What Is The Mle Of Geometric
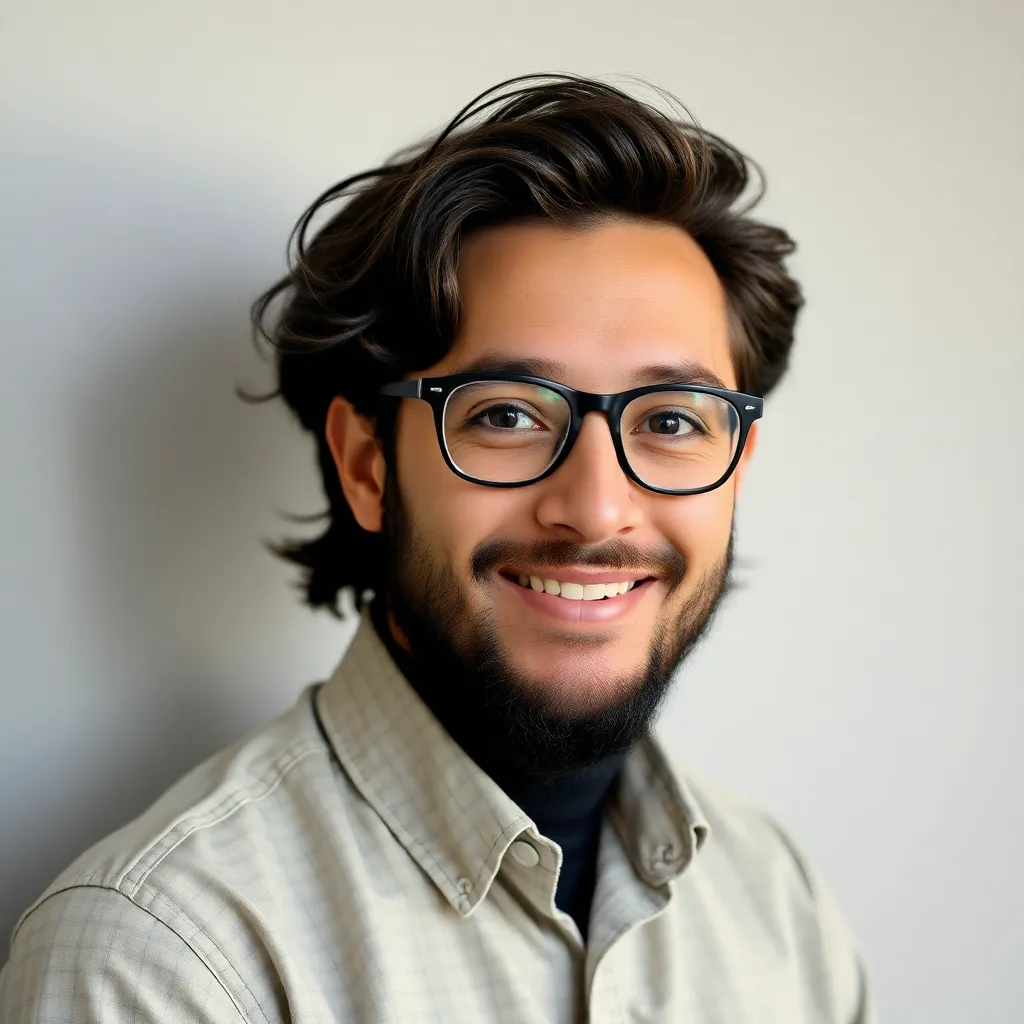
Kalali
May 24, 2025 · 3 min read
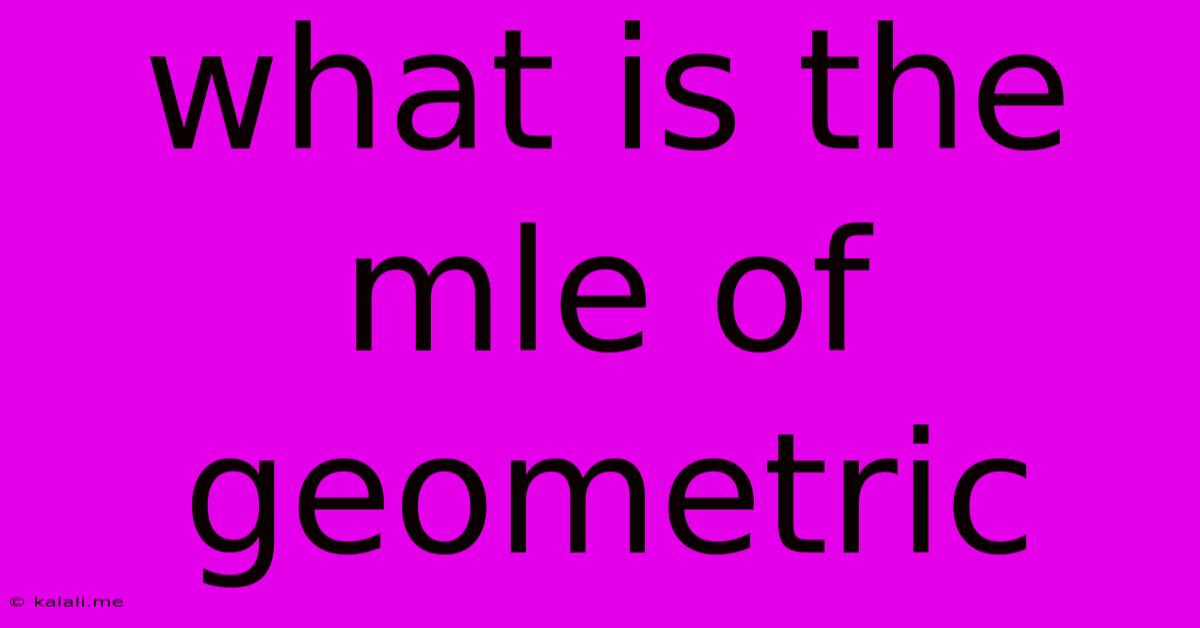
Table of Contents
What is the MLE of Geometric Distribution?
The Maximum Likelihood Estimation (MLE) is a powerful statistical method used to estimate the parameters of a probability distribution given a dataset. This article will delve into the specifics of deriving the MLE for the parameter of a geometric distribution. Understanding this process provides valuable insight into both MLE and the geometric distribution itself. This guide will walk you through the process step-by-step, making it accessible even for those with limited statistical background.
Understanding the Geometric Distribution
The geometric distribution models the probability of the number of trials needed to get the first success in a sequence of independent Bernoulli trials, where each trial has a constant probability of success, denoted by 'p'. This means that each trial is either a success (with probability p) or a failure (with probability 1-p). The probability mass function (PMF) of a geometric distribution is:
P(X = k) = (1-p)^(k-1) * p, where k = 1, 2, 3...
Here, 'k' represents the number of trials until the first success. The parameter 'p' is what we aim to estimate using the MLE method. Common applications include modeling the number of attempts needed to achieve a goal, like the number of times you flip a coin before getting heads.
Deriving the MLE for the Geometric Distribution Parameter (p)
Let's say we have a sample of 'n' independent observations from a geometric distribution: x₁, x₂, ..., xₙ. To find the MLE of 'p', we first construct the likelihood function, which is the joint probability of observing this sample given a particular value of 'p'. Since the observations are independent, the likelihood function is the product of the individual probabilities:
L(p | x₁, x₂, ..., xₙ) = Πᵢ₌₁ⁿ [(1-p)^(xᵢ-1) * p]
To simplify calculations, we usually work with the log-likelihood function, denoted as ℓ(p):
ℓ(p) = log[L(p | x₁, x₂, ..., xₙ)] = Σᵢ₌₁ⁿ [ (xᵢ - 1)log(1-p) + log(p) ]
Now, to find the MLE, we take the derivative of the log-likelihood function with respect to 'p', set it to zero, and solve for 'p':
dℓ(p)/dp = Σᵢ₌₁ⁿ [ -(xᵢ - 1)/(1-p) + 1/p ] = 0
Solving this equation involves some algebraic manipulation. After simplification, we arrive at:
Σᵢ₌₁ⁿ (xᵢ - 1) = (Σᵢ₌₁ⁿ xᵢ - n) = n/p * (1 - p)
This simplifies further to:
p̂ = 1 / ( Σᵢ₌₁ⁿ xᵢ / n) = n / Σᵢ₌₁ⁿ xᵢ
Therefore, the Maximum Likelihood Estimator (MLE) for the parameter 'p' of a geometric distribution is the reciprocal of the sample mean: p̂ = n / Σᵢ₌₁ⁿ xᵢ
This intuitively makes sense. A higher sample mean suggests lower probability of success (smaller p), and vice-versa.
Interpreting the Result
The MLE, p̂, provides the most likely value for the probability of success ('p') based on the observed data. It's important to remember that this is an estimate, and the true value of 'p' might be slightly different. The accuracy of this estimate increases with the size of the sample ('n').
In Conclusion
The derivation of the MLE for the geometric distribution parameter demonstrates a fundamental application of maximum likelihood estimation. Understanding this process allows for the robust estimation of key parameters in various probabilistic models, providing crucial insights into the underlying data. Remember to always consider the limitations of your estimate and the potential for sampling error when interpreting results.
Latest Posts
Latest Posts
-
Should Rotors Be Replaced With Brake Pads
May 24, 2025
-
Is Eigen Vector Princinpal Direct Or Principal Component
May 24, 2025
-
I Wash My Hands Of This
May 24, 2025
-
Another Word For Player In Dating
May 24, 2025
-
Ac Unit Cycles On And Off
May 24, 2025
Related Post
Thank you for visiting our website which covers about What Is The Mle Of Geometric . We hope the information provided has been useful to you. Feel free to contact us if you have any questions or need further assistance. See you next time and don't miss to bookmark.