Sum Of Squared Deviations From The Mean
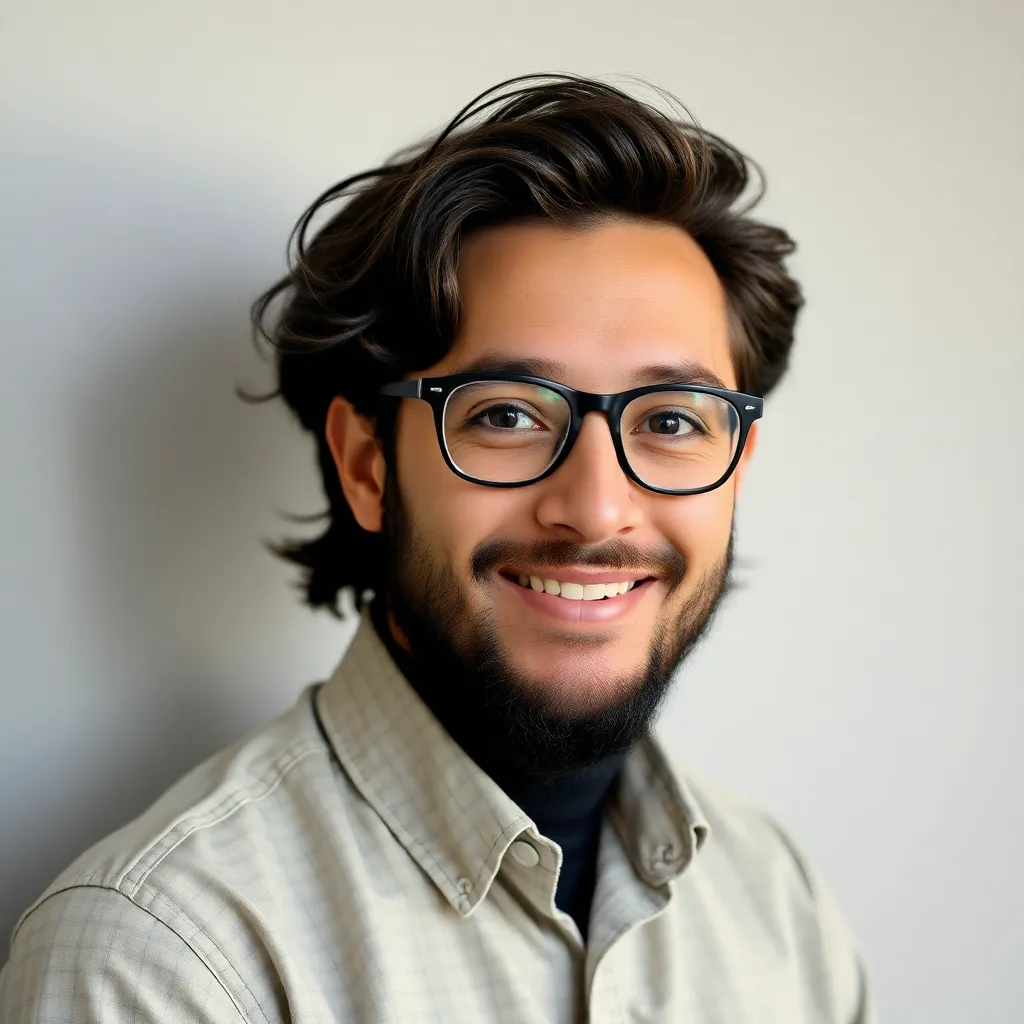
Kalali
Apr 27, 2025 · 6 min read
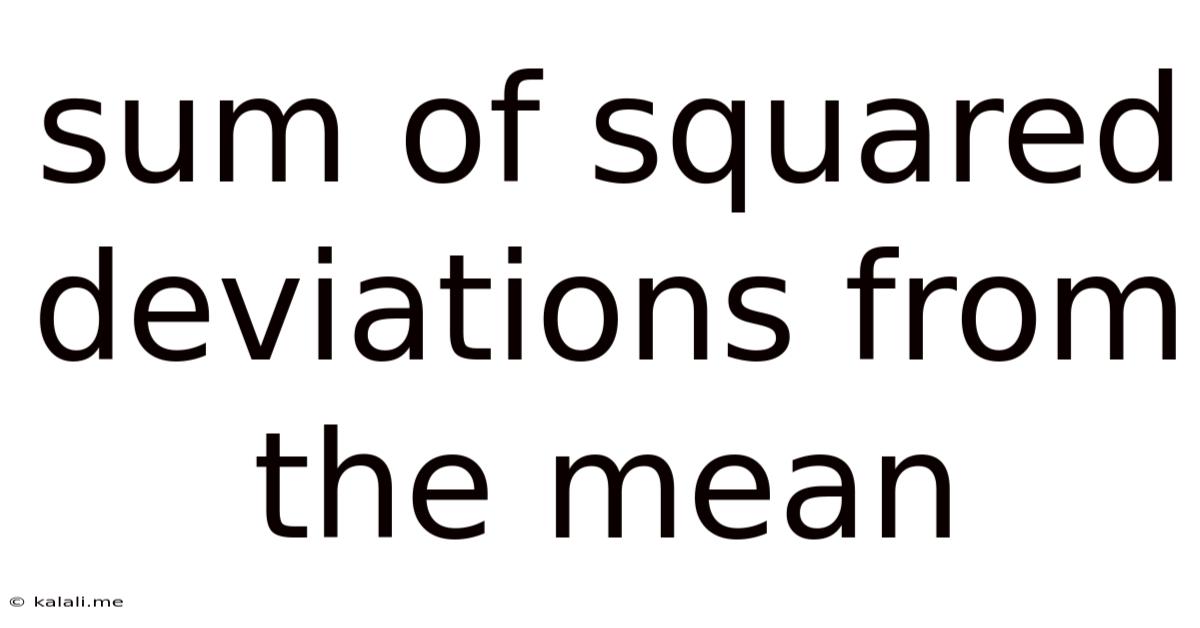
Table of Contents
Understanding the Sum of Squared Deviations from the Mean: A Comprehensive Guide
The sum of squared deviations from the mean (SS), often referred to as the sum of squares, is a fundamental concept in statistics. It forms the bedrock of many crucial statistical analyses, including variance, standard deviation, analysis of variance (ANOVA), and regression analysis. Understanding SS is vital for interpreting data and drawing meaningful conclusions. This article will provide a comprehensive explanation of the sum of squared deviations, its calculation, applications, and its significance in statistical modeling. We'll delve into the intricacies of this concept, clarifying its meaning and highlighting its importance in various statistical contexts.
What is the Sum of Squared Deviations from the Mean?
The sum of squared deviations from the mean represents the sum of the squared differences between each data point in a dataset and the mean of that dataset. In simpler terms, it quantifies the total dispersion or spread of the data points around the central tendency (the mean). A larger SS indicates greater variability in the data, while a smaller SS suggests less variability. This concept is crucial because it directly relates to the variance and standard deviation, which are widely used measures of data dispersion.
Calculating the Sum of Squared Deviations (SS)
The calculation of SS is straightforward, although it can be tedious for large datasets. The steps are as follows:
-
Calculate the mean (average) of the data set: Sum all the data points and divide by the number of data points.
-
Find the deviation of each data point from the mean: Subtract the mean from each individual data point.
-
Square each deviation: Square each of the deviations calculated in step 2. This eliminates negative values, ensuring that all deviations contribute positively to the sum.
-
Sum the squared deviations: Add up all the squared deviations. This final sum is the sum of squared deviations (SS).
Formula for Sum of Squared Deviations:
The formula for calculating the sum of squared deviations can be expressed as:
SS = Σ(xᵢ - μ)²
Where:
- Σ represents the summation symbol, indicating the sum of all values.
- xᵢ represents each individual data point in the dataset.
- μ represents the mean (average) of the dataset.
Example Calculation:
Let's consider a small dataset: {2, 4, 6, 8, 10}.
-
Mean (μ): (2 + 4 + 6 + 8 + 10) / 5 = 6
-
Deviations from the mean:
- 2 - 6 = -4
- 4 - 6 = -2
- 6 - 6 = 0
- 8 - 6 = 2
- 10 - 6 = 4
-
Squared deviations:
- (-4)² = 16
- (-2)² = 4
- (0)² = 0
- (2)² = 4
- (4)² = 16
-
Sum of squared deviations (SS): 16 + 4 + 0 + 4 + 16 = 40
Therefore, the sum of squared deviations for this dataset is 40.
Degrees of Freedom and the Sum of Squares
The concept of degrees of freedom (df) is intrinsically linked to the sum of squares. Degrees of freedom represent the number of independent pieces of information available to estimate a parameter. When calculating the sum of squares, one degree of freedom is lost because the mean is used in the calculation. Therefore, the degrees of freedom for the sum of squares is n-1, where n is the number of data points.
Relationship to Variance and Standard Deviation
The sum of squared deviations is directly related to variance and standard deviation, two fundamental measures of data dispersion.
-
Variance: The variance (σ²) is calculated by dividing the sum of squared deviations by the degrees of freedom (n-1). This provides an average measure of the squared deviations.
σ² = SS / (n - 1)
-
Standard Deviation: The standard deviation (σ) is the square root of the variance. It represents the average distance of data points from the mean, expressed in the original units of the data.
σ = √(SS / (n - 1))
Applications of the Sum of Squared Deviations
The sum of squared deviations plays a critical role in various statistical analyses:
-
Analysis of Variance (ANOVA): ANOVA utilizes the sum of squares to compare the means of two or more groups. It partitions the total sum of squares into different sources of variation, allowing researchers to determine if significant differences exist between group means. This is widely used in experimental design and hypothesis testing.
-
Regression Analysis: In regression analysis, the sum of squared deviations is used to assess the goodness of fit of a model. The total sum of squares is partitioned into the explained sum of squares (due to the model) and the residual sum of squares (unexplained variation). The ratio of these sums of squares forms the basis of the R-squared statistic, a measure of how well the model explains the variation in the data.
-
Principal Component Analysis (PCA): PCA uses the sum of squared deviations to find the principal components, which are new uncorrelated variables that capture the maximum variance in the data. This is used for dimensionality reduction and data visualization.
-
Hypothesis Testing: The sum of squares is frequently used in various hypothesis tests to assess the statistical significance of observed differences or relationships.
Interpreting the Sum of Squared Deviations
The magnitude of the sum of squared deviations provides insights into data variability. A larger SS indicates greater dispersion around the mean, suggesting higher variability within the dataset. Conversely, a smaller SS indicates less dispersion, suggesting lower variability. However, it's important to interpret SS in conjunction with the sample size and the scale of the data. Comparing SS values across datasets with different sample sizes or scales can be misleading without considering these factors. Therefore, it's often more informative to use variance or standard deviation, which are standardized measures of variability.
Advantages and Disadvantages of Using SS
Advantages:
- Fundamental Concept: SS is a fundamental concept that underlies many crucial statistical analyses.
- Simple Calculation: The calculation is relatively straightforward, although it can become computationally intensive for very large datasets.
- Intuitive Interpretation: A larger SS implies greater variability, which is generally easy to grasp intuitively.
Disadvantages:
- Scale-Dependent: The magnitude of SS is sensitive to the scale of the data. This makes comparing SS across datasets with different scales challenging.
- Not Standardized: SS itself is not a standardized measure; therefore, direct comparisons across datasets with different sample sizes may not be meaningful.
- Sensitive to Outliers: SS is significantly influenced by outliers, as squaring the deviations amplifies the effect of extreme values.
Conclusion
The sum of squared deviations from the mean is a fundamental concept in statistics with widespread applications in various analytical techniques. Understanding its calculation, interpretation, and relationship to variance and standard deviation is essential for anyone working with data analysis and statistical modeling. While it has limitations, especially regarding scale dependence and sensitivity to outliers, its role in underpinning many key statistical methods remains undeniable. By mastering the concept of SS, you gain a deeper understanding of data variability and the tools used to quantify and interpret it. This allows for more robust and meaningful statistical inferences.
Latest Posts
Latest Posts
-
What Percent Is 49 Of 50
Apr 28, 2025
-
How Many Hours Is 345 Minutes
Apr 28, 2025
-
6 Gallons Is How Many Cups
Apr 28, 2025
-
Cuanto Es Un Galon En Cuartos
Apr 28, 2025
-
How Much Is 8 5 Cm In Inches
Apr 28, 2025
Related Post
Thank you for visiting our website which covers about Sum Of Squared Deviations From The Mean . We hope the information provided has been useful to you. Feel free to contact us if you have any questions or need further assistance. See you next time and don't miss to bookmark.