Cosine Of A Normal Random Variable
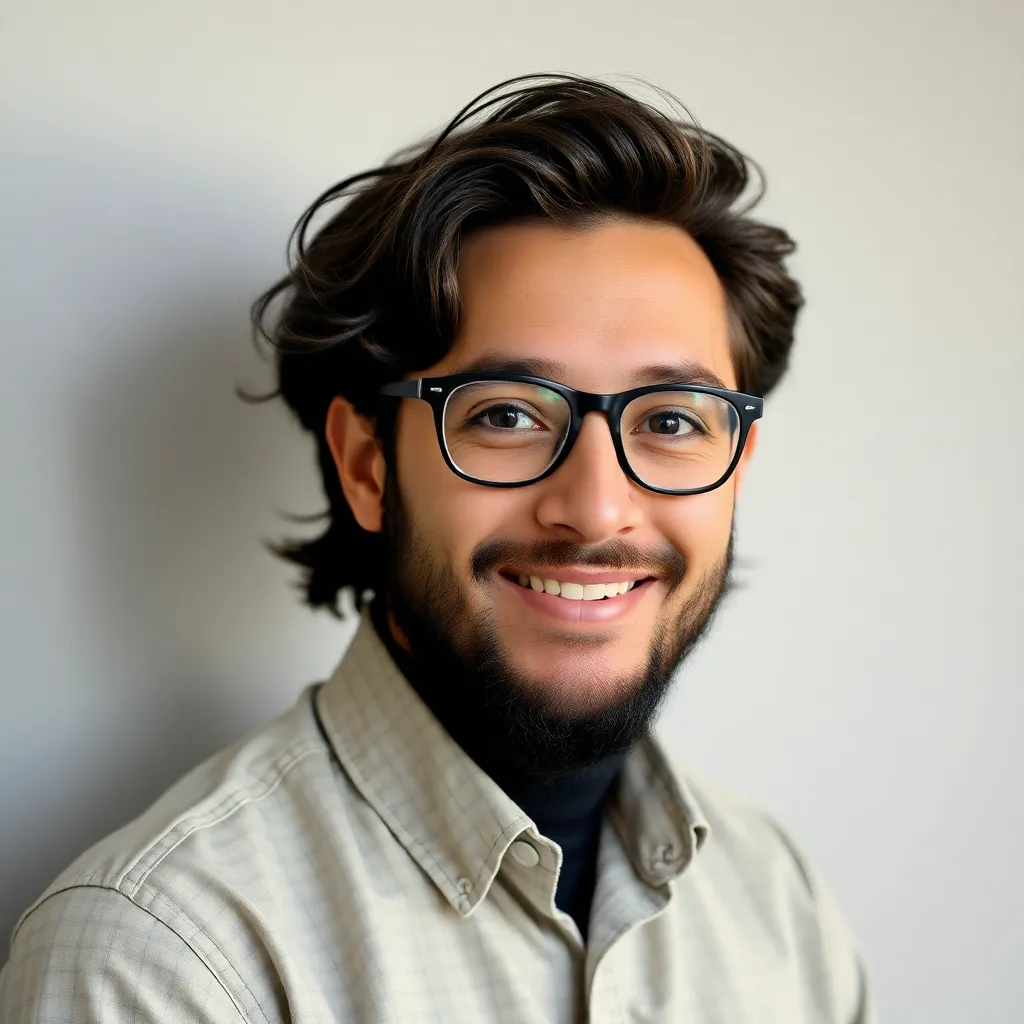
Kalali
May 26, 2025 · 3 min read
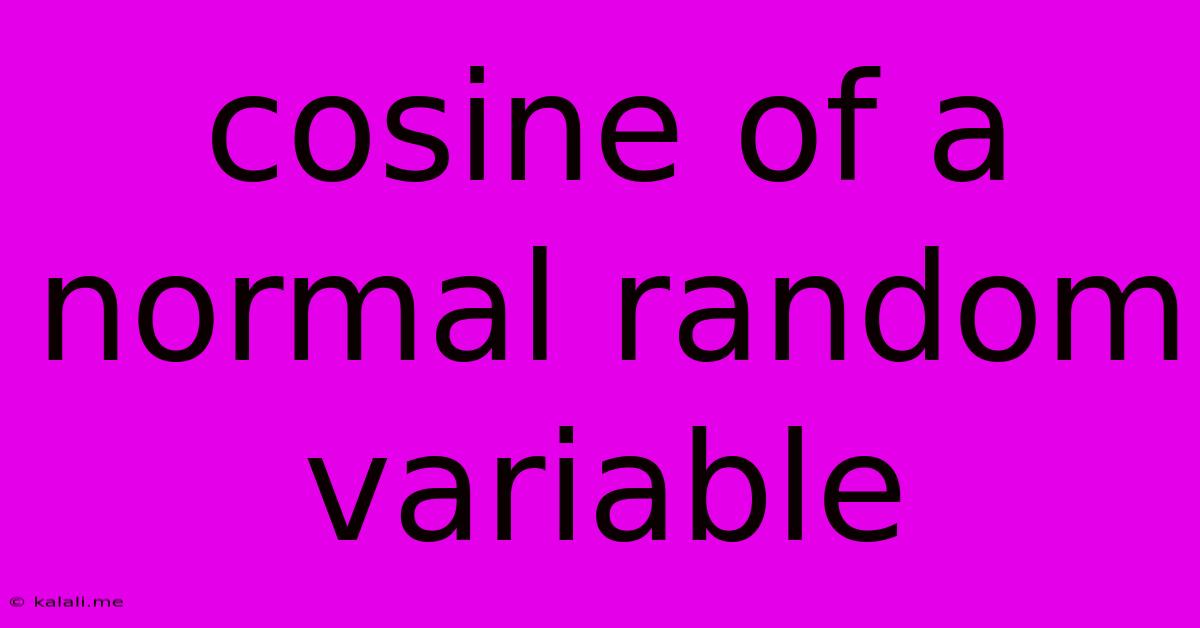
Table of Contents
The Cosine of a Normal Random Variable: Exploring its Distribution and Applications
The cosine of a normally distributed random variable is a fascinating topic that blends probability theory with trigonometric functions. Understanding its distribution and properties has implications in various fields, from signal processing to statistical modeling. This article delves into the intricacies of this mathematical concept, exploring its characteristics and potential applications. While a closed-form solution for the probability density function (PDF) isn't readily available, we can explore its properties and approximations.
What Makes This Problem Interesting?
The distribution of the cosine of a normal random variable isn't straightforward. Unlike simpler transformations of normal variables, applying the cosine function significantly alters the distribution's shape and properties. This complexity arises because the cosine function is periodic and non-linear, making direct analytical solutions challenging. Consequently, we often resort to numerical methods or approximations to understand its behavior.
Understanding the Challenges
The primary challenge lies in deriving the exact probability density function. Standard transformation techniques, which work well for linear transformations, fail when dealing with non-linear functions like cosine. The periodicity of the cosine function further complicates matters, leading to a distribution that's not easily characterized.
Approximation Methods
Several approaches can provide insights into the distribution:
-
Numerical Integration: We can numerically integrate to approximate the cumulative distribution function (CDF) and subsequently obtain the PDF. This method offers accurate results but can be computationally intensive, especially for complex scenarios.
-
Series Expansion: Representing the cosine function using a Taylor series expansion around a specific point might offer an approximate solution. The accuracy of this method depends on the range of values considered and the number of terms included in the expansion.
-
Monte Carlo Simulation: Generating a large number of random samples from a normal distribution, applying the cosine function to each sample, and then analyzing the resulting histogram provides a visual representation of the distribution. This method offers a good approximation, particularly for complex scenarios where analytical methods are impractical. This is a powerful technique for exploring the distribution's characteristics.
-
Approximations based on the Central Limit Theorem: For certain scenarios, considering the Central Limit Theorem might give an approximate understanding. Specifically, if we consider the cosine of the sum of many independent normal variables, its distribution could be approximated using other well-known distributions under specific conditions. This requires specific conditions and may not always be applicable.
Applications and Relevance
Despite the analytical challenges, the cosine of a normal random variable finds applications in:
-
Signal Processing: In signal processing, models often involve the superposition of sinusoidal signals with normally distributed phases. Understanding the distribution of the cosine of a normal variable is crucial for analyzing the resulting signal's statistical properties.
-
Circular Statistics: The cosine transformation is relevant in circular statistics, dealing with data on a circle. This area of statistics finds applications in various fields, including directional data analysis and time-series analysis of periodic phenomena.
-
Stochastic Modeling: Models involving stochastic processes might incorporate the cosine of a normal random variable, particularly in scenarios where periodic behavior is coupled with random fluctuations.
Conclusion
The distribution of the cosine of a normal random variable lacks a readily available closed-form solution. However, employing numerical methods, series expansions, or Monte Carlo simulations helps provide insights into its properties and behavior. Its relevance stretches across diverse fields, highlighting the importance of understanding this mathematically challenging yet practically significant concept. Further research into advanced approximation techniques and specific application scenarios continues to enhance our understanding of this intriguing mathematical entity.
Latest Posts
Latest Posts
-
Does 3 Of A Kind Beat 2 Pair
May 26, 2025
-
Flowchart For Basic Solar Backup System With Electric Co Power
May 26, 2025
-
The Best Is The Enemy Of The Good
May 26, 2025
-
How To Remove Wax From Clothing
May 26, 2025
-
Vscode Terminal Send Sequence Delete All Left Cursor Sendsequence
May 26, 2025
Related Post
Thank you for visiting our website which covers about Cosine Of A Normal Random Variable . We hope the information provided has been useful to you. Feel free to contact us if you have any questions or need further assistance. See you next time and don't miss to bookmark.