Why Cant You Do Confidence Interval For Convenience Sample
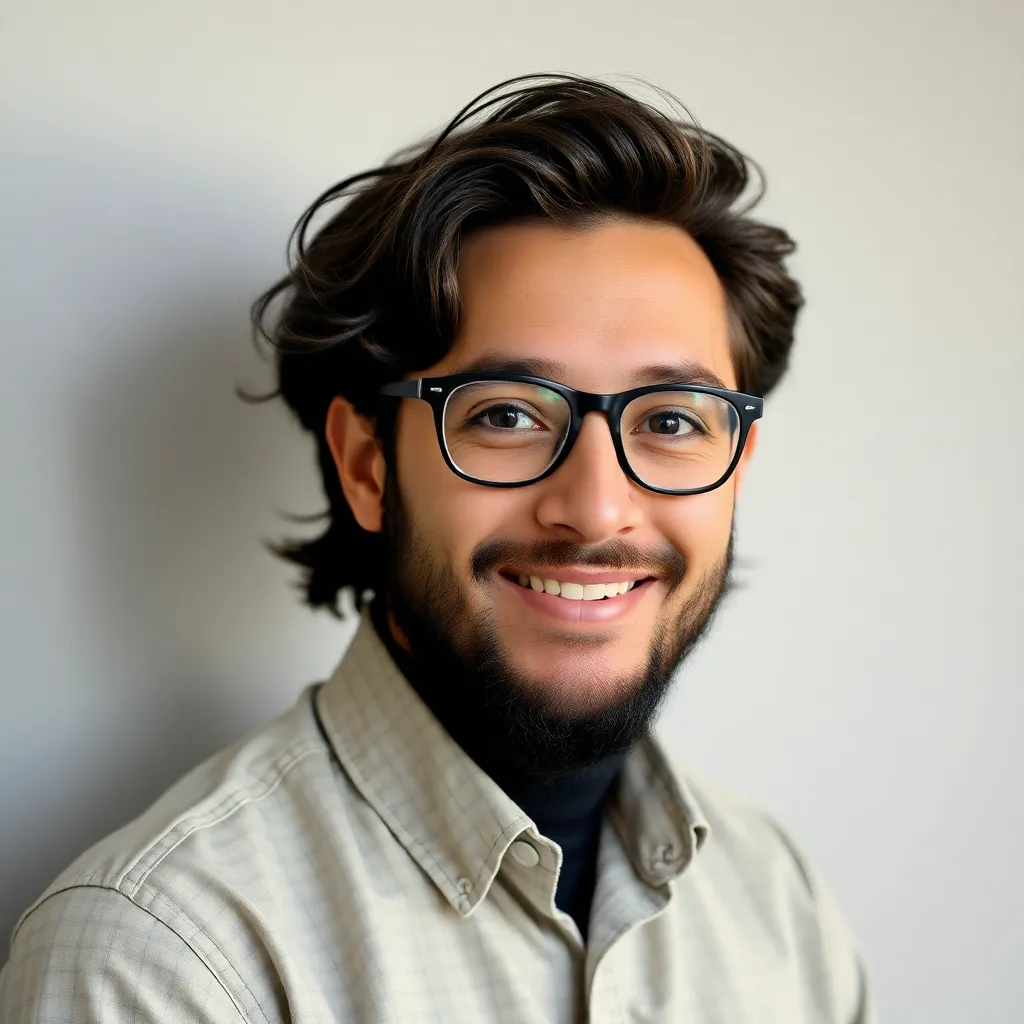
Kalali
May 23, 2025 · 3 min read
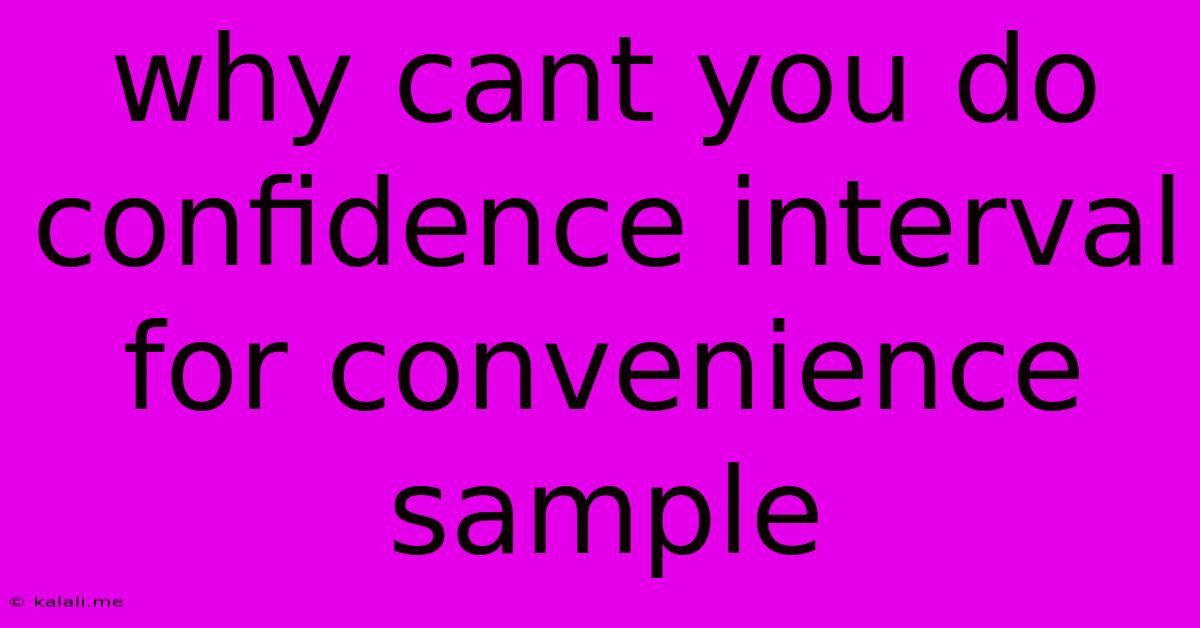
Table of Contents
Why You Can't (and Shouldn't) Calculate Confidence Intervals with Convenience Samples
Confidence intervals are a cornerstone of statistical inference, providing a range of plausible values for a population parameter based on sample data. However, a critical assumption underlying the validity of a confidence interval is that the sample is representative of the population. This is where convenience samples fall short, making confidence interval calculation inappropriate and potentially misleading. This article will delve into why you should avoid calculating confidence intervals from convenience samples and explore the underlying statistical reasons.
What is a Convenience Sample?
A convenience sample is a type of non-probability sampling where participants are selected based on their ease of accessibility. Researchers often opt for convenience samples because they are quick, inexpensive, and require minimal effort. Examples include surveying friends, family, or colleagues, or using readily available data sets without careful consideration of the population they represent. This ease of access, however, comes at a significant cost to the representativeness of the sample.
Why Confidence Intervals Fail with Convenience Samples
The fundamental problem with using convenience samples for confidence intervals lies in the inherent bias. Because participants are selected based on convenience rather than random selection, the sample is unlikely to accurately reflect the characteristics of the population of interest. This bias leads to several issues:
-
Unrepresentative Sample: Convenience samples inherently overrepresent easily accessible groups and underrepresent those who are harder to reach. This skewed representation leads to biased estimates of population parameters. A confidence interval calculated from such a sample will not accurately capture the true population parameter.
-
Unknown Sampling Distribution: Standard methods for calculating confidence intervals rely on the assumption of a known or estimable sampling distribution (often assumed to be normal, especially for large samples). With a convenience sample, the true sampling distribution is unknown and likely non-random, violating this crucial assumption. Therefore, any statistical inferences based on it are unreliable.
-
Inaccurate Standard Error: The standard error, a crucial component in confidence interval calculation, estimates the variability of the sample statistic. With a convenience sample, the standard error calculation is flawed because it's based on a non-representative sample, leading to an inaccurate estimate of the variability and, consequently, an inaccurate confidence interval.
-
Violation of Random Sampling Assumption: Many statistical tests, including those underlying confidence interval calculations, rest on the assumption of random sampling. Convenience sampling directly violates this assumption. The results, therefore, cannot be generalized to the broader population.
Alternatives to Convenience Samples for Robust Inference:
If you need to construct a valid confidence interval, you must employ probability sampling methods that ensure every member of the population has a known, non-zero chance of being selected. These include:
-
Simple Random Sampling: Every individual in the population has an equal chance of being selected.
-
Stratified Random Sampling: The population is divided into strata (subgroups), and random samples are drawn from each stratum.
-
Cluster Sampling: The population is divided into clusters, and a random sample of clusters is selected. All individuals within the selected clusters are then included in the sample.
Conclusion:
While convenience samples may offer practical advantages in terms of time and cost, their inherent biases render them unsuitable for calculating confidence intervals. The resulting confidence interval will not be a reliable representation of the population parameter and could lead to erroneous conclusions. Always prioritize probability sampling techniques to ensure the validity and reliability of statistical inferences, including confidence intervals. Choosing the right sampling method is paramount for drawing meaningful conclusions from your research.
Latest Posts
Latest Posts
-
Let Me Google It For You
May 23, 2025
-
Is It Buck Or Butt Naked
May 23, 2025
-
Moment Of Inertia For A Solid Sphere
May 23, 2025
-
Power Automate Get All Values From A Specific Column
May 23, 2025
-
How To Bypass Google Lock On Motorola
May 23, 2025
Related Post
Thank you for visiting our website which covers about Why Cant You Do Confidence Interval For Convenience Sample . We hope the information provided has been useful to you. Feel free to contact us if you have any questions or need further assistance. See you next time and don't miss to bookmark.