How To Calculate P Value For Categorical Data
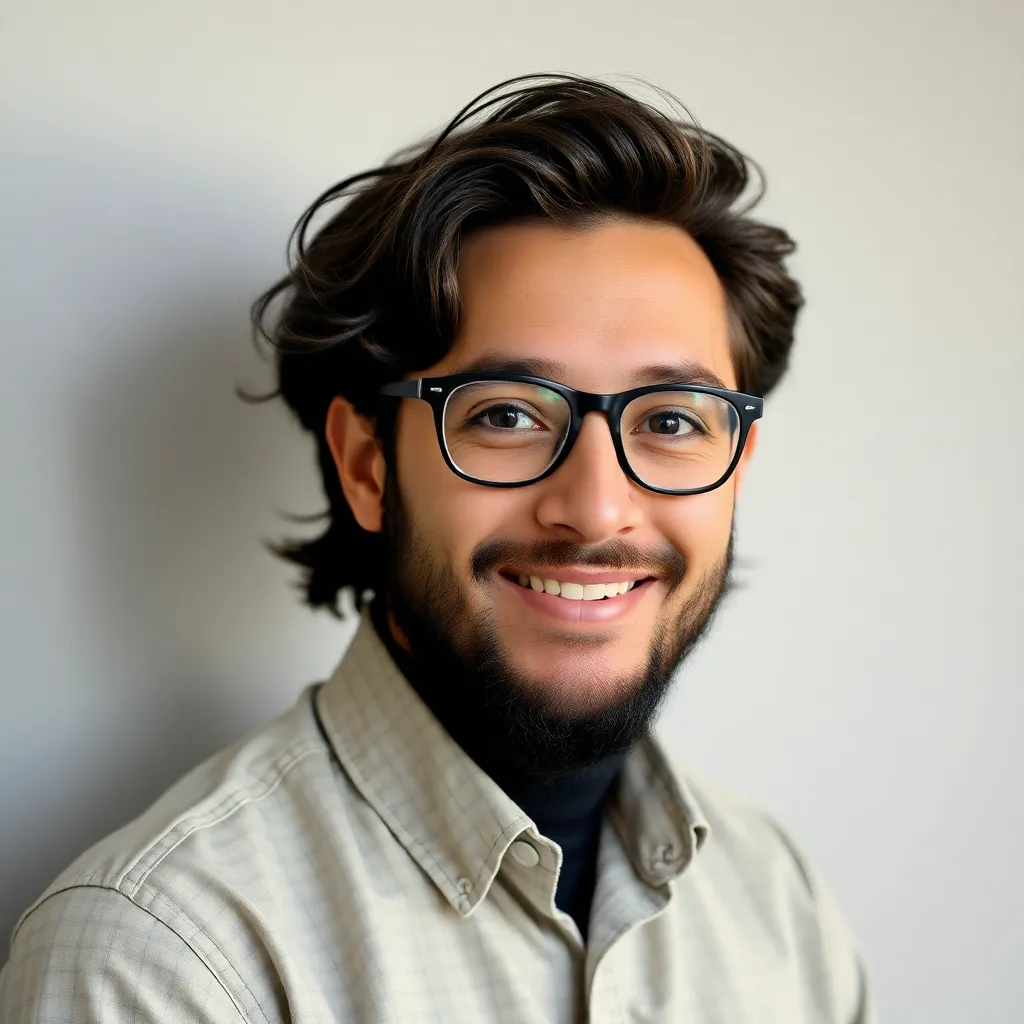
Kalali
May 24, 2025 · 4 min read
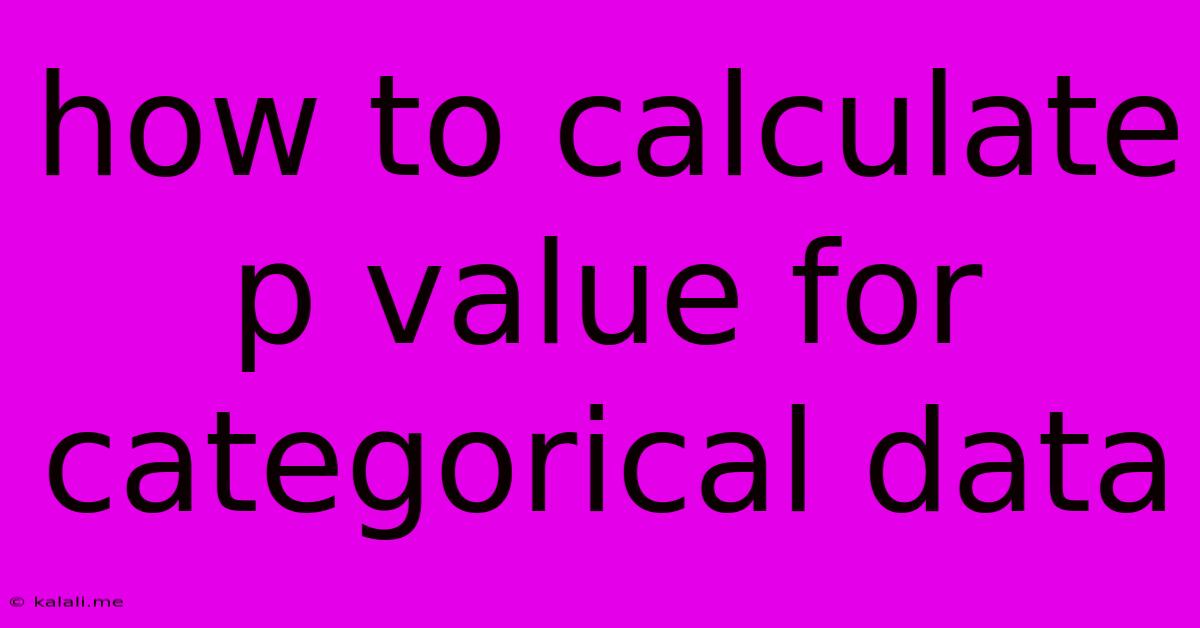
Table of Contents
How to Calculate the P-Value for Categorical Data
Calculating p-values for categorical data involves different statistical tests compared to continuous data. The choice of test depends heavily on the research question and the type of categorical variables involved. This article will guide you through common scenarios and the associated tests, explaining the concept of p-value in the context of categorical data. Understanding how to interpret these p-values is crucial for drawing valid conclusions from your analysis.
What is a P-value?
Before delving into specific tests, let's reiterate the meaning of a p-value. In simple terms, the p-value represents the probability of obtaining results as extreme as, or more extreme than, the observed results, assuming the null hypothesis is true. The null hypothesis typically states there's no significant difference or relationship between the variables. A small p-value (typically less than 0.05) suggests that the observed results are unlikely to have occurred by chance alone, leading us to reject the null hypothesis and conclude there's a statistically significant effect.
Common Statistical Tests for Categorical Data
Several statistical tests are used to analyze categorical data, leading to the calculation of a p-value. Here are some of the most frequently used:
1. Chi-Square Test:
The Chi-Square test is used to analyze the association between two categorical variables. It determines if there's a statistically significant difference between the observed frequencies and the expected frequencies if the variables were independent.
- Scenario: You want to investigate whether there's a relationship between smoking habits (smoker/non-smoker) and lung cancer diagnosis (yes/no).
- How it works: The Chi-square test compares the observed counts in each category to the expected counts under the assumption of independence. A large Chi-square statistic indicates a significant departure from independence. The associated p-value indicates the probability of observing such a departure if no real association exists.
- Software: Most statistical software packages (R, SPSS, SAS, Python with SciPy) can easily perform Chi-square tests.
2. Fisher's Exact Test:
Fisher's Exact Test is an alternative to the Chi-square test, particularly useful when dealing with small sample sizes (expected cell counts less than 5). It provides a more accurate p-value in these situations.
- Scenario: Investigating the relationship between a rare genetic mutation (present/absent) and a specific disease (present/absent) in a small sample of patients.
- How it works: Fisher's Exact Test calculates the exact probability of observing the obtained contingency table or a more extreme one under the null hypothesis of independence.
- Software: Similarly available in most statistical software packages.
3. McNemar's Test:
McNemar's Test is used for paired nominal data, often in before-and-after studies. It assesses the difference in proportions between two related categorical variables.
- Scenario: Evaluating the effectiveness of a new treatment by comparing the proportion of patients who improved before and after treatment.
- How it works: It focuses on the discordant pairs (those where the outcome changed). A significant p-value suggests a significant change in proportions.
- Software: Readily implemented in statistical software.
4. Cochran's Q Test:
Cochran's Q Test is used to analyze the association between multiple related categorical variables (e.g., multiple raters or multiple time points).
- Scenario: Assessing agreement among three different doctors on the diagnosis of a particular condition for the same set of patients.
- How it works: It tests the null hypothesis that the proportion of successes is the same across all groups.
- Software: Statistical software packages provide functions for Cochran's Q test.
Interpreting the P-value:
Once you've performed the appropriate test, the p-value provides crucial information.
- P-value < 0.05 (or your chosen significance level): Reject the null hypothesis. There is statistically significant evidence to suggest a relationship or difference between the categorical variables.
- P-value ≥ 0.05: Fail to reject the null hypothesis. There is not enough evidence to suggest a statistically significant relationship or difference.
Important Considerations:
- Significance level: The significance level (alpha) is typically set at 0.05, but this can be adjusted depending on the context of the study.
- Effect size: While the p-value indicates statistical significance, it doesn't necessarily reflect the practical significance or effect size. Consider effect size measures alongside the p-value for a complete understanding.
- Assumptions: Each test has underlying assumptions (e.g., independence of observations for Chi-square). Verify these assumptions before interpreting the results.
By understanding the different statistical tests and how to interpret their p-values, you can confidently analyze categorical data and draw meaningful conclusions from your research. Remember to choose the appropriate test based on your research question and the nature of your data. Always consult statistical resources and consider seeking expert advice if needed.
Latest Posts
Latest Posts
-
Double Pole 30 Amp Breaker For Dryer
May 25, 2025
-
Over The Range Microwave No Cabinet
May 25, 2025
-
How To Tell What Version Of Minecraft You Have
May 25, 2025
-
Substitute For Dry Milk Powder In Bread
May 25, 2025
-
Where Did Moses Write Of Jesus
May 25, 2025
Related Post
Thank you for visiting our website which covers about How To Calculate P Value For Categorical Data . We hope the information provided has been useful to you. Feel free to contact us if you have any questions or need further assistance. See you next time and don't miss to bookmark.