The Mean Of The Sample Means
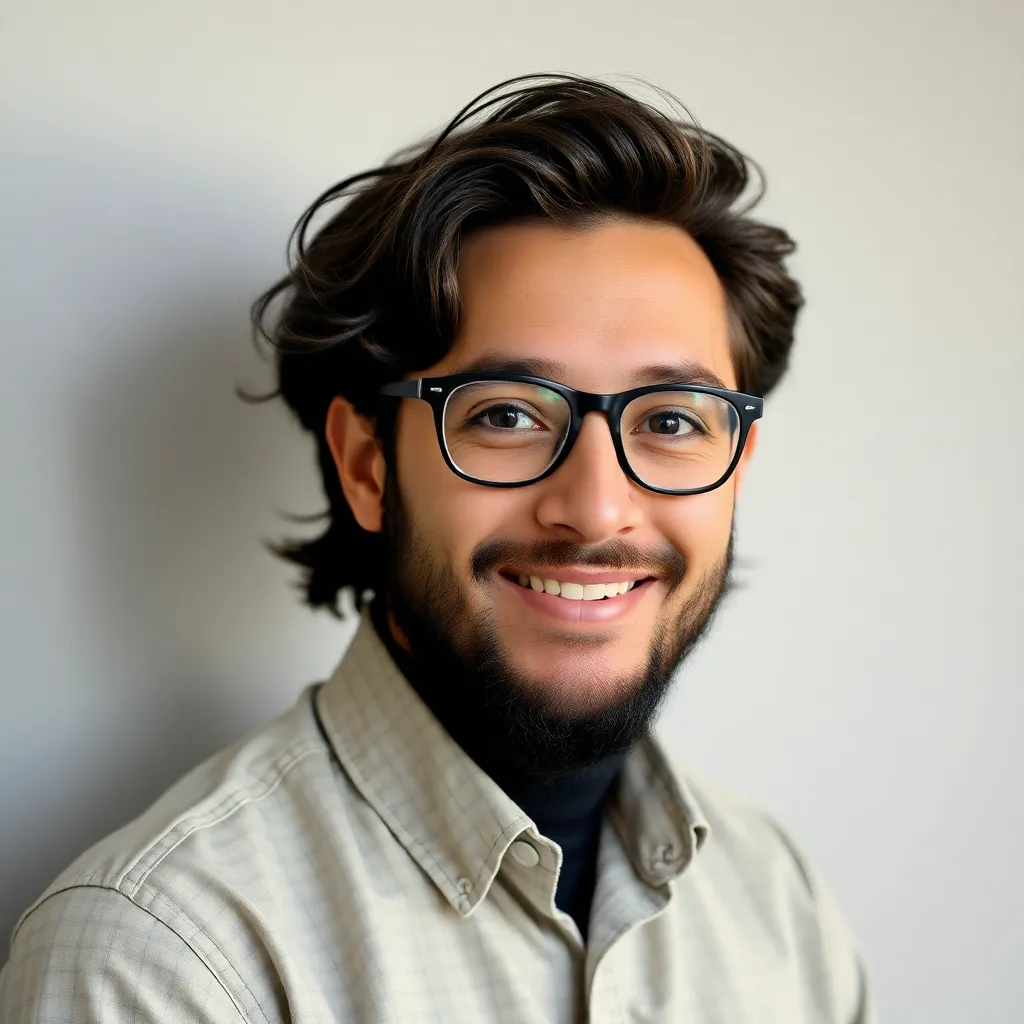
Kalali
Apr 17, 2025 · 7 min read
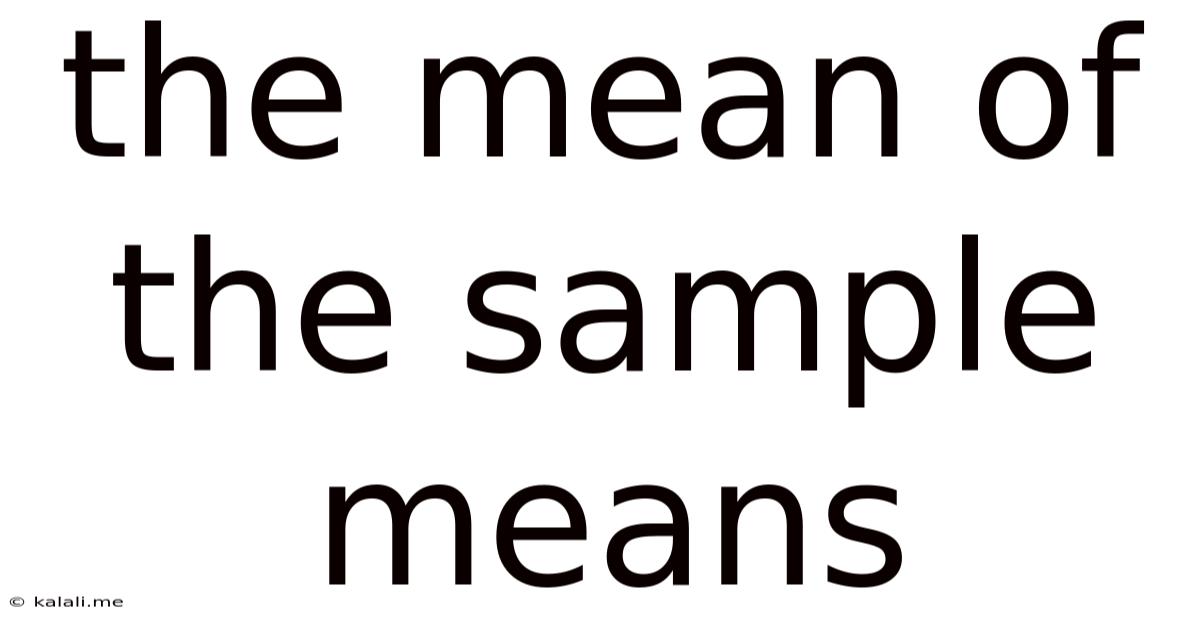
Table of Contents
Understanding the Mean of the Sample Means: A Deep Dive into Statistical Inference
The mean of the sample means, also known as the expected value of the sample mean or the mean of the sampling distribution of the mean, is a fundamental concept in statistical inference. It's a cornerstone of understanding how sample data can be used to make inferences about a larger population. This article provides a comprehensive explanation, exploring its meaning, calculation, applications, and importance in various statistical analyses. Understanding this concept is crucial for interpreting statistical results accurately and making informed decisions based on data analysis.
What is the Mean of the Sample Means?
Imagine you're interested in the average height of all students at a large university. Measuring every student is impractical, so you take several random samples of students, measure their heights, and calculate the mean height for each sample. The mean of all these sample means is the mean of the sample means. It's essentially the average of the averages.
More formally, the mean of the sample means is the expected value of the sample mean, denoted as E(x̄). It represents the average of all possible sample means that could be obtained from a population. Under certain conditions (primarily when samples are randomly selected and sufficiently large), this mean of the sample means is an unbiased estimator of the population mean (μ). This means, on average, the mean of the sample means will be very close to the true population mean.
Why is the Mean of the Sample Means Important?
The significance of the mean of the sample means stems from its central role in the Central Limit Theorem (CLT). The CLT states that, regardless of the shape of the population distribution, the sampling distribution of the mean approaches a normal distribution as the sample size increases. This is true even if the original population data is not normally distributed. This is a powerful result that greatly simplifies statistical inference.
The mean of this approximately normal sampling distribution is equal to the population mean (μ). Therefore, by understanding the mean of the sample means, we can use sample data to make inferences about the population mean with a known distribution and associated probabilities. This is the foundation of hypothesis testing and confidence interval estimation.
Calculating the Mean of the Sample Means
The calculation of the mean of the sample means is straightforward. If you have 'k' independent samples, each with its own sample mean (x̄₁, x̄₂, ..., x̄ₖ), then the mean of the sample means is simply the average of these sample means:
E(x̄) = (x̄₁ + x̄₂ + ... + x̄ₖ) / k
However, in practice, you rarely calculate this directly. The power of the mean of the sample means lies in its theoretical relationship to the population mean. The crucial point is that, under random sampling, E(x̄) = μ. This allows us to estimate the population mean (μ) using the sample mean (x̄) from a single, sufficiently large sample.
The Central Limit Theorem and its Implications
The Central Limit Theorem (CLT) is intrinsically linked to the mean of the sample means. The CLT doesn't just guarantee that the sampling distribution of the mean is approximately normal for large sample sizes; it also specifies the mean and standard deviation of this distribution. This is crucial for statistical inference:
- Mean: The mean of the sampling distribution of the mean (E(x̄)) is equal to the population mean (μ). This means the sample means tend to cluster around the true population mean.
- Standard Deviation: The standard deviation of the sampling distribution of the mean, known as the standard error of the mean (SEM), is given by σ/√n, where σ is the population standard deviation and n is the sample size. The SEM quantifies the variability of the sample means. As the sample size (n) increases, the SEM decreases, implying that the sample means become more tightly clustered around the population mean. This is why larger sample sizes lead to more precise estimations of the population mean.
Applications of the Mean of the Sample Means
The concept of the mean of the sample means has widespread applications in various fields:
-
Hypothesis Testing: We use the mean of the sample means (and its associated standard error) to test hypotheses about population means. For instance, we might test whether the average income in a city is significantly different from the national average. The sample mean provides the estimate, and the standard error allows us to determine the statistical significance of the difference.
-
Confidence Intervals: Confidence intervals provide a range of values within which the true population mean is likely to fall. The mean of the sample means and the standard error are used to construct these confidence intervals. A 95% confidence interval, for example, means that we are 95% confident that the true population mean lies within the calculated range.
-
Quality Control: In manufacturing and other industries, the mean of the sample means is crucial for monitoring product quality. By repeatedly sampling products and calculating the mean of a particular characteristic (like weight or length), manufacturers can track whether production is consistent with desired specifications. Deviations from the expected mean of the sample means may signal problems in the production process.
-
Polling and Surveys: Public opinion polls and surveys rely heavily on the principles of sampling and the mean of the sample means. The pollsters use the mean of the sample responses to estimate the proportion of the population holding a particular view. The standard error of this mean is used to determine the margin of error associated with the poll results.
-
Medical Research: Clinical trials often use the mean of the sample means to compare the effectiveness of different treatments. By measuring the average outcome (like blood pressure or tumor size) in treatment and control groups, researchers can determine whether a new treatment is significantly better than an existing one.
Understanding Standard Error and Sample Size
The standard error of the mean (SEM) is a critical factor in understanding the accuracy of our estimate of the population mean. A smaller SEM indicates a more precise estimate. The SEM is directly influenced by the sample size:
-
Larger Sample Size: A larger sample size leads to a smaller SEM. This is because larger samples provide more information about the population, resulting in a more precise estimate of the population mean. The sample means become more tightly clustered around the true mean.
-
Smaller Sample Size: A smaller sample size leads to a larger SEM. This means the sample means are more spread out, resulting in a less precise estimate of the population mean. There's more uncertainty about the true value.
Assumptions and Limitations
While the mean of the sample means is a powerful tool, it's crucial to be aware of its underlying assumptions and limitations:
-
Random Sampling: The samples must be randomly selected from the population. Non-random sampling can introduce bias and invalidate the results.
-
Independence: The samples should be independent of each other. This means the selection of one sample should not influence the selection of another.
-
Sample Size: While the CLT works well with reasonably large sample sizes, the approximation to normality may not be accurate for very small samples. In these cases, different statistical methods may be necessary.
-
Population Variance: The standard error calculation requires knowledge of the population standard deviation (σ). In many real-world scenarios, the population standard deviation is unknown. In such cases, the sample standard deviation (s) is often used as an estimate, leading to the use of the t-distribution instead of the normal distribution for inference.
Conclusion:
The mean of the sample means is a vital concept in statistics, providing a bridge between sample data and population parameters. Its role in the Central Limit Theorem allows for inference about population means even when the underlying population distribution is unknown or non-normal. Understanding this concept is essential for interpreting statistical results accurately and making informed decisions based on data. Its applications are extensive across diverse fields, underscoring its importance in data analysis and decision-making processes. By understanding the assumptions and limitations, researchers and analysts can effectively leverage this powerful tool for sound statistical inference.
Latest Posts
Latest Posts
-
9 As A Percentage Of 25
Apr 19, 2025
-
What Is 1 12 In A Decimal
Apr 19, 2025
-
Cuanto Es 39 Fahrenheit En Celsius
Apr 19, 2025
-
3 6 Amino Acids Per One Alpha Helix Turn
Apr 19, 2025
-
Is The Sun Biotic Or Abiotic
Apr 19, 2025
Related Post
Thank you for visiting our website which covers about The Mean Of The Sample Means . We hope the information provided has been useful to you. Feel free to contact us if you have any questions or need further assistance. See you next time and don't miss to bookmark.