Moment Generating Function Of A Poisson Distribution
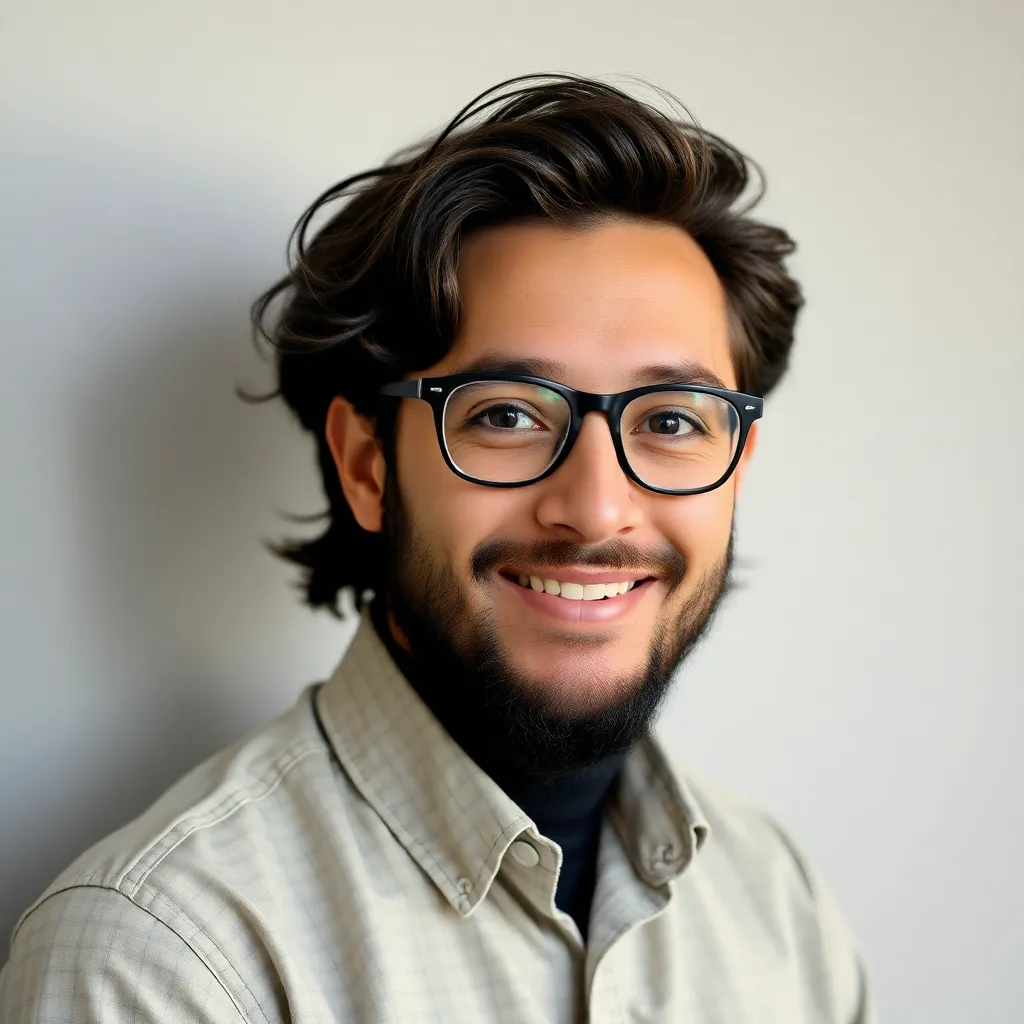
Kalali
May 19, 2025 · 3 min read
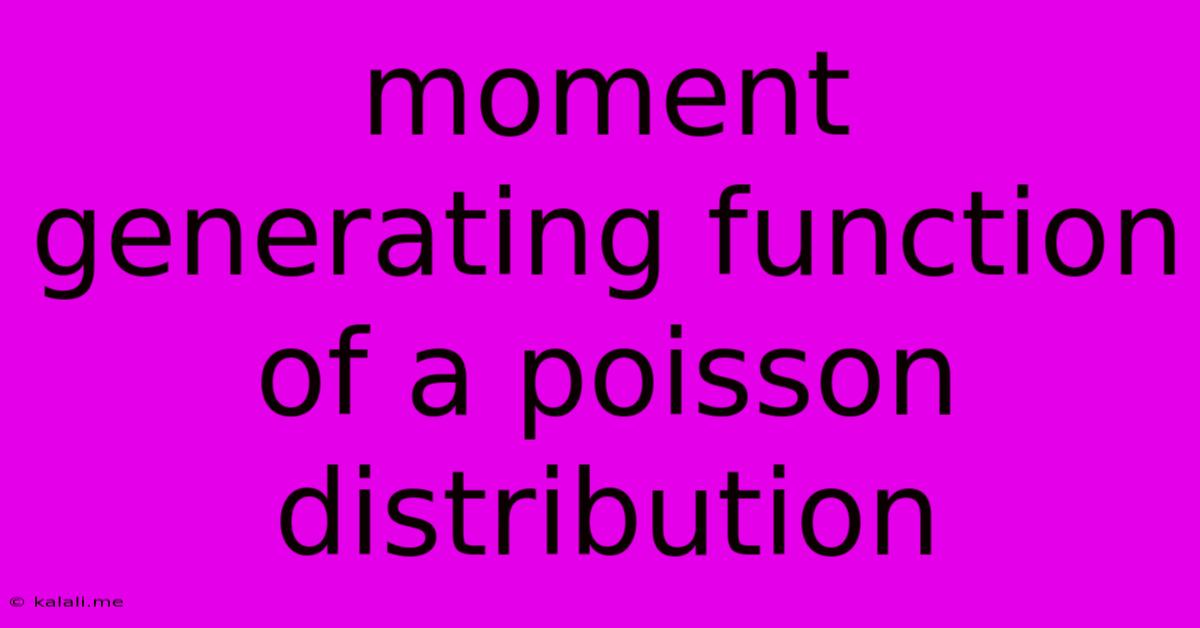
Table of Contents
Understanding the Moment Generating Function of a Poisson Distribution
The Poisson distribution, a staple in probability and statistics, models the probability of a given number of events occurring in a fixed interval of time or space if these events occur with a known average rate and independently of the time since the last event. Understanding its moment generating function (MGF) provides a powerful tool for deriving key characteristics like mean, variance, and higher moments. This article will delve into the derivation and applications of the Poisson distribution's MGF.
What is a Moment Generating Function (MGF)?
The MGF of a random variable X, denoted as M<sub>X</sub>(t), is defined as the expected value of e<sup>tX</sup>:
M<sub>X</sub>(t) = E[e<sup>tX</sup>] = Σ [e<sup>tx</sup> * P(X = x)] (for discrete random variables)
or
M<sub>X</sub>(t) = ∫ e<sup>tx</sup> * f<sub>X</sub>(x) dx (for continuous random variables)
where:
- t is a real number.
- P(X = x) is the probability mass function (PMF) for discrete variables.
- f<sub>X</sub>(x) is the probability density function (PDF) for continuous variables.
The MGF, when it exists, uniquely determines the probability distribution. It's a powerful tool because moments of the distribution can be easily obtained by differentiating the MGF and evaluating at t = 0.
Deriving the MGF of a Poisson Distribution
A Poisson random variable X with parameter λ (lambda representing the average rate of events) has the probability mass function:
P(X = k) = (λ<sup>k</sup> * e<sup>-λ</sup>) / k! for k = 0, 1, 2, ...
Let's derive the MGF:
M<sub>X</sub>(t) = E[e<sup>tX</sup>] = Σ [e<sup>tk</sup> * P(X = k)] = Σ [e<sup>tk</sup> * (λ<sup>k</sup> * e<sup>-λ</sup>) / k!]
= Σ [(e<sup>t</sup>λ)<sup>k</sup> * e<sup>-λ</sup> / k!]
We can factor out e<sup>-λ</sup>:
M<sub>X</sub>(t) = e<sup>-λ</sup> * Σ [(e<sup>t</sup>λ)<sup>k</sup> / k!]
Recall the Taylor series expansion of e<sup>x</sup>:
e<sup>x</sup> = Σ (x<sup>k</sup> / k!)
Substituting x = e<sup>t</sup>λ, we get:
M<sub>X</sub>(t) = e<sup>-λ</sup> * e<sup>(e<sup>t</sup>λ)</sup> = e<sup>λ(e<sup>t</sup> - 1)</sup>
Therefore, the moment generating function of a Poisson distribution with parameter λ is e<sup>λ(e<sup>t</sup> - 1)</sup>.
Applications of the MGF
The MGF simplifies the calculation of moments:
-
Mean (E[X]): The first moment, or mean, is obtained by taking the first derivative of the MGF with respect to t and evaluating at t = 0:
dM<sub>X</sub>(t)/dt |<sub>t=0</sub> = λ
-
Variance (Var[X]): The second moment is obtained by taking the second derivative of the MGF with respect to t and evaluating at t = 0. After some calculations involving the first and second derivatives, we find the variance to be λ.
-
Higher Moments: Higher-order moments (skewness, kurtosis, etc.) can also be derived using higher-order derivatives of the MGF.
In summary, the moment generating function provides an elegant and efficient method for characterizing the Poisson distribution and extracting its key statistical properties. Its use streamlines calculations, making it a valuable tool for statisticians and anyone working with Poisson processes. Understanding the MGF of the Poisson distribution opens doors to a deeper understanding of this widely applicable probability model.
Latest Posts
Latest Posts
-
Shock Absorbers Light Misting Of Oil
May 19, 2025
-
How To Say How Do You Do In French
May 19, 2025
-
Mix Ratio For Sand And Cement
May 19, 2025
-
How Many Calories In A Cup Rice
May 19, 2025
-
What Does Piano Mean In Italian
May 19, 2025
Related Post
Thank you for visiting our website which covers about Moment Generating Function Of A Poisson Distribution . We hope the information provided has been useful to you. Feel free to contact us if you have any questions or need further assistance. See you next time and don't miss to bookmark.