What Are Class Limits In Statistics
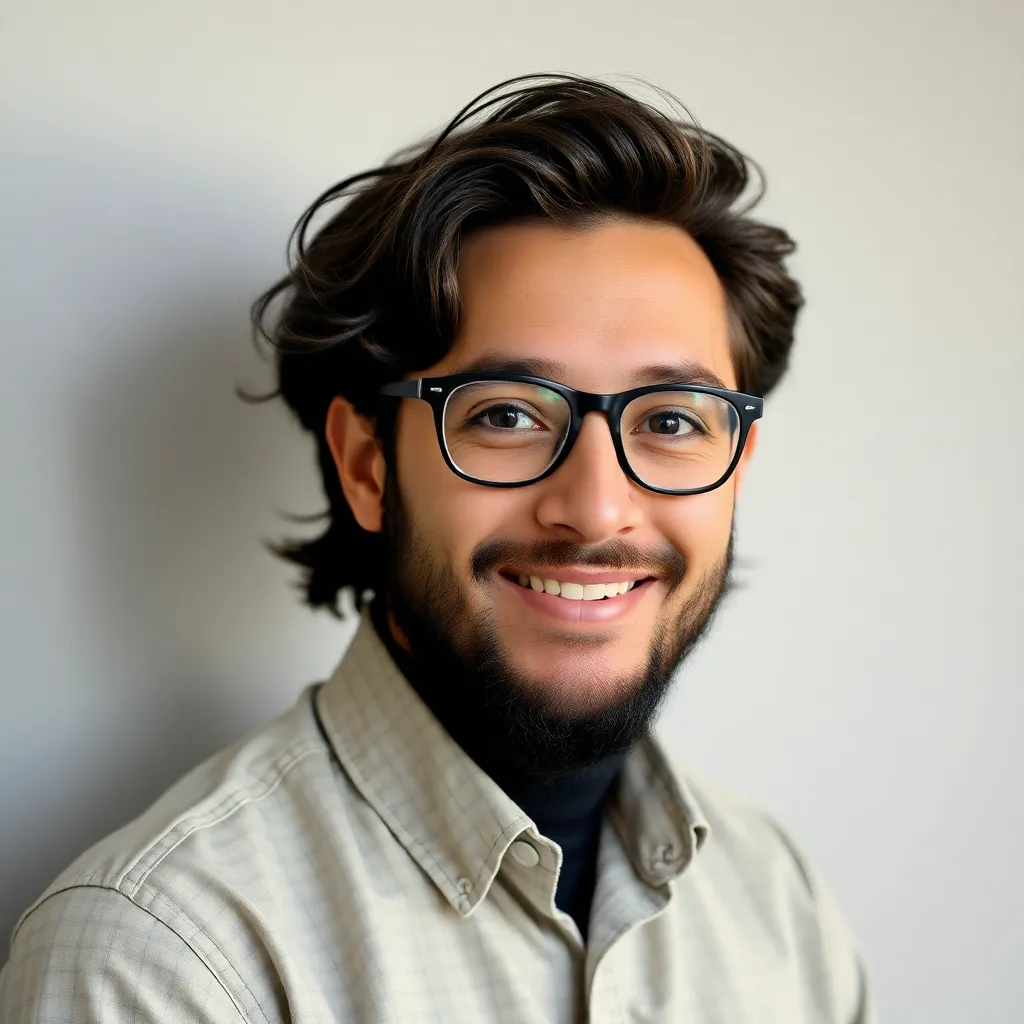
Kalali
Apr 22, 2025 · 5 min read
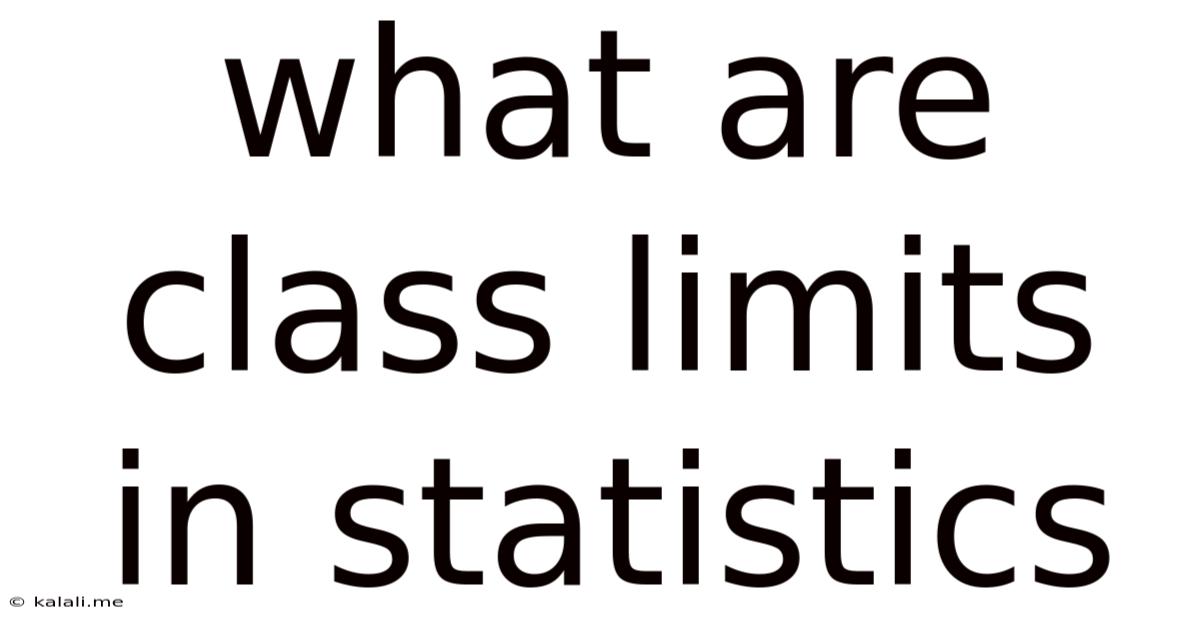
Table of Contents
Understanding Class Limits in Statistics: A Comprehensive Guide
Understanding class limits is crucial for anyone working with grouped data in statistics. This comprehensive guide will delve deep into the concept of class limits, explaining their significance, how to determine them, and their role in various statistical analyses. We'll explore different types of class limits, common misconceptions, and practical applications to solidify your understanding. By the end, you'll be confident in interpreting and utilizing class limits in your own statistical work.
What are Class Limits?
In statistics, particularly when dealing with large datasets, raw data is often organized into frequency distributions. This involves grouping data points into classes or intervals. Class limits define the boundaries of each class interval. They specify the minimum and maximum values that a data point can have to belong to a particular class. Understanding these boundaries is vital for accurately interpreting the distribution and performing further statistical calculations.
Types of Class Limits:
There are two main types of class limits:
-
Apparent Class Limits: These are the values directly stated as the boundaries of a class interval. They represent the easily visible limits of a class and are typically the values used to create a frequency distribution table or histogram.
-
Real Class Limits: These limits are adjusted apparent class limits used to ensure that there are no gaps between consecutive classes in a frequency distribution. They are especially important when working with continuous data. They effectively account for the precision of measurement. For instance, if your apparent class limits are 10-19, the real class limits might be 9.5 - 19.5, ensuring no data points are left uncategorized.
Determining Class Limits:
The process of determining class limits involves several steps:
-
Determine the Range: Find the difference between the highest and lowest values in your dataset. This range provides the basis for determining the number of classes and their width.
-
Determine the Number of Classes: The optimal number of classes depends on the size of your dataset and the level of detail required. Common rules of thumb include Sturges' formula (k = 1 + 3.322 log n, where k is the number of classes and n is the number of data points) or using between 5 and 20 classes. The choice depends on context and the nature of the data.
-
Determine the Class Width: Divide the range by the chosen number of classes. Round up the result to a convenient value to ensure that all data points are included within the classes.
-
Determine the Apparent Class Limits: Starting from the minimum value, define the upper and lower boundaries for each class, using the calculated class width. Ensure there's no overlap between classes.
-
Determine the Real Class Limits: Adjust the apparent class limits to eliminate gaps between classes. This often involves adding or subtracting half of the measurement unit. For instance, if your data is measured to the nearest whole number, add or subtract 0.5.
Example: Calculating Class Limits
Let's consider a dataset representing the weights (in kilograms) of 50 students:
Data: 50, 52, 55, 58, 60, 62, 65, 68, 70, 72, 75, 78, 80, 82, 85, 51, 53, 56, 59, 61, 63, 66, 69, 71, 73, 76, 79, 81, 83, 86, 49, 54, 57, 64, 67, 74, 77, 84, 87, 50, 52, 55, 58, 60, 62, 65, 68, 70, 72, 75
-
Range: The highest value is 87, and the lowest is 49. The range is 87 - 49 = 38.
-
Number of Classes: Let's use Sturges' formula: k = 1 + 3.322 log(50) ≈ 6.65. We'll round up to 7 classes.
-
Class Width: 38 / 7 ≈ 5.43. We'll round up to 6 for convenience.
-
Apparent Class Limits: Starting from 49, we can define the classes as:
- 49-54
- 55-60
- 61-66
- 67-72
- 73-78
- 79-84
- 85-90
-
Real Class Limits: Since the data is measured to the nearest kilogram, we adjust by 0.5:
- 48.5-54.5
- 54.5-60.5
- 60.5-66.5
- 66.5-72.5
- 72.5-78.5
- 78.5-84.5
- 84.5-90.5
Notice how the real class limits ensure there are no gaps between consecutive classes. A weight of 54.5kg would belong to the second class using real class limits, avoiding ambiguity.
Importance of Class Limits:
Accurate class limits are essential for several reasons:
-
Accurate Frequency Distributions: They ensure all data points are correctly categorized, avoiding data loss or misrepresentation.
-
Reliable Statistical Calculations: Calculations like mean, median, and standard deviation, when applied to grouped data, rely heavily on accurate class limits. Incorrect limits will lead to inaccurate results.
-
Clear Data Visualization: Histograms and frequency polygons, commonly used to visualize grouped data, require precise class limits for accurate representation.
-
Avoiding Ambiguity: Real class limits eliminate the ambiguity associated with data points falling exactly on the apparent class boundaries.
Common Misconceptions about Class Limits:
-
Ignoring Real Class Limits: Many beginners focus solely on apparent class limits, leading to inaccuracies in statistical analysis and data visualization.
-
Overlapping Class Limits: Classes should be mutually exclusive; a data point should belong to only one class. Overlapping limits create confusion and errors.
-
Incorrect Rounding: Incorrect rounding of the class width can lead to unequal class intervals and inaccurate results.
Advanced Applications:
Class limits play a crucial role in more advanced statistical concepts:
-
Data Smoothing: Class limits are fundamental in techniques that smooth out data variations, such as kernel density estimation.
-
Hypothesis Testing: When dealing with grouped data in hypothesis tests, accurate class limits are necessary for calculating test statistics.
-
Regression Analysis: In some cases, class limits can be used to create categorical predictors in regression models.
Conclusion:
Understanding class limits is fundamental to working with grouped data in statistics. Mastering the distinction between apparent and real class limits, along with the procedures for determining them accurately, is crucial for performing reliable statistical analyses and creating accurate data visualizations. By paying close attention to detail in defining and using class limits, you can ensure the validity and accuracy of your statistical findings. Remember to always choose the appropriate method and consider the precision of your data when determining class limits. Ignoring these details can lead to significant errors in your statistical work. The examples and explanations provided in this article should equip you to confidently handle class limits in your future statistical endeavors.
Latest Posts
Latest Posts
-
How Much Is 110 Cm In Inches
Apr 22, 2025
-
What Is The Lcm Of 3 And 4
Apr 22, 2025
-
40 Is What Percent Of 100
Apr 22, 2025
-
How Does Biosphere Interact With Hydrosphere
Apr 22, 2025
-
What Is 93 Inches In Feet
Apr 22, 2025
Related Post
Thank you for visiting our website which covers about What Are Class Limits In Statistics . We hope the information provided has been useful to you. Feel free to contact us if you have any questions or need further assistance. See you next time and don't miss to bookmark.