Prove The Bayes Estimator Under Absolute Loss Is Median
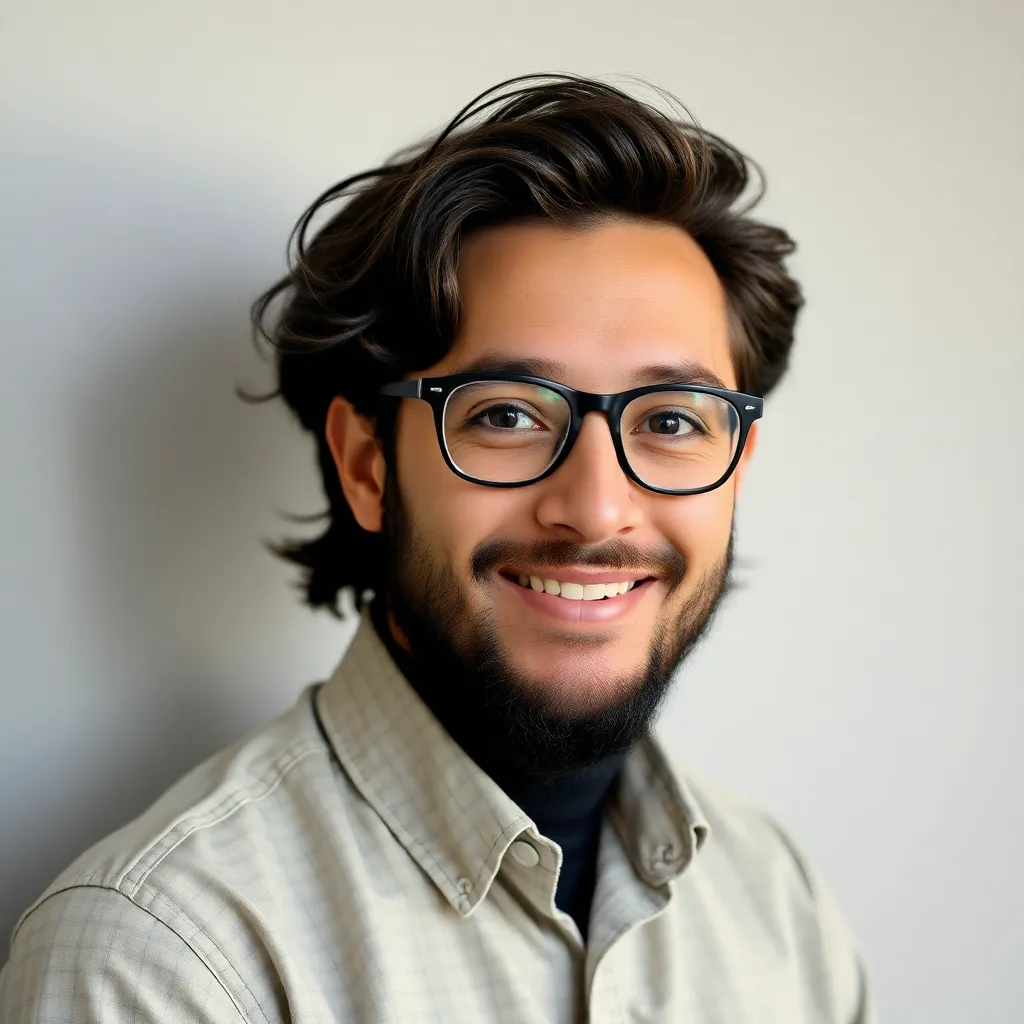
Kalali
May 23, 2025 · 3 min read
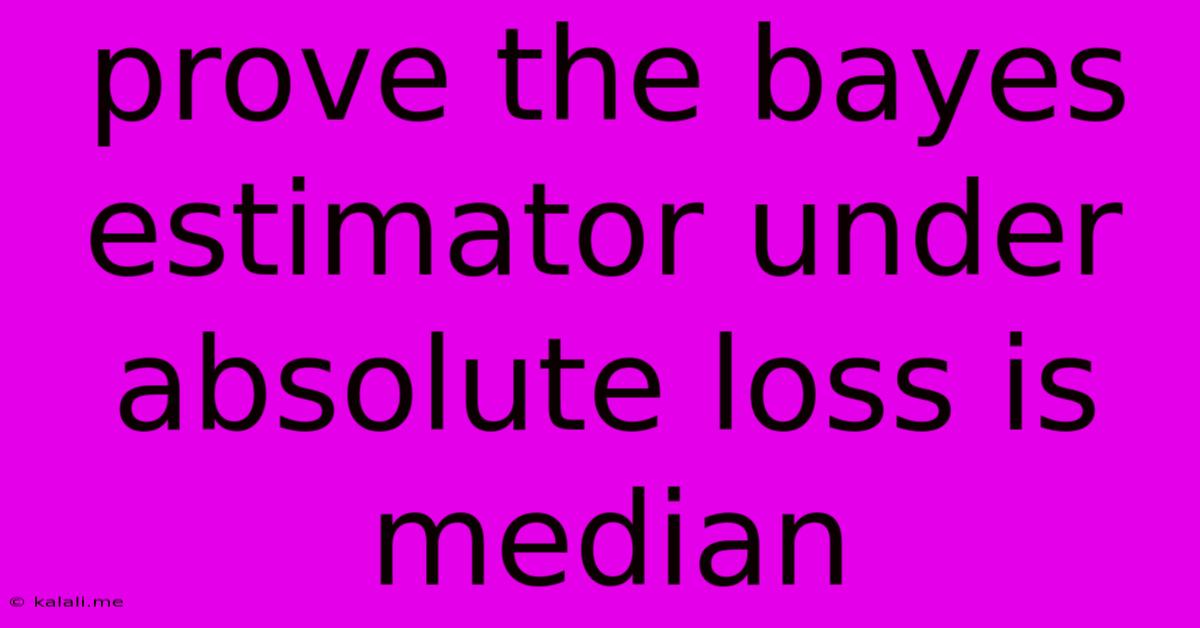
Table of Contents
Proving the Bayes Estimator Under Absolute Loss is the Median
This article will rigorously prove that under absolute loss, the Bayes estimator is the median of the posterior distribution. This is a fundamental result in Bayesian statistics with significant implications for decision-making under uncertainty. Understanding this proof enhances your grasp of Bayesian inference and optimal estimation.
What are we proving? We aim to demonstrate that for a parameter θ with a posterior distribution p(θ|x), the Bayes estimator under absolute loss, denoted as θ*, is the median of this posterior distribution. This means that the probability of θ being less than or equal to θ* is equal to the probability of θ being greater than or equal to θ*: P(θ ≤ θ* | x) = P(θ ≥ θ* | x) = 0.5.
Understanding the Components:
- Bayes Estimator: The Bayes estimator minimizes the expected posterior loss.
- Absolute Loss: The loss function is defined as L(θ, θ*) = |θ - θ*|, representing the absolute difference between the true parameter θ and the estimate θ*.
- Posterior Distribution: p(θ|x) is the probability distribution of the parameter θ given the observed data x. It is calculated using Bayes' theorem: p(θ|x) ∝ p(x|θ)p(θ), where p(x|θ) is the likelihood function and p(θ) is the prior distribution.
- Median: The median of a probability distribution is the value that divides the distribution into two equal halves.
The Proof:
The Bayes estimator θ* minimizes the expected posterior loss, which in the case of absolute loss is given by:
E[L(θ, θ*) | x] = ∫ |θ - θ*| p(θ|x) dθ
To minimize this expected loss, we can consider the derivative with respect to θ*. However, because the absolute value function is not differentiable at 0, we'll take a different approach. Let's break down the integral:
E[L(θ, θ*) | x] = ∫<sub>θ<θ*</sub> (θ* - θ) p(θ|x) dθ + ∫<sub>θ≥θ*</sub> (θ - θ*) p(θ|x) dθ
Now let's take the derivative with respect to θ* and set it to zero:
d/dθ* [∫<sub>θ<θ*</sub> (θ* - θ) p(θ|x) dθ + ∫<sub>θ≥θ*</sub> (θ - θ*) p(θ|x) dθ] = 0
Applying the Leibniz integral rule (carefully considering the change in integration limits):
∫<sub>θ<θ*</sub> p(θ|x) dθ - ∫<sub>θ≥θ*</sub> p(θ|x) dθ = 0
This simplifies to:
∫<sub>θ<θ*</sub> p(θ|x) dθ = ∫<sub>θ≥θ*</sub> p(θ|x) dθ
The left-hand side is P(θ < θ* | x), and the right-hand side is P(θ ≥ θ* | x). Therefore:
P(θ < θ* | x) = P(θ ≥ θ* | x)
Since these probabilities must sum to 1, we conclude that:
P(θ < θ* | x) = P(θ ≥ θ* | x) = 0.5
This proves that the Bayes estimator under absolute loss is the median of the posterior distribution.
Conclusion:
This proof demonstrates the fundamental relationship between the Bayes estimator, the absolute loss function, and the median of the posterior distribution. This result is crucial for Bayesian data analysis and provides a clear, intuitive way to choose an optimal estimator when absolute error is the primary concern. The median, as a robust measure of central tendency, is less sensitive to outliers than the mean, making it a valuable estimator in various applications. This understanding strengthens your ability to apply Bayesian methods effectively and interpret the results correctly.
Latest Posts
Latest Posts
-
Bg3 Do Downed Characters Get Xp
May 23, 2025
-
Keyboard Key Near The Top Left
May 23, 2025
-
Fresh Basil To Dried Basil Conversion
May 23, 2025
-
How To Increase Friendship In Pokerogue
May 23, 2025
-
How Long Does It Take To Bleed Brakes
May 23, 2025
Related Post
Thank you for visiting our website which covers about Prove The Bayes Estimator Under Absolute Loss Is Median . We hope the information provided has been useful to you. Feel free to contact us if you have any questions or need further assistance. See you next time and don't miss to bookmark.