Find A Rao Cramer Bound For 1/theta Instead Of Theta
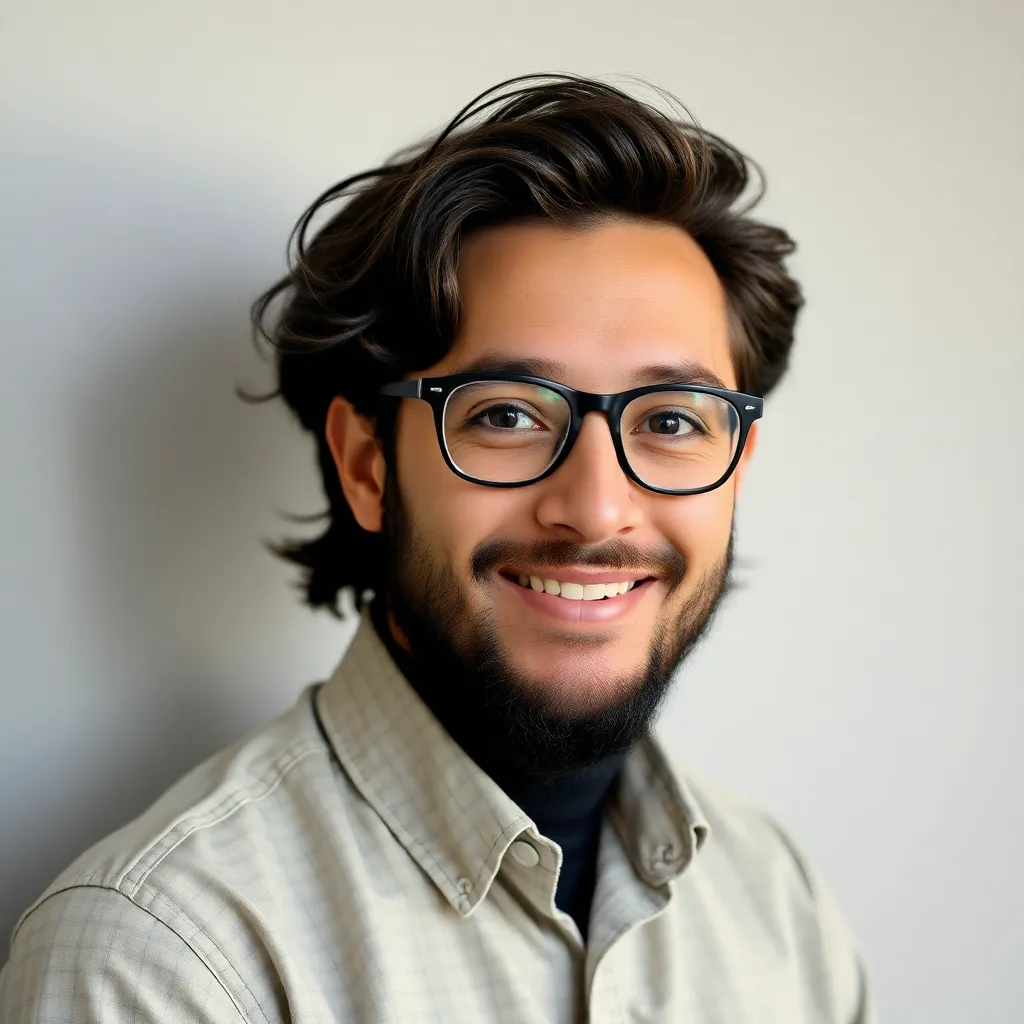
Kalali
May 23, 2025 · 3 min read
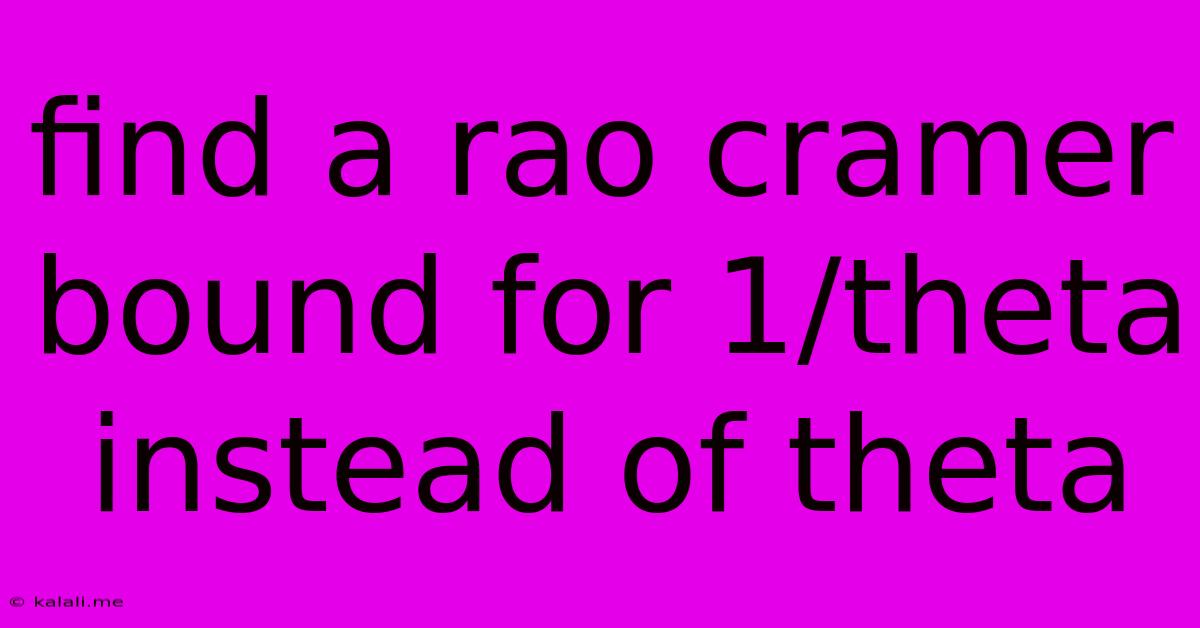
Table of Contents
Finding the Rao-Cramer Lower Bound for 1/θ Instead of θ
The Rao-Cramer Lower Bound (RCLB) is a fundamental concept in statistical inference, providing a lower bound on the variance of any unbiased estimator of a parameter. Typically, we derive the RCLB for the parameter itself (e.g., θ). However, situations arise where we need the RCLB for a function of the parameter, such as 1/θ. This article will guide you through the process of finding the RCLB for 1/θ, focusing on the necessary adjustments to the standard approach. This is particularly relevant in scenarios involving exponential distributions or other models where the reciprocal of the parameter is of direct interest.
Understanding the Rao-Cramer Lower Bound
Before diving into the specific case of 1/θ, let's briefly revisit the core idea of the RCLB. For an unbiased estimator T of a parameter θ, based on a random sample from a probability distribution with probability density function (pdf) or probability mass function (pmf) f(x;θ), the RCLB states that:
Var(T) ≥ 1/I(θ)
where I(θ) is the Fisher information, defined as:
I(θ) = E[(∂/∂θ log f(X;θ))²] = -E[(∂²/∂θ² log f(X;θ))]
The second equality holds under regularity conditions. The Fisher information essentially quantifies the amount of information the data contains about the parameter θ. A higher Fisher information indicates a more precise estimation is possible.
Deriving the RCLB for 1/θ
To find the RCLB for g(θ) = 1/θ, we utilize the Delta method, a powerful tool for approximating the variance of a function of a random variable. Let's assume we have an unbiased estimator T of θ with variance Var(T) ≥ 1/I(θ). We want to find the lower bound for the variance of an unbiased estimator of 1/θ.
- Apply the Delta Method: The Delta method states that if we have an estimator T of θ and a function g(θ), then the variance of g(T) can be approximated by:
Var[g(T)] ≈ [g'(θ)]² Var(T)
where g'(θ) is the derivative of g(θ) with respect to θ. In our case, g(θ) = 1/θ, so g'(θ) = -1/θ².
- Substitute and Simplify: Substituting into the approximation above, we get:
Var[g(T)] ≈ (-1/θ²)² Var(T) = (1/θ⁴) Var(T)
- Apply the RCLB for θ: Since Var(T) ≥ 1/I(θ), we can substitute this inequality into the approximation:
Var[g(T)] ≈ (1/θ⁴) Var(T) ≥ (1/θ⁴) (1/I(θ)) = 1/[θ⁴ I(θ)]
Therefore, the Rao-Cramer Lower Bound for the variance of an unbiased estimator of 1/θ is approximately 1/[θ⁴ I(θ)].
Important Considerations:
-
Approximation: The Delta method provides an approximation. The accuracy of this approximation depends on the sample size and the behavior of the estimator T. For large sample sizes, the approximation is generally quite good.
-
Bias: The derivation assumes an unbiased estimator for θ. If the estimator for θ is biased, adjustments must be made.
-
Regularity Conditions: The standard regularity conditions for the RCLB must be satisfied for the derivation to be valid. These typically include the existence of the first and second derivatives of the log-likelihood function.
-
Specific Distribution: The exact form of the RCLB for 1/θ will depend on the specific probability distribution from which the sample is drawn. You will need to calculate the Fisher information I(θ) for that distribution.
By following these steps and adapting them to your specific distribution, you can effectively determine the Rao-Cramer Lower Bound for 1/θ, providing a valuable benchmark for evaluating the efficiency of estimators for this function of the parameter. Remember to carefully consider the approximations involved and the assumptions underlying the RCLB.
Latest Posts
Latest Posts
-
Gravitational Pull Of A Black Hole
May 23, 2025
-
How To I Access Grub On Windows
May 23, 2025
-
What Does It Mean If The Groundhog Sees His Shadow
May 23, 2025
-
How Long Is A Life Sentence In Georgia
May 23, 2025
-
The Square Of Sum As Integral Examples
May 23, 2025
Related Post
Thank you for visiting our website which covers about Find A Rao Cramer Bound For 1/theta Instead Of Theta . We hope the information provided has been useful to you. Feel free to contact us if you have any questions or need further assistance. See you next time and don't miss to bookmark.