Pincipal Component Analysis Of The Yield Curve
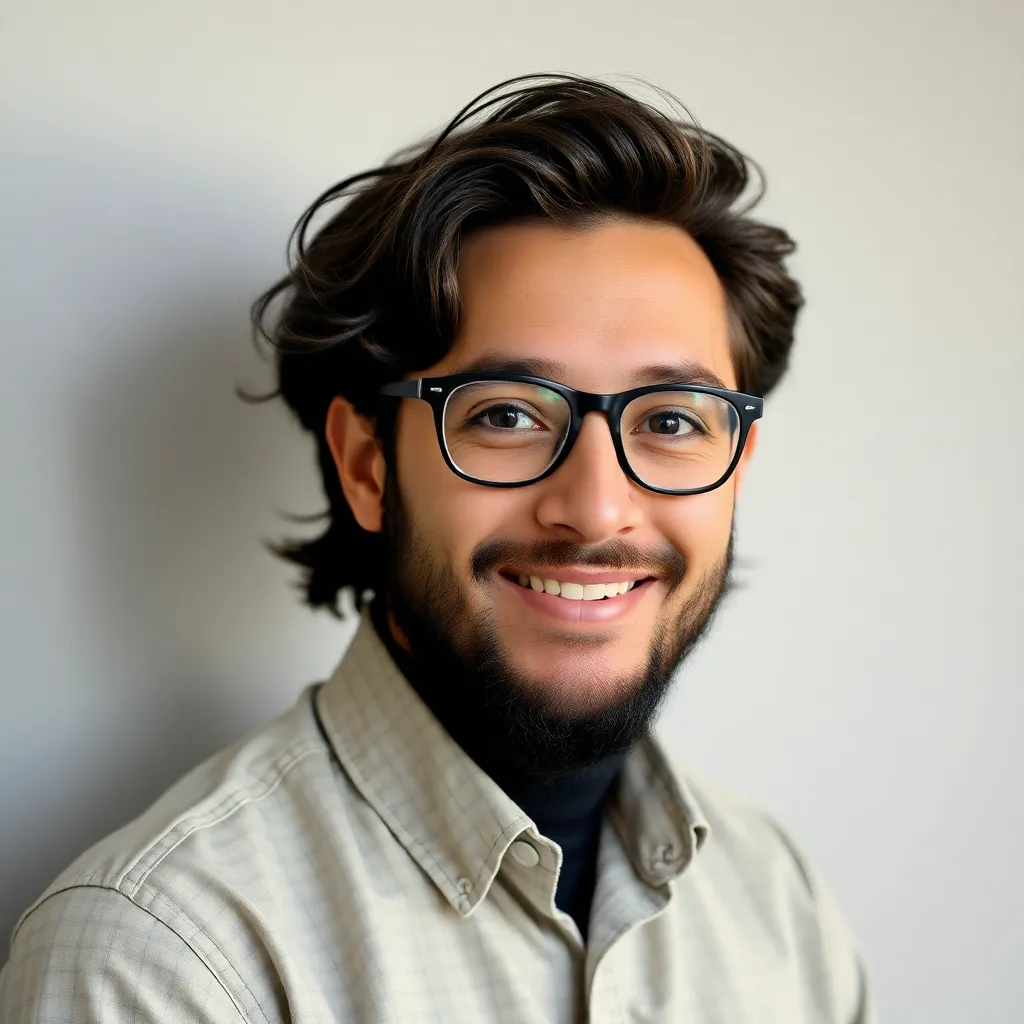
Kalali
May 24, 2025 · 4 min read
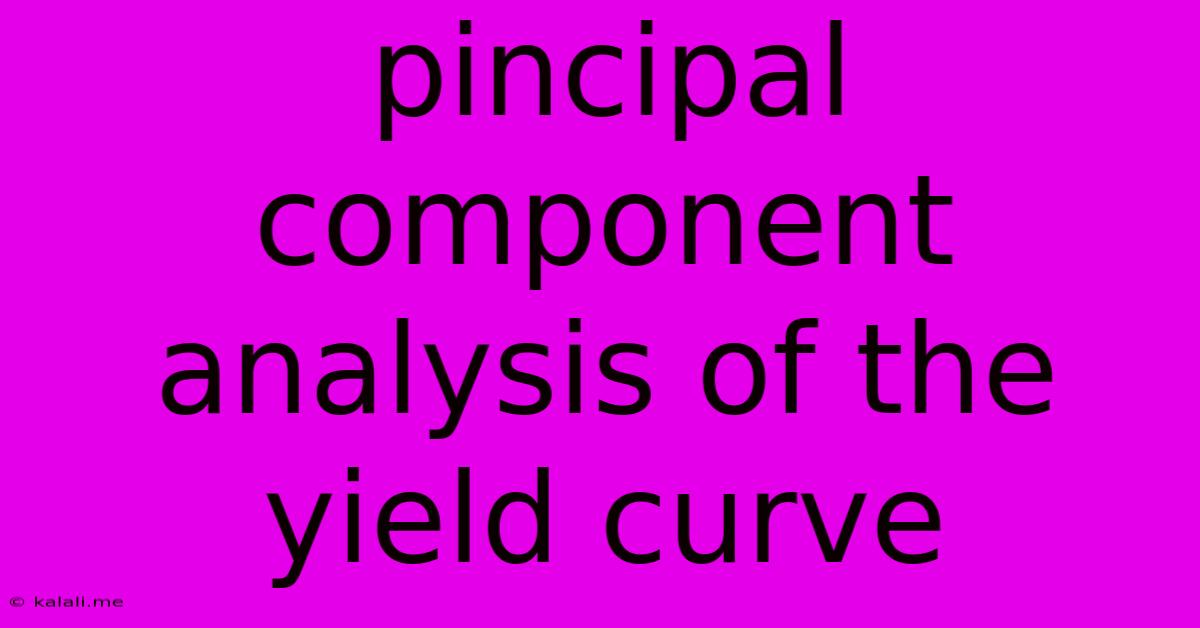
Table of Contents
Principal Component Analysis of the Yield Curve: Uncovering Hidden Factors
The yield curve, a graphical representation of the relationship between interest rates and time to maturity, is a cornerstone of fixed-income analysis. However, its multi-dimensional nature makes direct interpretation challenging. This is where Principal Component Analysis (PCA) comes in, offering a powerful tool to distill the curve's complexity into a smaller set of uncorrelated factors that capture the majority of its variation. This article delves into the application of PCA to yield curve analysis, explaining its mechanics, interpretation, and practical implications.
What is Principal Component Analysis (PCA)?
PCA is a statistical technique used for dimensionality reduction. It transforms a large set of correlated variables into a smaller set of uncorrelated variables called principal components. These components are ordered by the amount of variance they explain, with the first principal component accounting for the largest portion of the total variance. Subsequent components explain progressively smaller portions. By focusing on the most significant components, we can effectively summarize the essential information contained in the original dataset while reducing noise and complexity.
Applying PCA to the Yield Curve
In the context of the yield curve, the input data typically consists of a time series of yields across different maturities (e.g., 3-month, 6-month, 1-year, 2-year, 5-year, 10-year Treasury yields). PCA then identifies the underlying factors driving the changes in these yields. The resulting principal components represent these latent factors, offering a more concise and interpretable view of yield curve dynamics.
Interpreting the Principal Components
Typically, the first three principal components of a yield curve explain a significant portion (often exceeding 90%) of the total variance. These components often have intuitive economic interpretations:
-
Level: The first principal component usually represents the overall level of interest rates. A positive change in this component indicates a general upward shift in the entire yield curve. This component captures parallel shifts in yields across all maturities.
-
Slope: The second principal component often reflects the slope of the yield curve. A positive value suggests a steepening yield curve (longer-term yields rising faster than shorter-term yields), while a negative value indicates a flattening or inverting curve. This component captures changes in the relationship between short-term and long-term interest rates.
-
Curvature: The third principal component typically captures the curvature or convexity of the yield curve. A positive value might indicate a more pronounced humped shape, while a negative value could suggest a flatter or more inverted curve. This component reflects changes in the shape of the yield curve beyond simple slope changes.
Practical Implications and Uses
Understanding the principal components of the yield curve offers several practical advantages:
-
Forecasting: By modeling the dynamics of the principal components, we can improve the accuracy of yield curve forecasts. This is because the components capture the essential information in a more parsimonious way than modeling individual yields.
-
Risk Management: PCA facilitates better risk management by identifying and quantifying the key risk factors driving yield curve movements. Portfolio managers can then use this information to tailor their strategies accordingly.
-
Trading Strategies: Traders can use principal component analysis to construct trading strategies that exploit relationships between these factors. For instance, a strategy might be developed to capitalize on changes in the slope of the curve.
-
Macroeconomic Analysis: The extracted components can provide insights into macroeconomic conditions and expectations. For instance, a persistently steepening yield curve (positive slope component) might signal strong economic growth.
Limitations
It's crucial to acknowledge that PCA has limitations:
-
Interpretation: While the interpretation of the first few components is often intuitive, higher-order components can be more difficult to interpret economically.
-
Data Dependency: The results of PCA are sensitive to the choice of data (e.g., specific maturities used, time period considered).
-
Stationarity: PCA assumes that the underlying data is stationary. If this assumption is violated, the results might be misleading.
Conclusion
Principal Component Analysis provides a powerful framework for analyzing and understanding the complexities of the yield curve. By transforming the high-dimensional yield data into a smaller set of uncorrelated factors, PCA facilitates forecasting, risk management, and the development of sophisticated trading strategies. However, careful consideration of the limitations and assumptions is essential for proper interpretation and application. Combining PCA with other quantitative techniques can enhance its analytical power and provide a comprehensive understanding of yield curve dynamics.
Latest Posts
Latest Posts
-
How Do You Get Gasoline Smell Off Hands
May 24, 2025
-
How To Remove The Rust Stains From Clothes
May 24, 2025
-
How Do You Get Gasoline Smell Off Your Hands
May 24, 2025
-
How To Refresh Chunks In Minecraft
May 24, 2025
-
Moves That Cant Be Used At All
May 24, 2025
Related Post
Thank you for visiting our website which covers about Pincipal Component Analysis Of The Yield Curve . We hope the information provided has been useful to you. Feel free to contact us if you have any questions or need further assistance. See you next time and don't miss to bookmark.