Expected Value For Negative Binomial Distribution
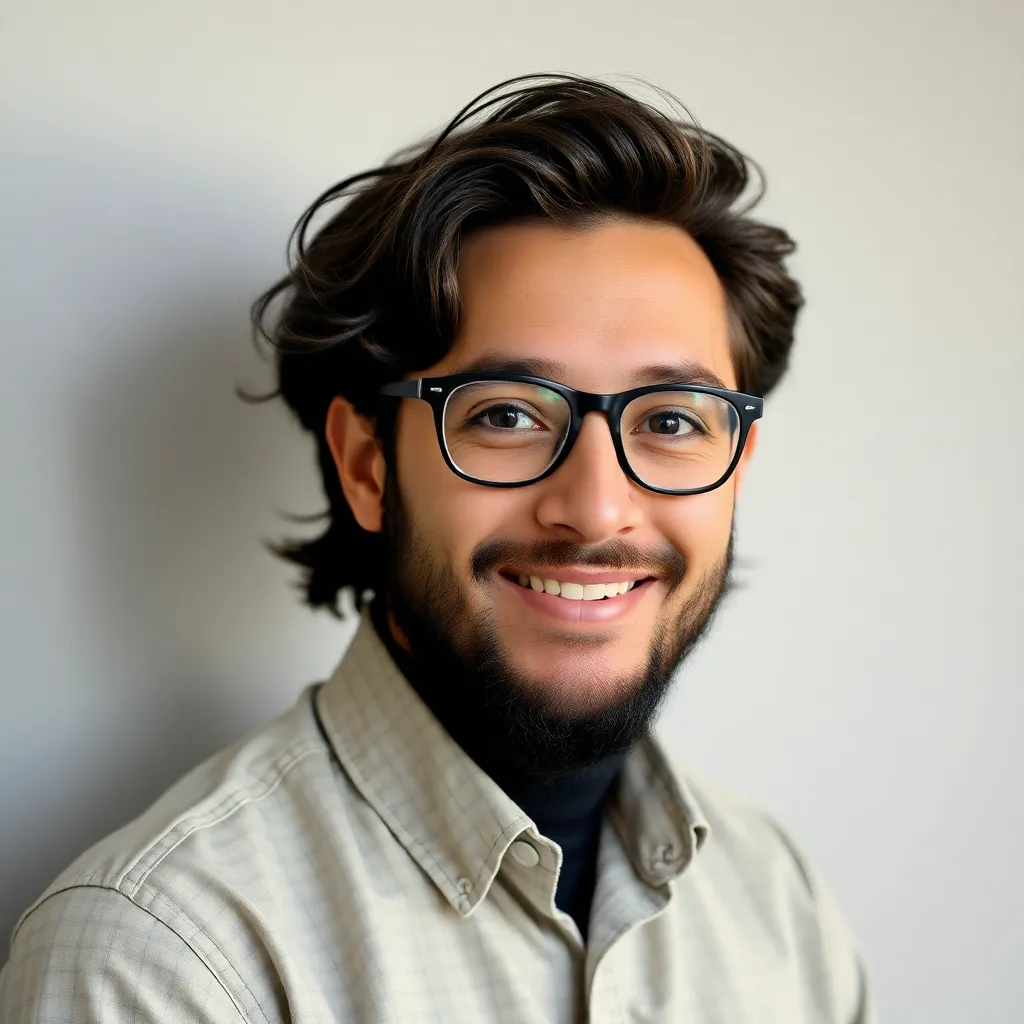
Kalali
May 25, 2025 · 3 min read
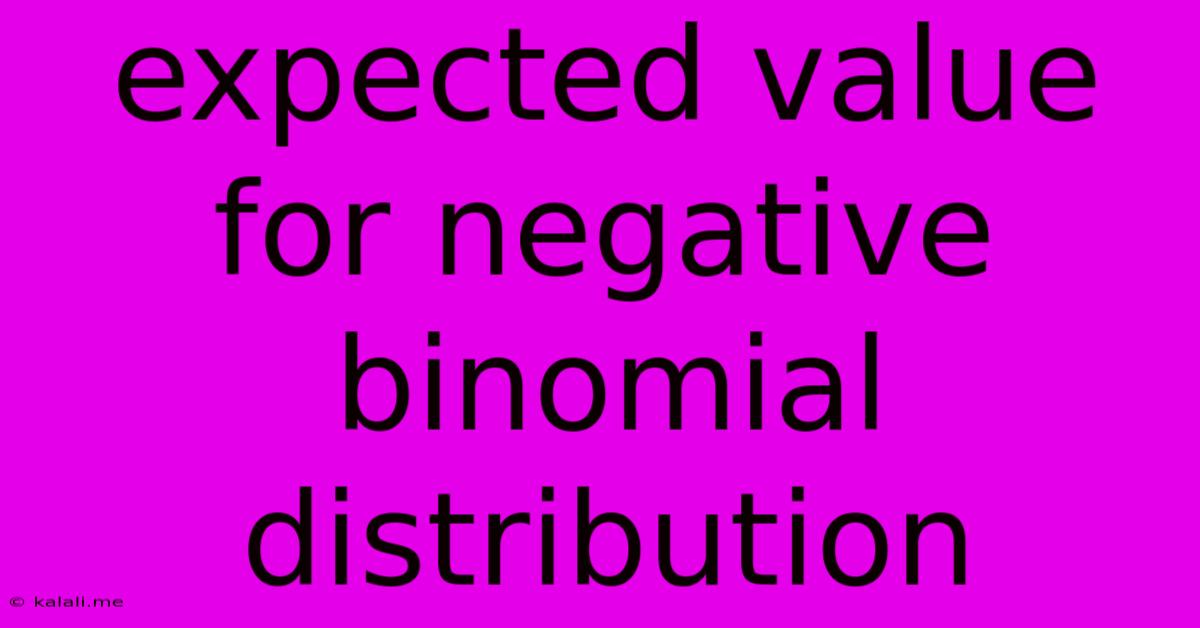
Table of Contents
Understanding Expected Value in the Negative Binomial Distribution
The negative binomial distribution is a powerful tool for modeling the number of failures before a specified number of successes occurs in a sequence of independent Bernoulli trials. Understanding its expected value is crucial for various applications in statistics, from analyzing customer churn to predicting the number of defects in a manufacturing process. This article will delve into the concept of expected value (also known as the mean) for the negative binomial distribution, explaining its formula, derivation, and practical implications.
What is the Negative Binomial Distribution?
Before diving into the expected value, let's briefly recap the negative binomial distribution. It describes the probability of observing r failures before the k-th success in a sequence of independent Bernoulli trials, each with a probability of success p. The distribution is defined by two parameters:
- k: The number of successes (a positive integer).
- p: The probability of success in each trial (0 < p < 1).
The probability mass function (PMF) of the negative binomial distribution is given by:
P(X = x) = (x + k - 1 choose x) * p^k * (1-p)^x where x = 0, 1, 2, ...
Calculating the Expected Value
The expected value, E(X), represents the average number of failures before the k-th success. For the negative binomial distribution, the formula for the expected value is surprisingly simple:
E(X) = k(1-p) / p
Derivation of the Expected Value Formula
While a full mathematical derivation requires some advanced probability concepts, we can intuitively understand this formula. The term (1-p)/p represents the expected number of failures before a single success. Since we're looking for k successes, we multiply this by k to get the expected number of failures before all k successes.
Interpreting the Expected Value
The expected value provides a valuable measure of the central tendency of the negative binomial distribution. For example:
- Higher k leads to a higher expected number of failures. This is intuitive, as more successes require more trials, and hence more potential failures.
- Higher p leads to a lower expected number of failures. A higher probability of success means fewer trials are expected before achieving the k successes.
Practical Applications
The expected value of the negative binomial distribution finds applications in diverse fields, including:
- Quality Control: Predicting the number of defective items before finding a certain number of non-defective ones.
- Customer Churn: Modeling the number of customers lost before acquiring a specific number of new ones.
- Clinical Trials: Estimating the number of unsuccessful treatments before achieving a desired number of successful outcomes.
- Ecology: Determining the number of unsuccessful attempts to find a specific species before encountering a given quantity.
Conclusion
The expected value of the negative binomial distribution provides a concise and meaningful summary of the distribution's central tendency. Understanding its formula and its interpretation allows for informed decision-making in a variety of scenarios where the number of failures before a certain number of successes is a crucial factor. By utilizing this parameter effectively, we can gain valuable insights into various probabilistic phenomena.
Latest Posts
Latest Posts
-
Wiring Diagram For A Four Way Switch
May 25, 2025
-
How Many Cups In One Box Powdered Sugar
May 25, 2025
-
How To Say Common Cold In Spanish
May 25, 2025
-
Look Forward To Talk With You
May 25, 2025
-
Wire Size For 100 Amp Sub Panel
May 25, 2025
Related Post
Thank you for visiting our website which covers about Expected Value For Negative Binomial Distribution . We hope the information provided has been useful to you. Feel free to contact us if you have any questions or need further assistance. See you next time and don't miss to bookmark.