Results Of Convergence Of Empirical Distribution To True Distribution
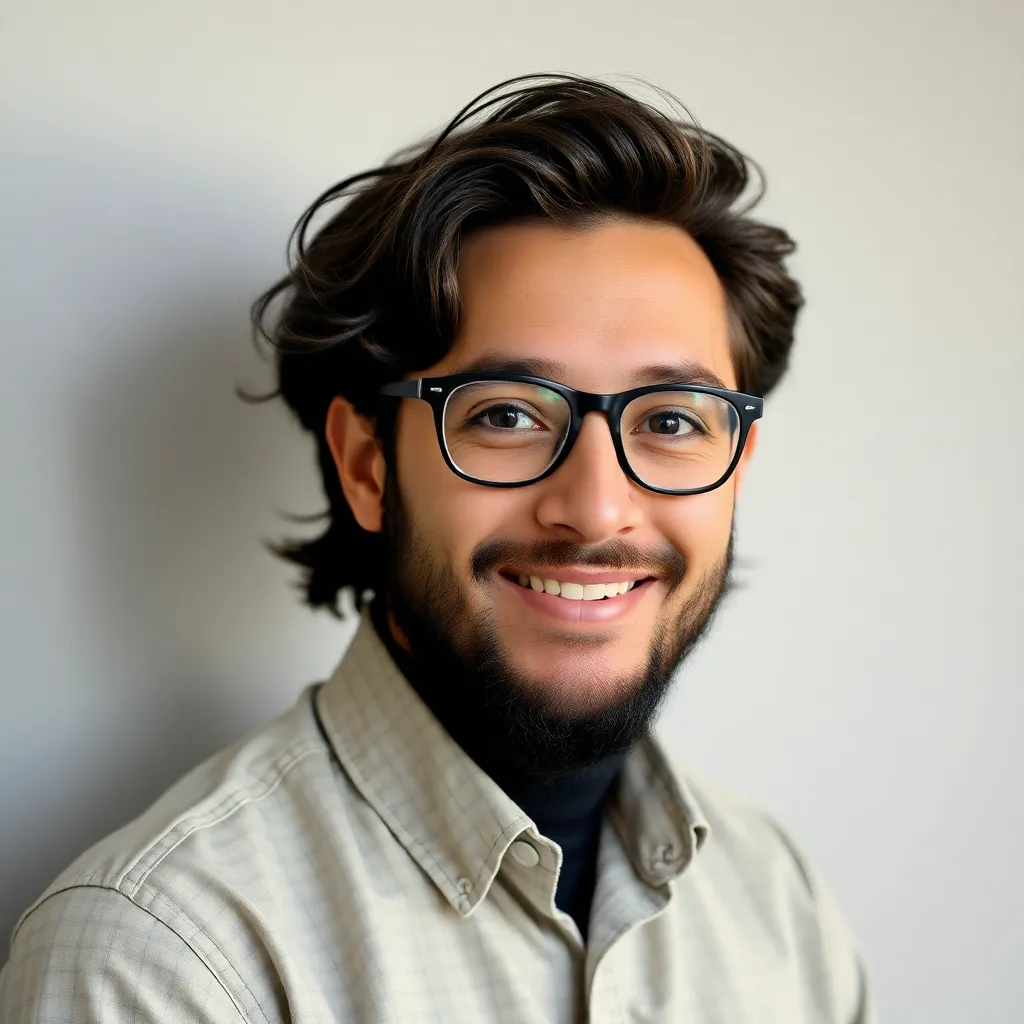
Kalali
May 25, 2025 · 3 min read
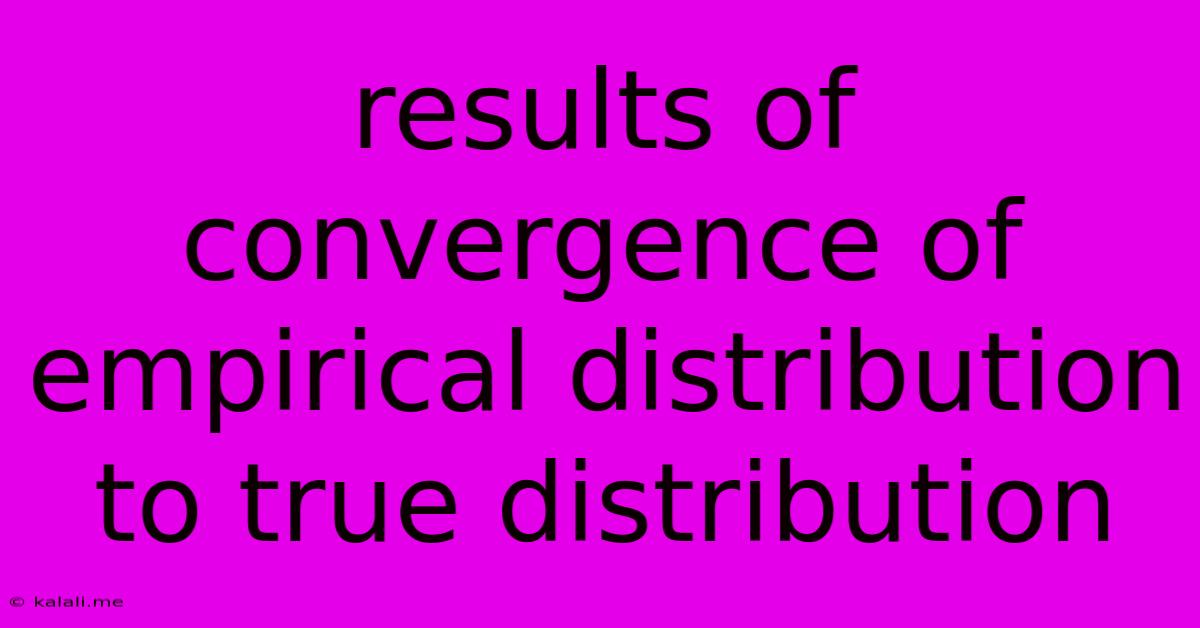
Table of Contents
The Convergence of Empirical Distributions to True Distributions: A Deep Dive
This article explores the crucial concept of the convergence of empirical distributions to their underlying true distributions. Understanding this convergence is fundamental to statistical inference, machine learning, and various data-driven applications. We'll examine the theoretical underpinnings, practical implications, and limitations of this vital statistical principle.
What is an Empirical Distribution?
Before delving into convergence, let's define our terms. An empirical distribution is a probability distribution derived from observed data. Imagine you're surveying the heights of 100 people. The empirical distribution would represent the observed distribution of those 100 heights – how many are tall, how many are short, and the frequency of each height measurement. This contrasts with the true distribution, which represents the actual, underlying probability distribution of heights in the entire population (not just your sample of 100).
The Law of Large Numbers: The Foundation of Convergence
The cornerstone of empirical distribution convergence is the Law of Large Numbers (LLN). The LLN states that as the sample size (number of observations) increases, the sample average (mean) converges to the true population mean. This isn't just limited to the mean; it extends to other characteristics of the distribution, such as variance, skewness, and quantiles. In essence, the empirical distribution becomes an increasingly accurate representation of the true distribution as more data is collected.
Different Types of Convergence
Several types of convergence define how the empirical distribution approaches the true distribution:
-
Convergence in Probability: This implies that the probability of the empirical distribution differing significantly from the true distribution approaches zero as the sample size increases. It's a weaker form of convergence.
-
Almost Sure Convergence: This is a stronger form, stating that the empirical distribution converges to the true distribution with probability one. This essentially means that the convergence is guaranteed, except for events with probability zero.
-
Convergence in Distribution: This describes the convergence of the cumulative distribution functions (CDFs) of the empirical and true distributions. It's important for approximating the probability of events using the empirical distribution.
Practical Implications and Applications
The convergence of empirical distributions underpins many statistical techniques:
-
Estimation: We use sample statistics (calculated from the empirical distribution) to estimate population parameters (characteristics of the true distribution). The LLN assures us that these estimates become increasingly accurate with larger sample sizes.
-
Hypothesis Testing: Many statistical tests rely on the assumption that the empirical distribution accurately reflects the true distribution. The convergence guarantees that this assumption becomes increasingly valid with more data.
-
Machine Learning: Training machine learning models often involves estimating parameters from training data. The convergence principle guides our understanding of how well the model generalizes to unseen data, improving accuracy and reducing overfitting.
-
Monte Carlo Simulations: These simulations rely on generating random samples from an assumed distribution. The convergence of empirical distributions allows us to use these samples to approximate the behavior of complex systems.
Limitations and Considerations
While powerful, the convergence of empirical distributions is not without limitations:
-
Sample Bias: If the data sample isn't representative of the true population (due to sampling bias), the empirical distribution might never accurately reflect the true distribution, no matter how large the sample size becomes.
-
Infinite Populations: While the LLN deals with increasing sample size, it doesn't guarantee perfect accuracy, especially with infinite population sizes.
Conclusion
The convergence of empirical distributions to their true counterparts is a cornerstone of statistical inference. Understanding the various types of convergence, their implications, and their limitations is critical for effectively analyzing data, building statistical models, and making informed decisions based on data-driven insights. While practical limitations exist, the Law of Large Numbers provides a robust theoretical foundation for this essential concept in statistics and related fields.
Latest Posts
Latest Posts
-
Bash Read Lines Into Multiple Variables
May 25, 2025
-
How To Loosen A Wheel Nut
May 25, 2025
-
Stepper Motor Driver Soem Does Not Work Control Frequency
May 25, 2025
-
Check The External Field Of The Error Object
May 25, 2025
-
Can You Use Linseed Oil Over Painted Wood
May 25, 2025
Related Post
Thank you for visiting our website which covers about Results Of Convergence Of Empirical Distribution To True Distribution . We hope the information provided has been useful to you. Feel free to contact us if you have any questions or need further assistance. See you next time and don't miss to bookmark.