Expectation And Function Order Exchange Jensen
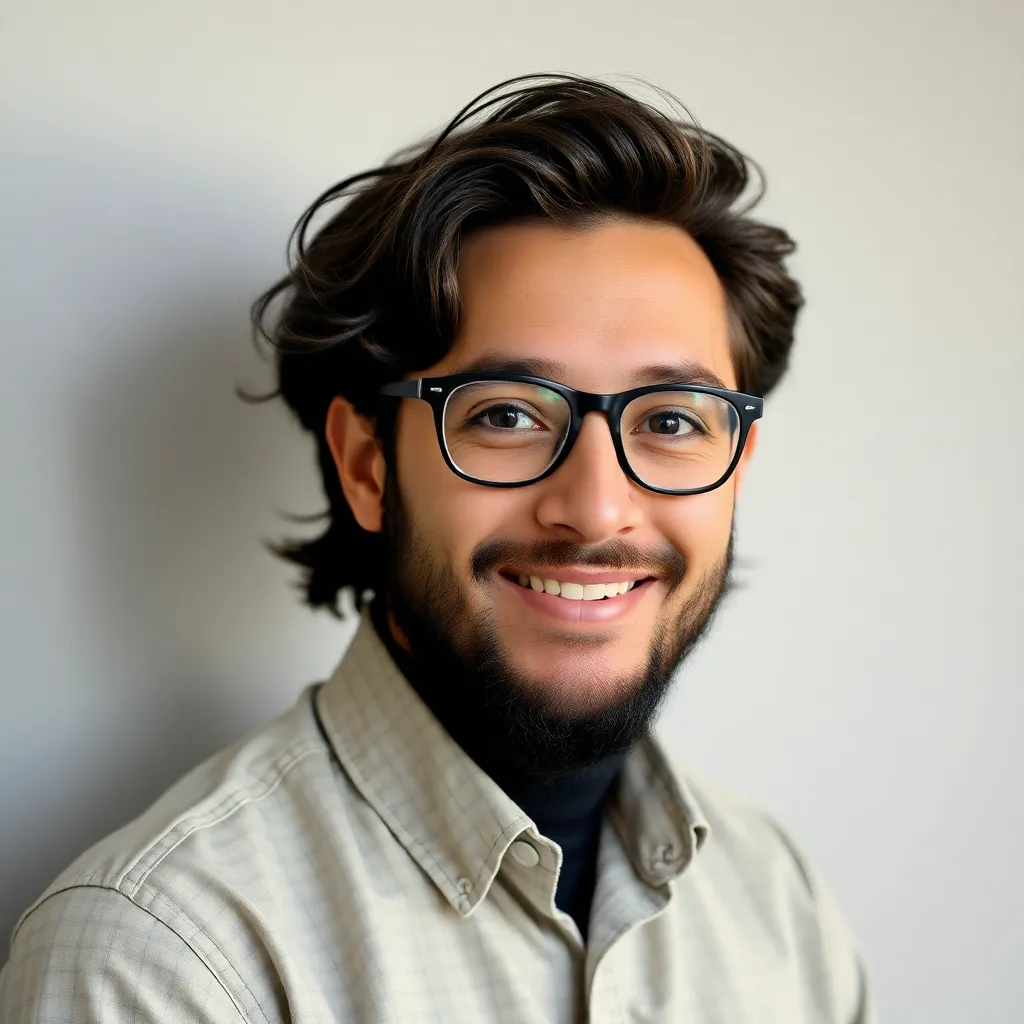
Kalali
May 23, 2025 · 3 min read
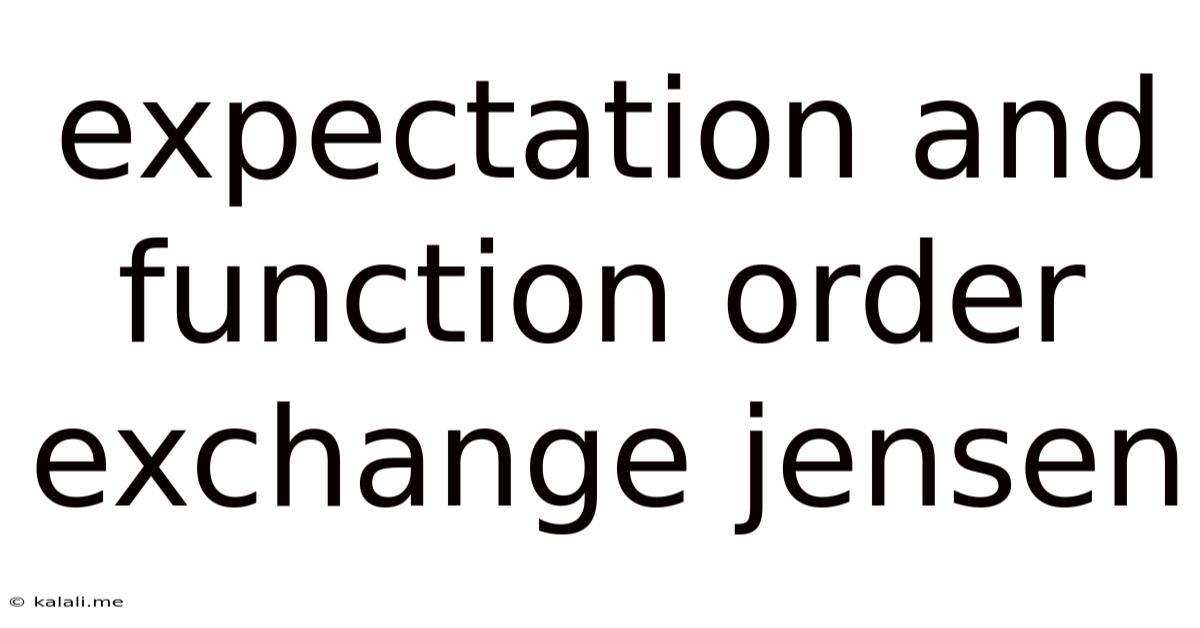
Table of Contents
Expectation and Function Order Exchange: Understanding Jensen's Work
This article delves into the fascinating concept of expectation and function order exchange, particularly as explored by Jensen and others. We'll explore the mathematical underpinnings, practical applications, and potential pitfalls of this powerful technique. Understanding this concept is crucial for anyone working with probability, statistics, and various fields of applied mathematics.
What is Expectation and Function Order Exchange?
At its core, the expectation and function order exchange (also sometimes referred to as the linearity of expectation or the law of the unconscious statistician) deals with the order in which we apply a function and an expectation operator to a random variable. Simply put, under certain conditions, we can swap the order of these operations without altering the result. This is incredibly useful because it often simplifies complex calculations. Formally, if X is a random variable and g is a function, then the theorem states:
E[g(X)] = ∫g(x)f<sub>X</sub>(x)dx
where f<sub>X</sub>(x) is the probability density function (PDF) of X. For discrete random variables, the integral becomes a summation.
Conditions for Valid Exchange
The key to successfully exchanging the expectation and function order lies in fulfilling specific conditions. The validity of the exchange depends heavily on the properties of both the random variable X and the function g. The most common sufficient conditions include:
- g(X) is integrable: This means that the expected value of |g(X)| is finite. This ensures the integral (or sum) converges.
- X has a well-defined expectation: The expectation of X itself must exist. This is often straightforward for common probability distributions.
- g is a measurable function: This condition, while technically important, often holds true for the functions encountered in practical applications. It essentially guarantees that the composition g(X) is a well-defined random variable.
Practical Applications and Examples
The expectation and function order exchange theorem finds applications in diverse areas:
- Calculating moments: Finding the variance of a random variable often involves calculating E[X²]. The theorem allows us to calculate this easily by evaluating the integral (or sum) of x² times the probability density function.
- Analyzing transformed variables: If we apply a transformation to a random variable (e.g., taking the square root or logarithm), this theorem helps find the expectation of the transformed variable.
- Monte Carlo simulations: In simulations, this theorem provides a crucial theoretical foundation for estimating expectations using sample averages.
- Machine learning: The concept underpins various algorithms, aiding in the analysis of model performance and prediction accuracy.
Potential Pitfalls and Limitations
While a powerful tool, misapplication can lead to erroneous results. Failing to satisfy the necessary conditions will invalidate the exchange. It's crucial to verify that the chosen function g and the random variable X meet the criteria before applying the theorem. Furthermore, there are situations where the direct calculation of E[g(X)] might be simpler than using the exchanged form.
Beyond Jensen's Inequality
While closely related to the topic of expectation and function order exchange, Jensen's inequality provides a further refinement and offers insights into the relationship between the expectation of a function and the function of an expectation. It states that for a convex function, the expectation of the function is greater than or equal to the function of the expectation.
Conclusion
The ability to exchange the expectation and function order is a fundamental concept in probability and statistics. By understanding the underlying conditions and applying it judiciously, researchers and practitioners can simplify complex calculations, analyze transformed variables, and build robust mathematical models. Remember that careful consideration of the conditions is paramount to avoid errors and ensure the accuracy of results.
Latest Posts
Latest Posts
-
How To Write A Matrix In Latex
May 24, 2025
-
Where To Connect C Wire On Furnace
May 24, 2025
-
Convert Eigen Vector Matrix To Angle
May 24, 2025
-
Pathfinder 2e Magic Items Increase Save Dc
May 24, 2025
-
Can You Put Water Based Polyurethane Over Oil Based Polyurethane
May 24, 2025
Related Post
Thank you for visiting our website which covers about Expectation And Function Order Exchange Jensen . We hope the information provided has been useful to you. Feel free to contact us if you have any questions or need further assistance. See you next time and don't miss to bookmark.