Cumulative Link Mixed Models Threshold Coefficients
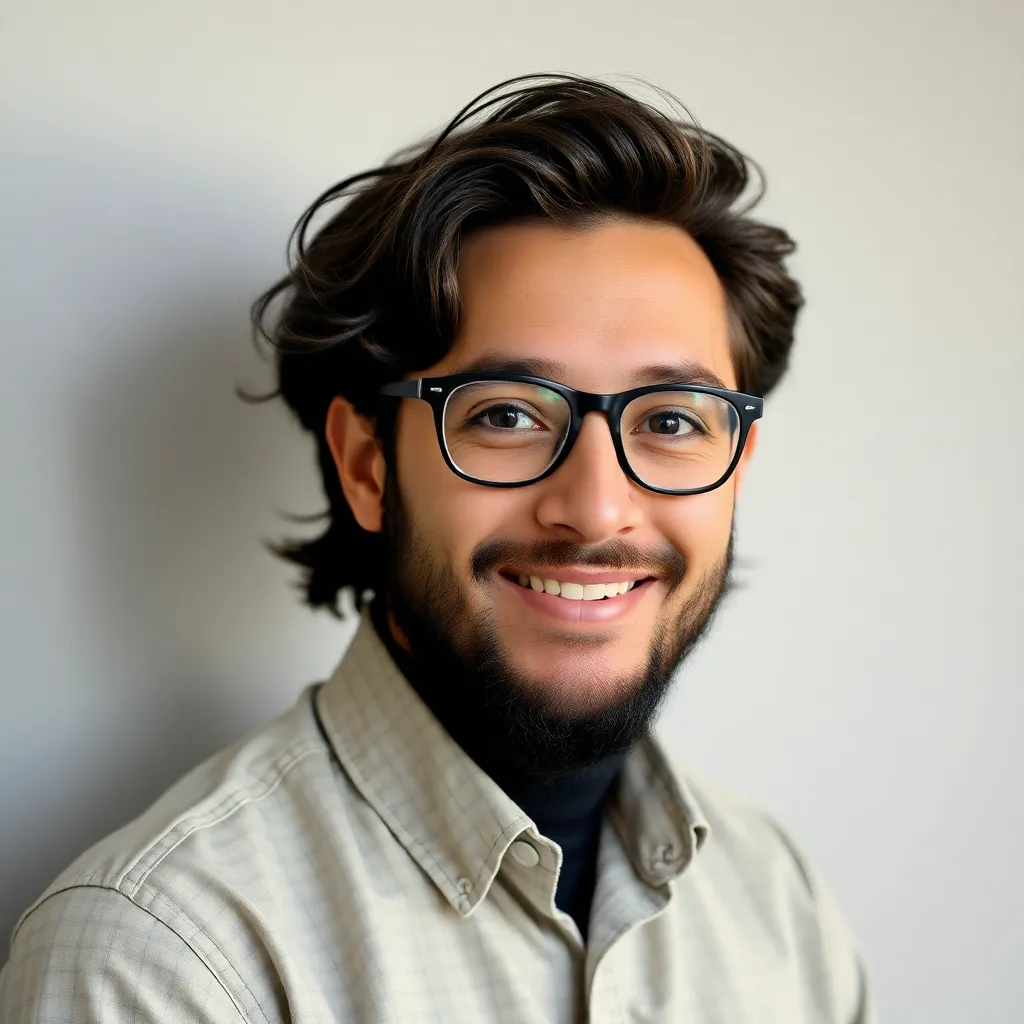
Kalali
May 24, 2025 · 3 min read
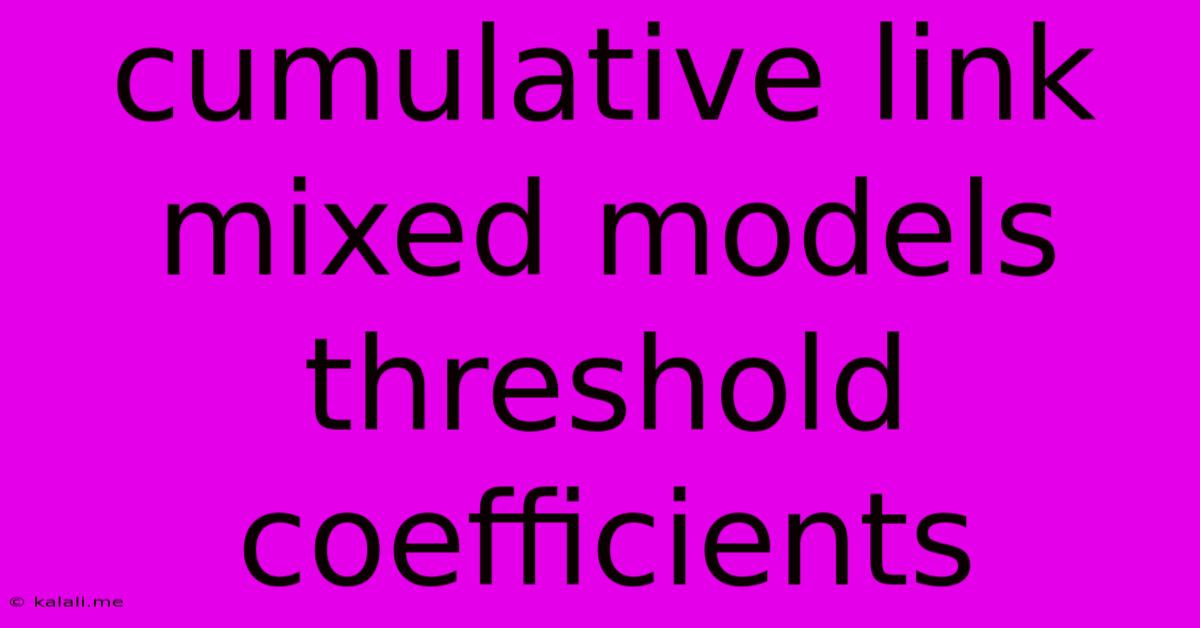
Table of Contents
Understanding Cumulative Link Mixed Models and Threshold Coefficients
This article delves into the intricacies of cumulative link mixed models (CLMMs) and their crucial component: threshold coefficients. CLMMs are powerful statistical tools used to analyze ordinal dependent variables, meaning variables with ordered categories. Understanding threshold coefficients is key to interpreting the results of these models and drawing meaningful conclusions from your data. This explanation will be beneficial for researchers working with ordered response data in fields like psychology, sociology, and education.
What are Cumulative Link Mixed Models?
CLMMs are an extension of generalized linear mixed models (GLMMs) specifically designed for ordinal data. Unlike models that treat ordinal data as continuous or nominal, CLMMs acknowledge the ordered nature of the categories. They do this by modeling the cumulative probabilities of observing a response in a particular category or below. This approach accounts for the inherent ordering and avoids potential biases associated with simplifying ordinal data. A key advantage of CLMMs is their ability to incorporate random effects, acknowledging the clustering or correlation within the data. This is particularly important when dealing with repeated measures or hierarchical data structures.
Threshold Coefficients: The Key to Interpretation
At the heart of a CLMM lie the threshold coefficients. These coefficients define the cut-points on the latent continuous scale that separates the ordered categories of the ordinal dependent variable. Imagine an underlying continuous variable that we cannot directly observe, but which influences the observed ordinal outcome. The threshold coefficients represent the points on this latent scale where the probability of belonging to a higher category surpasses the probability of belonging to the lower category.
How Threshold Coefficients Work:
Let's say we have an ordinal dependent variable with three categories (low, medium, high). The CLMM will estimate two threshold coefficients, denoted as τ₁
and τ₂
. These are interpreted as follows:
-
τ₁
: This is the cut-point between the "low" and "medium" categories. If the latent continuous variable is belowτ₁
, the observation is classified as "low." If it's aboveτ₁
, it's classified as "medium" or "high". -
τ₂
: This is the cut-point between "medium" and "high". If the latent continuous variable is belowτ₂
, the observation is classified as "low" or "medium." If it's aboveτ₂
, it's classified as "high."
Interpreting the Threshold Coefficients:
The exact interpretation of threshold coefficients depends on the model and the context of the study. However, some general guidelines apply:
-
Magnitude: Larger absolute values of threshold coefficients indicate wider separations between categories. A large difference between two adjacent threshold coefficients suggests a clear distinction between those two specific categories.
-
Sign: The sign of a threshold coefficient (positive or negative) usually doesn't carry much direct substantive meaning in most applications. It's the relative positions of these coefficients that matter.
-
In Relation to Predictors: The influence of predictor variables on the threshold coefficients is key. A significant effect of a predictor variable on a specific threshold suggests that this predictor has a strong impact on the probability of crossing the boundary between the corresponding categories. For example, a positive effect on
τ₁
suggests the predictor increases the probability of moving from "low" to "medium" or higher.
Software for CLMMs:
Several statistical software packages can fit CLMMs. Popular choices include R (using packages like ordinal
or lme4
), SAS (using PROC GLIMMIX), and Stata (using meologit
).
Conclusion:
Cumulative link mixed models provide a robust and statistically sound method for analyzing ordinal dependent variables. Understanding the role and interpretation of threshold coefficients is vital for accurately interpreting the results and drawing meaningful conclusions from your data. By carefully examining these coefficients in relation to predictor variables, researchers can gain valuable insights into the factors influencing the ordered categorical outcome. Remember to always consider the specific context of your study and use appropriate visualization techniques to aid in the interpretation of your results.
Latest Posts
Latest Posts
-
How To Make An Image Transparent In Google Slides
May 24, 2025
-
How To Type Theta On Mac
May 24, 2025
-
How To Clean A Whirlpool Tub
May 24, 2025
-
Where To Get Fire Extinguisher Recharged
May 24, 2025
-
How To Sharpen A Lawn Mower Blade
May 24, 2025
Related Post
Thank you for visiting our website which covers about Cumulative Link Mixed Models Threshold Coefficients . We hope the information provided has been useful to you. Feel free to contact us if you have any questions or need further assistance. See you next time and don't miss to bookmark.