How To Find Lower Class Limits
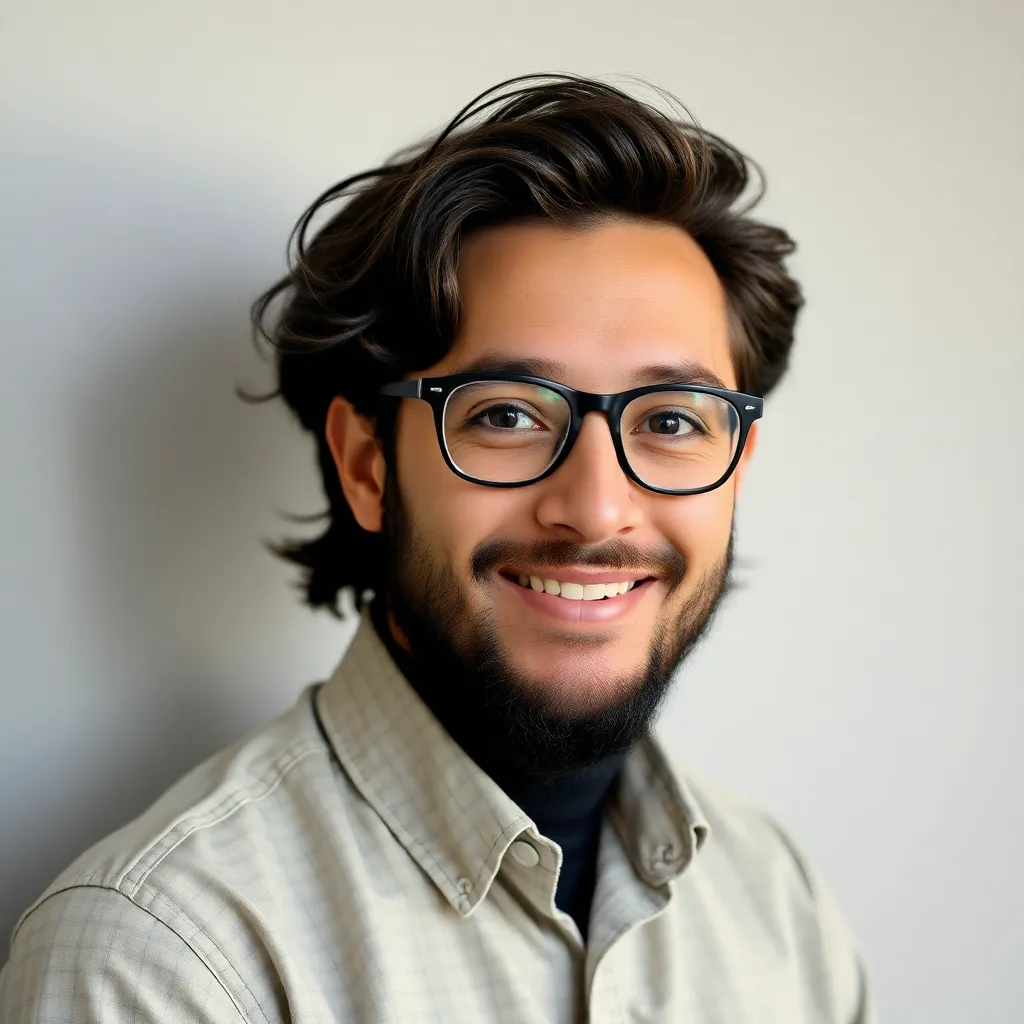
Kalali
Apr 14, 2025 · 6 min read
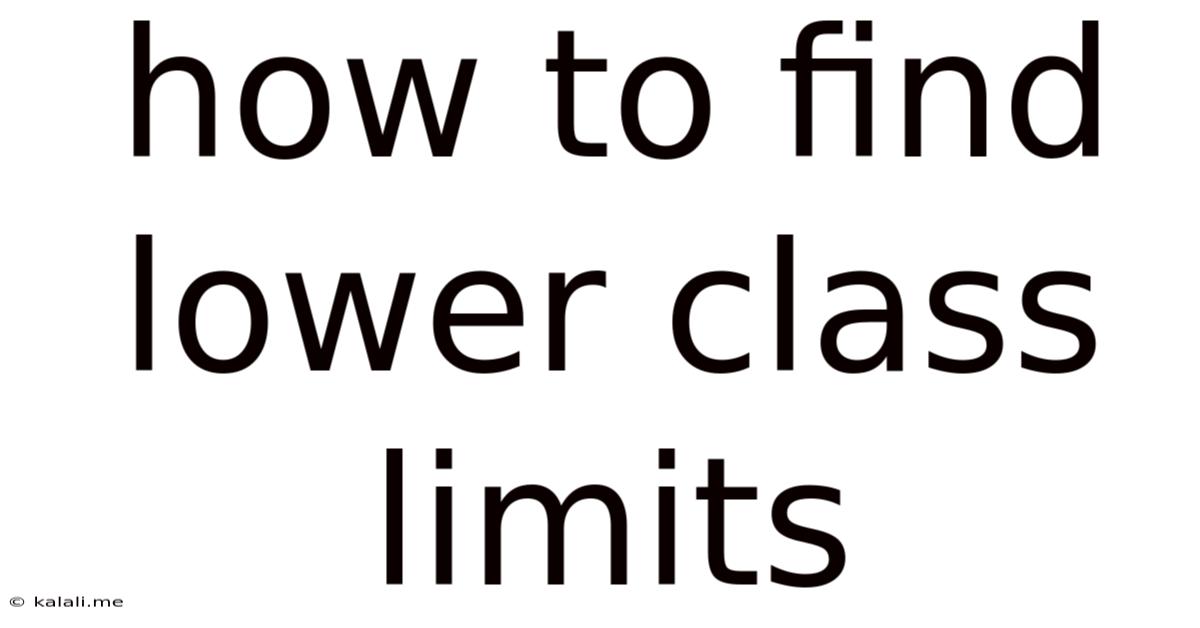
Table of Contents
How to Find Lower Class Limits: A Comprehensive Guide for Data Analysis
Finding lower class limits is a crucial step in data analysis, particularly when working with grouped frequency distributions. Understanding how to determine these limits accurately is essential for creating clear, informative visualizations and conducting meaningful statistical analyses. This comprehensive guide will walk you through various methods and scenarios, ensuring you master this fundamental concept. This article will cover the basics of frequency distributions, explain the importance of lower class limits, and provide step-by-step instructions with numerous examples to solidify your understanding. We'll also explore potential challenges and best practices to ensure accuracy and consistency in your data analysis.
What are Frequency Distributions and Why are Lower Class Limits Important?
Before diving into the mechanics of finding lower class limits, let's establish a firm understanding of frequency distributions. A frequency distribution is a table that summarizes the distribution of a dataset by showing the number of observations (frequency) that fall within specific intervals or classes. These intervals are defined by their upper and lower limits. The lower class limit represents the smallest value that can belong to a particular class, while the upper class limit represents the largest.
Understanding lower class limits is crucial because they form the foundation of your grouped data representation. Inaccurate limits can lead to misinterpretations of the data, skewed visualizations, and erroneous statistical conclusions. They are essential for:
- Accurate Data Grouping: Properly defined limits ensure that each data point is assigned to only one class, preventing ambiguity and data loss.
- Creating Histograms and Frequency Polygons: Lower class limits are used to define the boundaries of bars in histograms and points in frequency polygons, providing a visual representation of the data distribution.
- Calculating Descriptive Statistics: Many statistical calculations, such as mean, median, and standard deviation, are often performed on grouped data, and the accuracy of these calculations depends heavily on the accuracy of the class limits.
- Data Interpretation: Clearly defined class limits facilitate the accurate interpretation and communication of data findings.
Methods for Determining Lower Class Limits
The method used to find lower class limits depends on the nature of your data and the desired level of detail in your frequency distribution. Here are the most common approaches:
1. Direct Observation from Raw Data:
This is the simplest method, applicable when you have a small, ungrouped dataset. By visually inspecting the data, you can identify the smallest value. This smallest value becomes the lower class limit of the first class.
Example:
Let's say you have the following ungrouped data representing the ages of participants in a study: 22, 25, 28, 30, 31, 33, 35, 38, 40, 42.
The smallest value is 22. Therefore, the lower class limit of the first class is 22.
2. Determining Class Width and Number of Classes:
This method is more common when dealing with larger datasets. It involves:
- Determining the range: Find the difference between the highest and lowest values in your data.
- Choosing the number of classes: The number of classes depends on the size of your data and the desired level of detail. Too few classes may obscure important patterns, while too many classes may make the data too granular. A common guideline is to use between 5 and 20 classes. The optimal number often depends on the specific dataset and its characteristics.
- Calculating the class width: Divide the range by the desired number of classes. Round the result up to a convenient value (e.g., a whole number or a multiple of 5 or 10). This ensures that all data points fall within a class.
- Defining the lower class limit: The lower class limit of the first class is usually set as the minimum value in your dataset or a slightly lower, convenient value to encompass all data points. Subsequent lower class limits are found by adding the class width to the previous class's lower class limit.
Example:
Let's say you have data on the weights (in kilograms) of 100 students, with a minimum weight of 45 kg and a maximum weight of 92 kg.
- Range: 92 kg - 45 kg = 47 kg
- Number of classes: Let's choose 7 classes.
- Class width: 47 kg / 7 ≈ 6.7 kg. We round this up to 7 kg.
- Lower class limits: We'll start with a lower class limit of 45 kg for the first class. The subsequent lower class limits would be: 45 kg, 52 kg (45 + 7), 59 kg (52 + 7), 66 kg (59 + 7), 73 kg (66 + 7), 80 kg (73 + 7), 87 kg (80 + 7).
3. Using Existing Class Intervals:
Sometimes, you may be working with data that already has predefined class intervals. In such cases, the lower class limits are simply the lower boundaries of these intervals.
Example:
Imagine you're analyzing data presented in a table with class intervals like 10-19, 20-29, 30-39. The lower class limits would be 10, 20, and 30, respectively.
4. Dealing with Decimal Data:
When working with decimal data, you need to be mindful of the level of precision you want to maintain. You might need to round your class width and lower class limits to a specific number of decimal places to maintain consistency.
Potential Challenges and Best Practices
Several challenges can arise when determining lower class limits:
- Outliers: Extreme values (outliers) can significantly influence the range and, consequently, the class width and lower class limits. Consider whether to exclude outliers or adjust your class intervals to accommodate them.
- Unequal Class Intervals: While generally avoided, unequal class intervals might be necessary in certain situations, such as when dealing with skewed data or when specific ranges need emphasis. However, this requires careful consideration and clear labeling.
- Data Transformation: In some cases, data transformation (e.g., logarithmic transformation) might be necessary to improve the distribution and make it easier to define class intervals.
- Choice of Number of Classes: Selecting the optimal number of classes is subjective. Experiment with different numbers of classes to find the representation that best reflects the underlying data distribution.
Best Practices:
- Clearly define your methodology: Document the method used to determine class limits for transparency and reproducibility.
- Use convenient values: Choose class widths and lower class limits that are easy to understand and interpret.
- Avoid overlapping classes: Ensure that each data point belongs to only one class.
- Consider the context: The choice of class intervals should be informed by the nature of the data and the research question.
Conclusion:
Finding lower class limits is a fundamental yet crucial step in data analysis. By mastering the techniques discussed in this guide, you can ensure the accuracy and clarity of your grouped frequency distributions, leading to more reliable statistical analyses and more effective communication of your findings. Remember to consider the specific characteristics of your data and choose the method best suited to the situation, always prioritizing clear and transparent data representation. Thorough understanding and careful application of these methods will ensure robust and reliable results in your data analysis endeavors.
Latest Posts
Latest Posts
-
What Is The Fraction For 1 6
Apr 15, 2025
-
What Is 20 5 Inches In Cm
Apr 15, 2025
-
How Many Gallons Are In 7 L
Apr 15, 2025
-
How Many Lines Of Symmetry Has A Rhombus
Apr 15, 2025
-
What Is 1 3 Of A Pound
Apr 15, 2025
Related Post
Thank you for visiting our website which covers about How To Find Lower Class Limits . We hope the information provided has been useful to you. Feel free to contact us if you have any questions or need further assistance. See you next time and don't miss to bookmark.