How Does The Outlier Affect The Mean
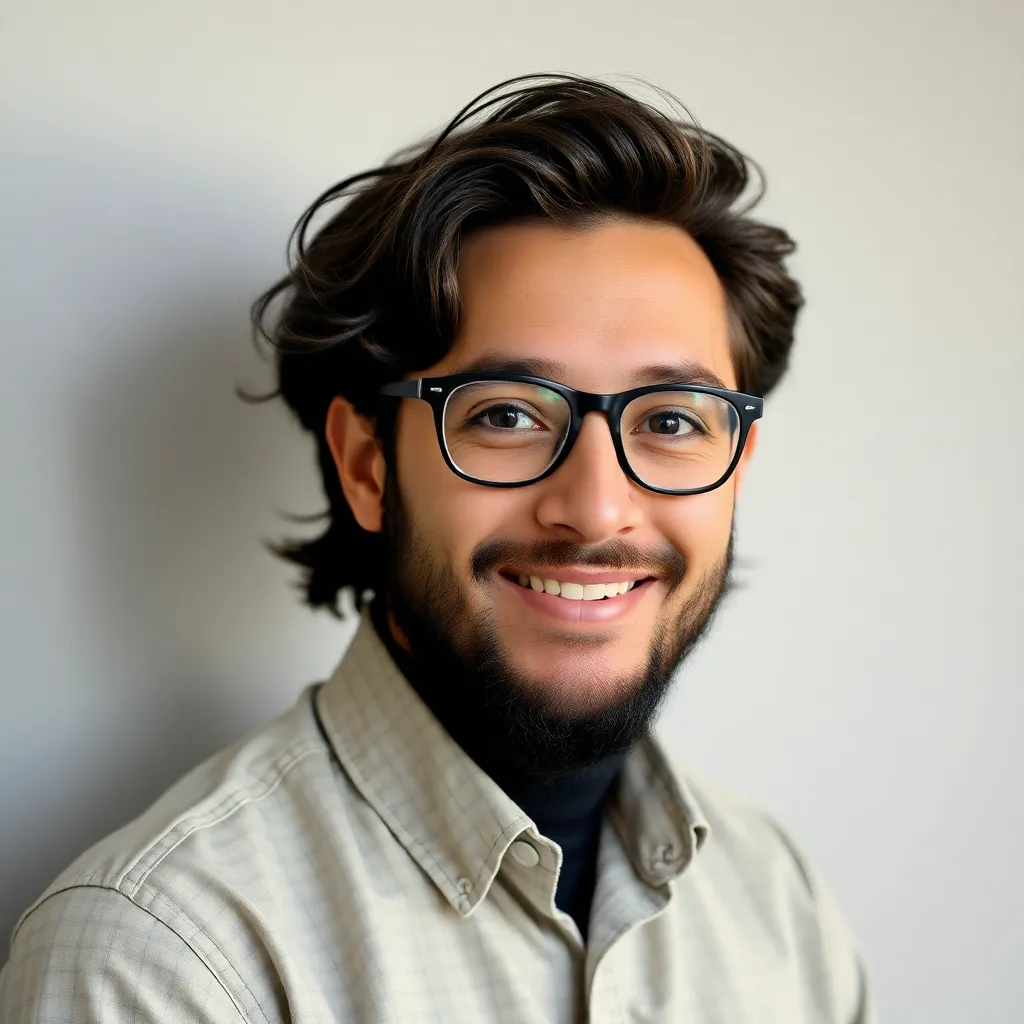
Kalali
Mar 31, 2025 · 6 min read
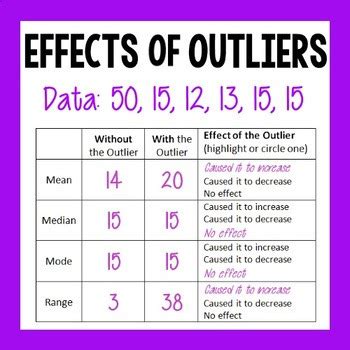
Table of Contents
How Does the Outlier Affect the Mean? Understanding and Addressing Extreme Values in Data Analysis
Data analysis is the bedrock of informed decision-making across numerous fields, from scientific research and financial modeling to social sciences and market research. A crucial aspect of this analysis involves understanding the different measures of central tendency, primarily the mean, median, and mode. While all three offer insights into the central value of a dataset, the mean, or average, is particularly susceptible to the influence of outliers. This article delves deep into how outliers impact the mean, the implications of this impact, and strategies to mitigate its effects.
What is an Outlier?
Before examining the interaction between outliers and the mean, it's crucial to define what constitutes an outlier. Simply put, an outlier is a data point that significantly deviates from other observations in a dataset. This deviation can be due to various reasons, including:
- Data entry errors: Simple mistakes in recording data can lead to extreme values.
- Measurement errors: Faulty instruments or inaccurate measurement techniques might generate outliers.
- Sampling errors: A non-representative sample can include extreme values that don't reflect the population accurately.
- Natural variation: In some cases, outliers genuinely represent extreme values within the population being studied. However, it's important to carefully assess whether this is the case.
Identifying outliers requires careful consideration. There isn't a single universally accepted definition, and the best approach depends on the context and characteristics of the data. Common methods include visual inspection using box plots, scatter plots, and histograms. Statistical methods like the Z-score and Interquartile Range (IQR) are also frequently employed to quantify the extent of deviation and flag potential outliers.
The Mean: A Susceptible Measure
The mean is calculated by summing all values in a dataset and dividing by the number of observations. Its simplicity and intuitive nature make it a widely used measure of central tendency. However, this very simplicity is its Achilles' heel when dealing with outliers.
Because the mean is calculated by summing all values, a single outlier – especially an extreme one – can disproportionately inflate or deflate the average. This can significantly distort the representation of the typical value within the dataset and mislead interpretations. Consider this example:
A small company has 5 employees earning the following annual salaries: $40,000, $45,000, $50,000, $55,000, and $500,000. The mean salary is $138,000. However, this figure is heavily skewed by the extraordinarily high salary of one employee. The mean does not accurately reflect the typical salary within the company. In contrast, the median salary ($50,000) provides a much more representative picture.
The Impact of Outliers on the Mean: A Deeper Dive
The impact of an outlier on the mean is directly proportional to its magnitude and the size of the dataset. A single extreme value in a small dataset will have a far more significant effect than in a large dataset. This is because the extreme value constitutes a larger percentage of the total sum in smaller datasets.
Furthermore, the direction of the impact (increasing or decreasing the mean) depends on whether the outlier is exceptionally high or low. A high outlier will pull the mean upwards, while a low outlier will pull it downwards.
Consequences of Misleading Means:
The distortion of the mean by outliers can lead to several serious consequences in data analysis and interpretation:
- Inaccurate conclusions: Decisions based on a skewed mean can be flawed and lead to wrong inferences.
- Misinterpretation of trends: Outliers can obscure actual trends or patterns in the data.
- Ineffective policy decisions: In areas like public policy or resource allocation, incorrect means can result in ineffective strategies.
- Erroneous statistical tests: Many statistical tests rely on the assumption of normally distributed data. Outliers can violate this assumption and lead to inaccurate test results.
Mitigation Strategies: Dealing with Outliers
Recognizing the influence of outliers on the mean is crucial, but equally important is knowing how to deal with them effectively. The best approach often depends on the context, the reason for the outlier, and the goals of the analysis.
1. Identify and Investigate:
Before taking any action, meticulously investigate the cause of the outlier. Is it a genuine data point reflecting natural variation, or is it due to errors?
2. Data Cleaning and Correction:
If the outlier is due to a clear error (e.g., data entry mistake), the best solution is to correct it or remove it from the dataset. However, this should be done cautiously, with thorough documentation of the changes made.
3. Transformation:
Logarithmic or other transformations can sometimes reduce the influence of outliers by compressing the range of values. This is particularly useful when dealing with skewed data.
4. Robust Alternatives to the Mean:
When outliers are unavoidable or correcting them isn't feasible, robust alternatives to the mean can be more appropriate. The median, the middle value in a sorted dataset, is largely unaffected by outliers. The trimmed mean, which discards a specified percentage of the highest and lowest values, is another robust alternative. Winsorizing, a similar technique, replaces extreme values with less extreme ones, but closer to the data’s core.
5. Non-parametric methods:
Statistical tests that don't rely on assumptions about the data distribution, such as the Mann-Whitney U test or the Kruskal-Wallis test, can be used instead of parametric tests that are sensitive to outliers.
6. Reporting and Transparency:
Even if outliers are removed or mitigated, it’s crucial to transparently report this in the analysis. Document the methods used and explain the rationale behind decisions concerning outlier treatment. This ensures the reproducibility and integrity of the analysis.
Case Studies: Real-World Examples
The impact of outliers on the mean manifests in various real-world scenarios:
-
Financial Markets: Stock prices can experience extreme fluctuations, creating outliers that distort the average returns of a portfolio. Robust measures like the median return provide a more reliable picture of investment performance.
-
Healthcare: A patient with an exceptionally high or low medical cost can skew the average cost of healthcare services within a population. Analyzing costs with the median or considering cost distributions offers a more representative understanding.
-
Environmental Science: Extreme weather events can produce outlier data points when measuring temperature, rainfall, or pollution levels. The median or other robust measures can give a better understanding of general trends, excluding extreme anomalies.
-
Social Sciences: Income inequality frequently generates outliers in income data, making the mean a misleading indicator of average income. The median income often provides a more accurate representation of the typical income level.
Conclusion: The Importance of Context and Critical Thinking
The mean, while a simple and widely used measure, is vulnerable to the distortion caused by outliers. Understanding how outliers affect the mean is paramount for accurate data analysis and informed decision-making. By employing appropriate methods for outlier detection, mitigation, and employing robust alternatives, analysts can ensure a more accurate and reliable representation of their data and draw meaningful conclusions. The key takeaway is the critical importance of context and critical thinking when interpreting statistical measures, particularly the mean, and being aware of the potential biases introduced by extreme values. Remember that the choice of the best method for handling outliers depends heavily on the specific context and goals of the analysis, requiring careful consideration and justification in every case.
Latest Posts
Latest Posts
-
30 To The Power Of 2
Apr 02, 2025
-
What Is 400 Fahrenheit In Centigrade
Apr 02, 2025
-
50 Oz Is How Many Liters
Apr 02, 2025
-
How Many Feet Is 109 Inches
Apr 02, 2025
-
What Is In Both Eukaryotic And Prokaryotic Cells
Apr 02, 2025
Related Post
Thank you for visiting our website which covers about How Does The Outlier Affect The Mean . We hope the information provided has been useful to you. Feel free to contact us if you have any questions or need further assistance. See you next time and don't miss to bookmark.