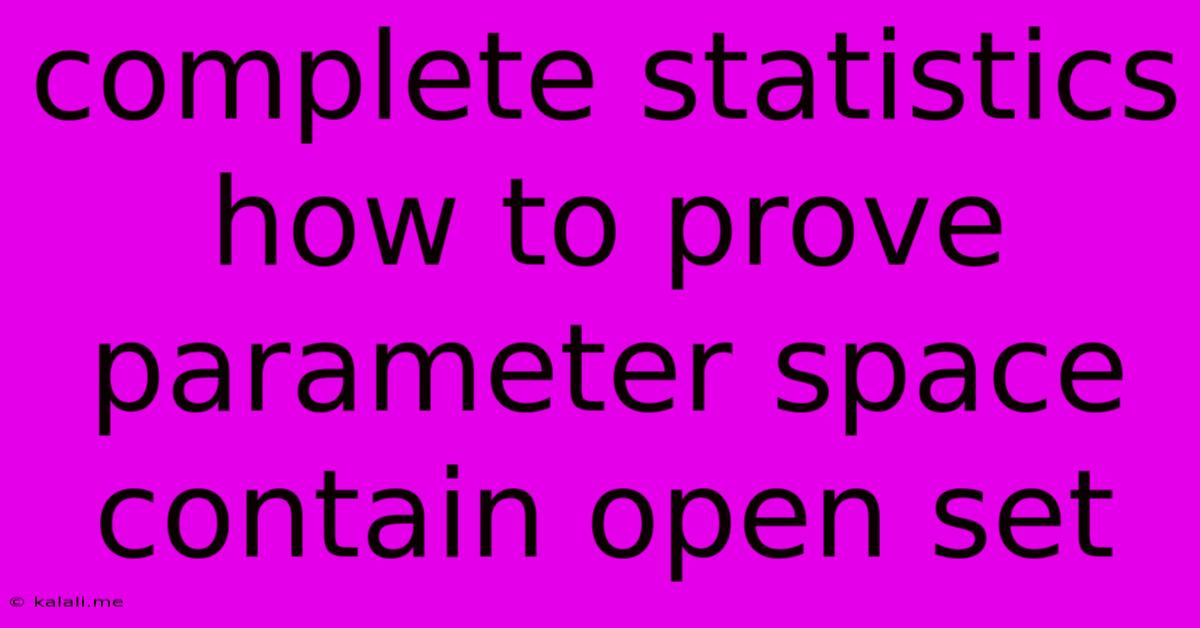
Table of Contents
Proving a Parameter Space Contains an Open Set: A Statistical Approach
This article explores how to statistically demonstrate that a parameter space contains an open set. This is a crucial concept in various statistical fields, particularly in maximum likelihood estimation and hypothesis testing, where the existence of an open set around the true parameter value is often a necessary condition for desirable properties like consistency and asymptotic normality of estimators. We'll delve into the theoretical underpinnings and practical methods to provide compelling evidence.
What is an Open Set in a Parameter Space?
Before tackling the statistical proof, let's clarify the concept. A parameter space, denoted by Θ, is the set of all possible values for a statistical parameter (e.g., the mean of a normal distribution, the regression coefficients in a linear model). An open set within Θ, say Θ<sub>0</sub> ⊂ Θ, is a set where for every point θ ∈ Θ<sub>0</sub>, there exists a small neighborhood (a ball of some radius ε > 0) entirely contained within Θ<sub>0</sub>. In simpler terms, you can find a small region around any point in the open set that is still entirely within the parameter space. This absence of boundary points is essential for many statistical theorems.
Why Proving the Existence of an Open Set Matters
Demonstrating that the parameter space contains an open set around the true parameter value is vital for several reasons:
- Consistency of Estimators: Many estimators, such as maximum likelihood estimators (MLEs), are only guaranteed to be consistent (converging to the true parameter value as the sample size increases) if the true parameter lies in the interior of an open set within the parameter space.
- Asymptotic Normality: The asymptotic normality of estimators, a key property allowing for the construction of confidence intervals and hypothesis tests, often relies on the true parameter being in an open set where regularity conditions are satisfied.
- Invertibility of Information Matrix: The Fisher information matrix, crucial for assessing estimator efficiency, often requires invertibility, which frequently hinges on the true parameter residing within an open set.
Statistical Methods to Demonstrate the Existence of an Open Set
Directly proving the existence of an open set can be challenging. Instead, we often focus on demonstrating conditions that imply the existence of such a set. This usually involves leveraging properties of the likelihood function or other relevant statistical quantities. Here are some common approaches:
1. Regularity Conditions: Many statistical theorems rely on regularity conditions related to the likelihood function (or other relevant functions like the log-likelihood). These conditions implicitly guarantee the existence of an open set. These conditions often involve:
- Differentiability: The likelihood function is sufficiently differentiable within a neighborhood of the true parameter.
- Identifiability: The parameter is uniquely identifiable; different parameter values lead to distinct likelihood functions.
- Non-singularity of the Information Matrix: The Fisher information matrix is positive definite at the true parameter value. This implies that the likelihood function is well-behaved and has a unique maximum within a region around the true parameter.
Satisfying these regularity conditions strongly suggests that the parameter space contains an open set around the true parameter, though it’s not a direct proof.
2. Empirical Analysis of the Likelihood Function: In practice, we often analyze the likelihood function (or its logarithm) numerically. Plotting the likelihood surface can provide visual evidence of a well-defined maximum surrounded by a region where the likelihood is high. This, along with the satisfaction of the regularity conditions, strengthens the argument for the existence of an open set.
3. Bootstrapping: Bootstrapping can help assess the variability of the estimator and provide indirect evidence for the existence of an open set. If the bootstrap distribution is well-behaved and concentrated around a consistent estimator, it supports the idea that the true parameter resides within an open set where the estimator functions appropriately.
Conclusion:
Proving that a parameter space contains an open set is crucial for the validity of many statistical inference methods. Although a direct proof may be complex, demonstrating the satisfaction of regularity conditions, analyzing the likelihood function, or using bootstrapping techniques provides strong statistical evidence supporting the existence of such an open set, bolstering the reliability and validity of the statistical conclusions drawn. Remember that rigorous mathematical proofs often require specialized knowledge and are context-specific. The approaches described here offer practical strategies for demonstrating the necessary conditions in various statistical modeling scenarios.
Latest Posts
Latest Posts
-
How Long Is Thawed Chicken Good For
May 24, 2025
-
How Long Can Coffee Sit Out
May 24, 2025
-
What Increases He Amount Of Magnetic Energy Of A Magnet
May 24, 2025
-
How Often Should Spark Plugs Be Replaced
May 24, 2025
-
How To Remove Water Marks From Wood
May 24, 2025
Related Post
Thank you for visiting our website which covers about Complete Statistics How To Prove Parameter Space Contain Open Set . We hope the information provided has been useful to you. Feel free to contact us if you have any questions or need further assistance. See you next time and don't miss to bookmark.