Joint Of Two Continuous Rvs Not Continuous
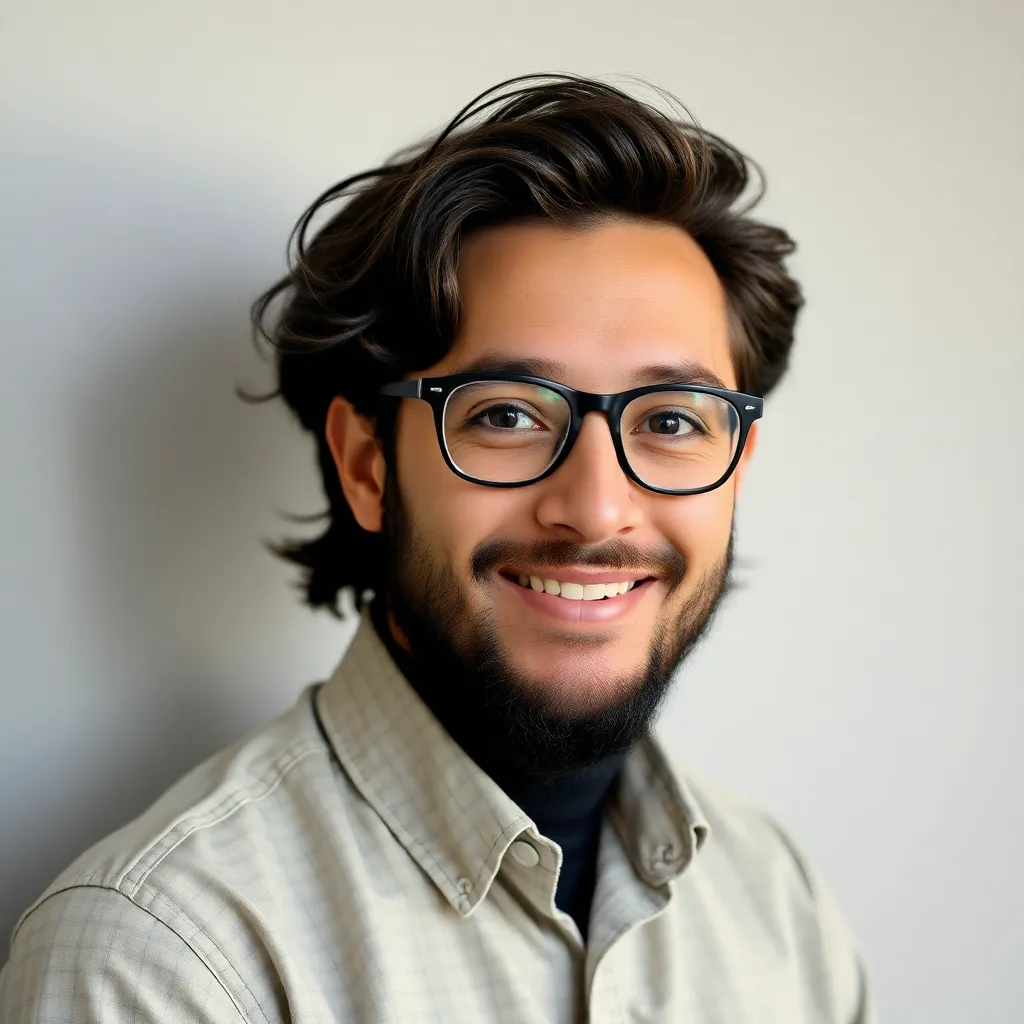
Kalali
May 23, 2025 · 3 min read
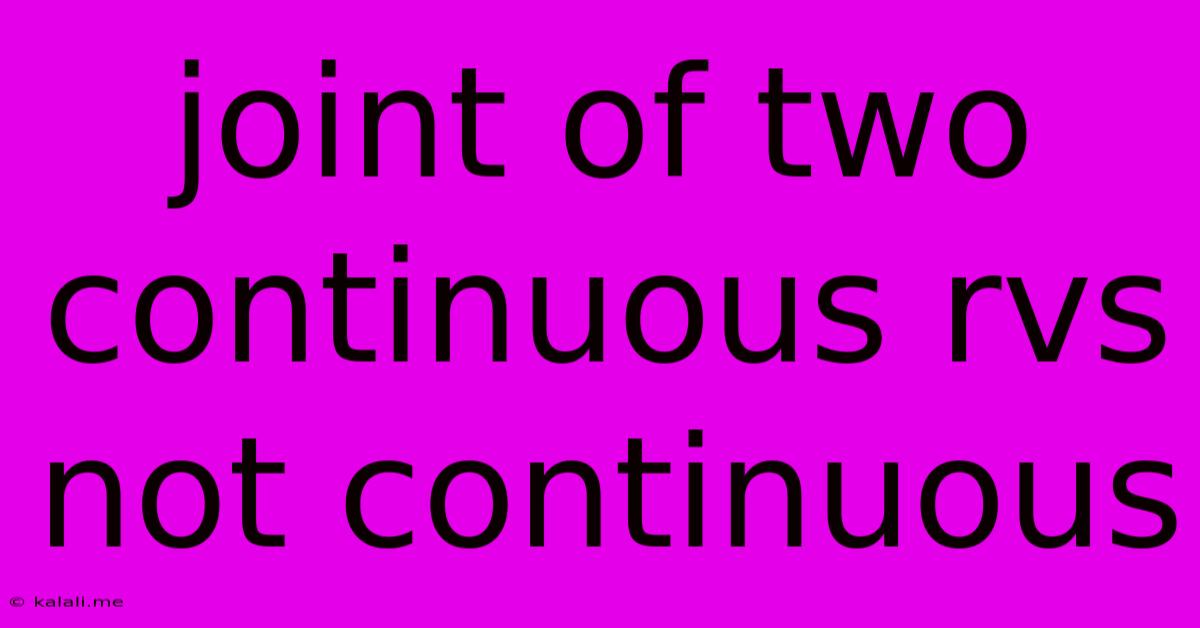
Table of Contents
When the Sum of Continuous Random Variables Isn't Continuous: A Deep Dive into Convolution and its Exceptions
This article explores the seemingly paradoxical situation where the sum of two continuous random variables fails to be continuous. While the convolution theorem generally dictates that the sum of independent continuous random variables is itself continuous, specific scenarios can produce exceptions. Understanding these exceptions requires delving into the intricacies of probability density functions (PDFs) and the limitations of the convolution process. This is crucial for accurate modeling in various fields like signal processing and statistical mechanics.
Understanding the Convolution Theorem
The standard approach to finding the distribution of the sum of two independent continuous random variables, X and Y, with PDFs f<sub>X</sub>(x) and f<sub>Y</sub>(y) respectively, involves convolution:
f<sub>Z</sub>(z) = ∫ f<sub>X</sub>(x)f<sub>Y</sub>(z-x) dx
where Z = X + Y. This integral represents the overlap of the two PDFs, effectively summing the probabilities of all possible combinations of X and Y that result in a specific value of Z. Intuitively, the result is usually a continuous function reflecting a continuous range of possible sums.
Scenarios Leading to Discontinuous Sums
However, this continuous result hinges on certain assumptions. Departures from these assumptions can lead to a discontinuous distribution for Z, even if X and Y are individually continuous. Here are some key exceptions:
1. Non-Independent Random Variables:
The convolution formula relies critically on the independence of X and Y. If X and Y are dependent, their joint distribution cannot be simply expressed as the product of their marginal distributions. Consequently, the sum's distribution may be discontinuous, reflecting the dependencies between the variables. For example, consider X and Y such that Y = -X. Their sum will always be zero, resulting in a highly discontinuous distribution.
2. Singular Distributions:**
While less common, certain continuous random variables can possess PDFs that are not absolutely continuous. These singular distributions concentrate probability mass on sets of measure zero, such as specific points or curves. Adding two random variables with singular distributions can lead to a discontinuous sum distribution. These scenarios often involve pathological functions, and are less frequently encountered in practical applications.
3. Discrete Components within Continuous Distributions:**
A less obvious exception occurs when either X or Y (or both) exhibit a mixture of continuous and discrete components in their distributions. This might arise from a probabilistic model incorporating both continuous and discrete elements. Suppose X is a continuous variable, but Y has a non-zero probability of taking a specific value. This discrete component will directly influence the sum Z, potentially creating discontinuities in the resulting distribution.
Practical Implications and Further Considerations:
The scenarios outlined above highlight the importance of carefully considering the nature of the involved random variables before assuming the sum will be continuous. Misunderstanding these exceptions can lead to inaccurate probabilistic models and erroneous conclusions in various applications. Advanced techniques, such as characteristic functions, can sometimes be used to determine the distribution of the sum even in cases where direct convolution becomes intractable or yields discontinuous results.
In summary, while the convolution theorem provides a powerful tool for analyzing the sum of continuous random variables, it's crucial to acknowledge its limitations. The independence of variables and the nature of their individual distributions play a crucial role in determining whether the resulting sum distribution will be continuous or exhibit discontinuities. Understanding these exceptions is paramount for constructing accurate and reliable probabilistic models.
Latest Posts
Latest Posts
-
Apex Rest Api Handle 1gb Data
May 23, 2025
-
Do Vanity Receptacles Have To Be At Counter Height
May 23, 2025
-
Subcaption Package Latex Set Caption Size
May 23, 2025
-
How To Use Jcp Reference In Beamer Latex
May 23, 2025
-
Is Delta E Zero At Equilibrium
May 23, 2025
Related Post
Thank you for visiting our website which covers about Joint Of Two Continuous Rvs Not Continuous . We hope the information provided has been useful to you. Feel free to contact us if you have any questions or need further assistance. See you next time and don't miss to bookmark.