Probability Distribution Of X And X_n
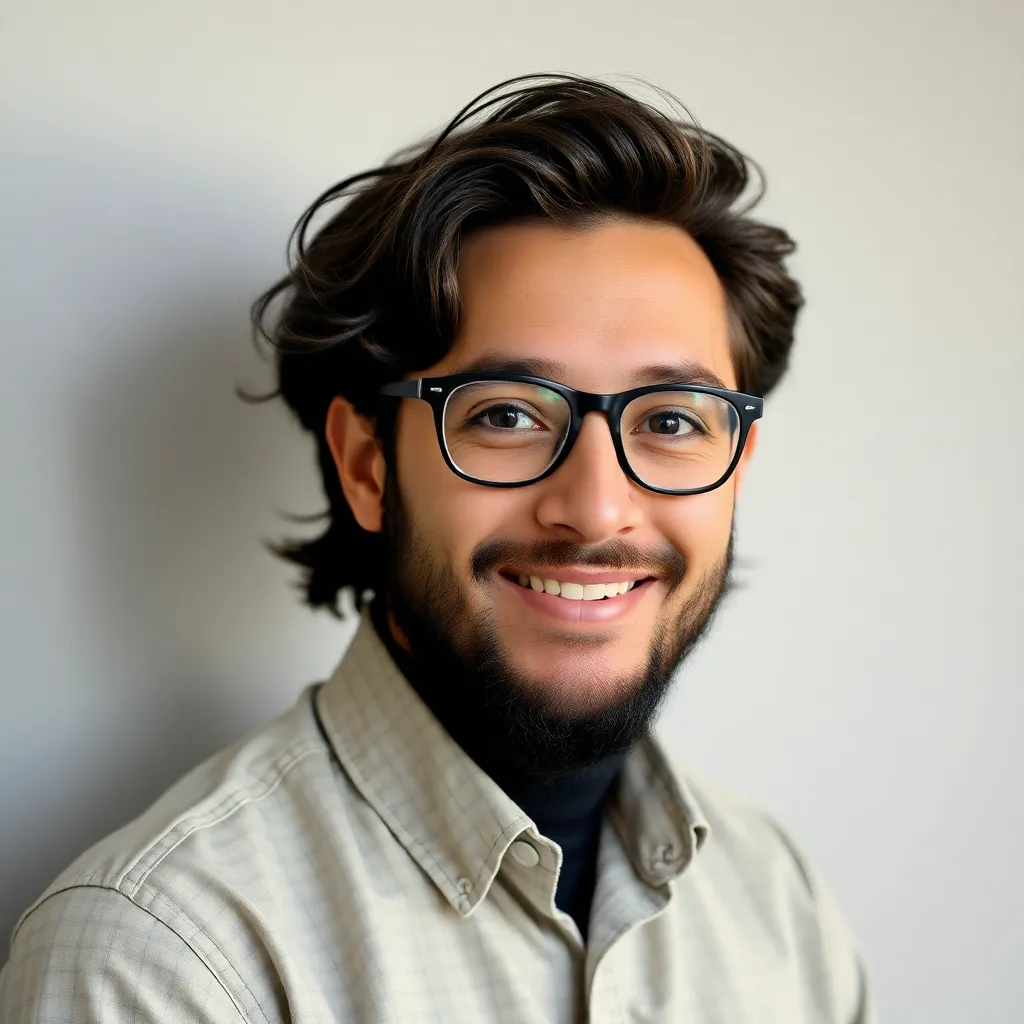
Kalali
May 23, 2025 · 3 min read
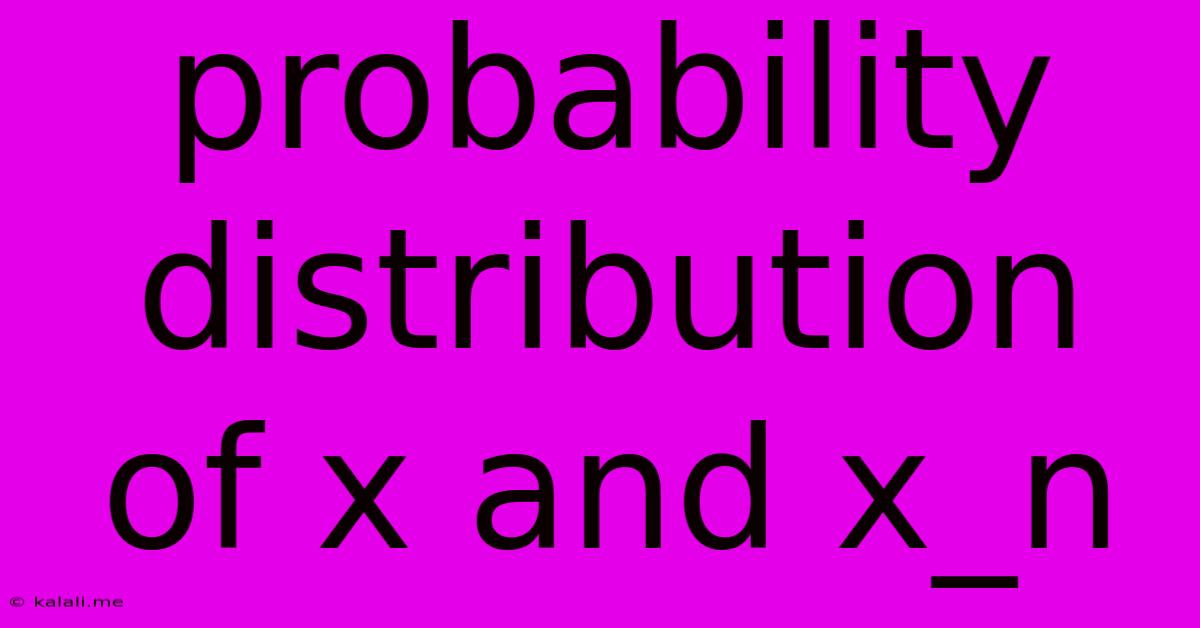
Table of Contents
Understanding Probability Distributions of X and Xₙ: A Deep Dive
Understanding probability distributions is fundamental to statistics and numerous applications across various fields. This article delves into the probability distributions of a random variable X and its sequence of approximations, Xₙ. We'll explore key concepts, differences, and their practical implications. This will cover topics including the law of large numbers and the central limit theorem.
What is a Probability Distribution?
A probability distribution describes the likelihood of obtaining different possible values for a random variable. It's a mathematical function that assigns probabilities to all possible outcomes of a random variable. These distributions can be discrete (for variables taking on distinct values like the number of heads in three coin tosses) or continuous (for variables taking on values within a range, like the height of students in a class). Key characteristics of a probability distribution include its mean (average value), variance (spread or dispersion), and standard deviation (square root of variance).
The Random Variable X:
The random variable X represents the outcome of a single random event. Its probability distribution, denoted as P(X=x) for discrete variables or f(x) for continuous variables, provides the probability of X taking on a specific value x. The type of distribution (e.g., binomial, normal, Poisson, exponential) depends on the nature of the random process generating X.
The Sequence of Random Variables Xₙ:
Xₙ represents a sequence of random variables, often resulting from repeated trials or observations of the same random process. Each Xₙ is a random variable with its own probability distribution. The sequence X₁, X₂, X₃,... aims to approximate or estimate some underlying parameter or characteristic of the process. For instance, Xₙ could be the average of n independent and identically distributed (i.i.d.) random variables.
Relationship Between X and Xₙ:
The relationship between X and Xₙ hinges on the concepts of:
1. The Law of Large Numbers:
This fundamental theorem states that as the number of trials (n) increases, the sample average (Xₙ) converges towards the expected value (mean) of the underlying random variable X. Formally, lim (n→∞) Xₙ = E(X). This means that with enough data, the average of our observations gets increasingly closer to the true population mean.
2. The Central Limit Theorem:
This theorem is crucial for statistical inference. It states that the distribution of the sample average (Xₙ), properly normalized, converges to a standard normal distribution as n approaches infinity, regardless of the underlying distribution of X (provided it has a finite mean and variance). This allows us to use the normal distribution to approximate probabilities related to sample averages, even if the original data isn't normally distributed. This is invaluable for hypothesis testing and confidence interval construction.
Practical Implications:
Understanding the distributions of X and Xₙ is essential in:
- Statistical Inference: Drawing conclusions about a population based on a sample. The central limit theorem justifies the use of normal approximations for hypothesis tests and confidence intervals.
- Risk Management: Assessing and managing risks associated with uncertain events. Probability distributions help quantify the likelihood of different outcomes.
- Machine Learning: Modeling and predicting outcomes using data. Probability distributions are fundamental to many machine learning algorithms.
- Simulation: Modeling complex systems using random variables. Understanding distributions allows for more accurate simulations.
Conclusion:
The probability distributions of X and Xₙ are cornerstones of statistical analysis and numerous applications. The law of large numbers and the central limit theorem provide powerful insights into the behavior of sample averages and enable the use of powerful statistical tools. A thorough understanding of these concepts is crucial for anyone working with data and uncertainty.
Latest Posts
Latest Posts
-
Linux How To Unzip A Zip File
May 23, 2025
-
How Often Should You Change Spark Plugs
May 23, 2025
-
If A Tree Falls In The Forest Philosophy
May 23, 2025
-
Will Any Soldering Iron Work For Micro Soldering
May 23, 2025
-
Why Is My Furnace Blowing Cold Air
May 23, 2025
Related Post
Thank you for visiting our website which covers about Probability Distribution Of X And X_n . We hope the information provided has been useful to you. Feel free to contact us if you have any questions or need further assistance. See you next time and don't miss to bookmark.