Using Ordinal Measures In Multiple Regression
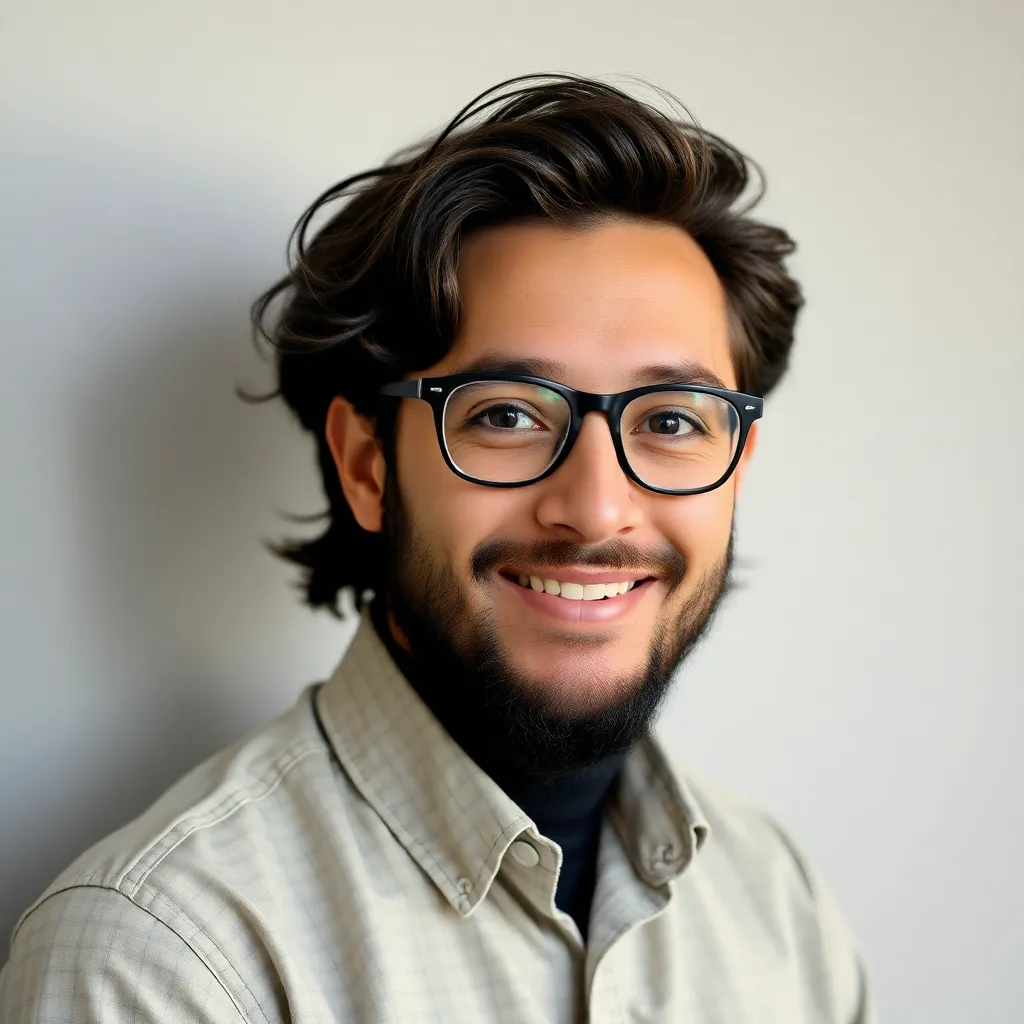
Kalali
May 23, 2025 · 4 min read
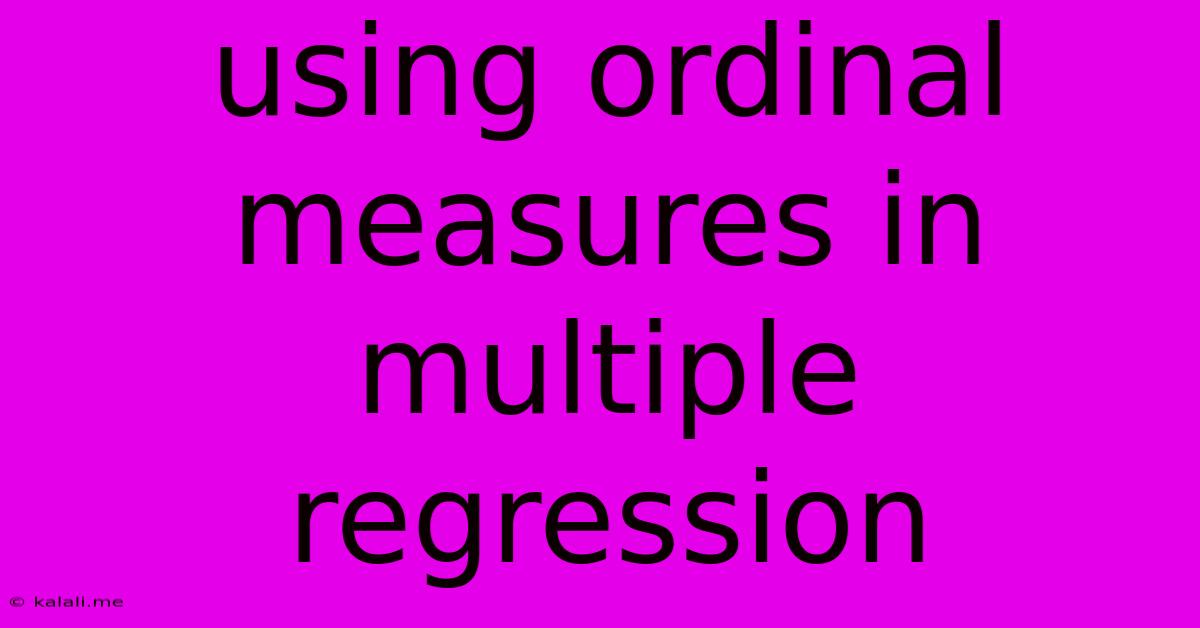
Table of Contents
Using Ordinal Measures in Multiple Regression: A Comprehensive Guide
Meta Description: Learn how to effectively incorporate ordinal measures into your multiple regression analysis. This guide covers various approaches, their strengths and weaknesses, and best practices for accurate and meaningful results. Discover how to handle ordinal data for improved statistical modeling.
Multiple regression analysis is a powerful statistical tool for examining the relationship between a dependent variable and multiple independent variables. However, the assumption of interval or ratio level data for independent variables often presents a challenge when dealing with ordinal data – data that represents rank order but not necessarily equal intervals between categories (e.g., Likert scales, educational attainment levels). This article explores effective strategies for incorporating ordinal measures in multiple regression, navigating the complexities and ensuring accurate interpretations.
Understanding Ordinal Data and its Challenges in Regression
Ordinal data, representing ranked categories, differs significantly from interval or ratio data. While it indicates order, the distances between categories aren't necessarily uniform. Directly including ordinal variables as predictors in standard multiple regression can lead to misinterpretations and biased results, violating the assumption of linearity.
Methods for Handling Ordinal Predictors in Multiple Regression
Several techniques exist to address the challenges posed by ordinal predictors in multiple regression. The best approach depends on the specific research question, data characteristics, and the desired level of complexity.
1. Dummy Coding (Binary Encoding):
This involves creating binary (0/1) dummy variables for each category of the ordinal variable except the reference category (usually the lowest). For instance, a five-level ordinal variable ("strongly disagree" to "strongly agree") would be represented by four dummy variables.
- Advantages: Simple to implement and widely understood.
- Disadvantages: It ignores the inherent order of categories, leading to potential loss of information and potentially less efficient models compared to other methods. It can also increase the number of predictors substantially if the ordinal variable has many categories.
2. Effect Coding:
Similar to dummy coding, effect coding creates dummy variables, but it assigns values that reflect the order and distance between categories (e.g., -2, -1, 0, 1, 2). This approach preserves more of the ordinal information than dummy coding.
- Advantages: Retains more ordinal information than dummy coding.
- Disadvantages: Still a simplification of the underlying ordinal nature of the variable, potentially less powerful than other methods. Interpretation might be slightly more complex.
3. Polytomous Logistic Regression (for ordinal dependent variable):
If your dependent variable is ordinal, polytomous logistic regression is a more appropriate model. This method accounts for the ordered nature of the outcome and provides estimates for the cumulative probability of being in a certain category or below.
- Advantages: Specifically designed for ordinal dependent variables, producing more accurate and interpretable results than standard linear regression.
- Disadvantages: Not suitable when the independent variable is ordinal.
4. Ordinal Regression (Proportional Odds Model):
This is a powerful technique explicitly designed for handling ordinal dependent variables. This model considers the cumulative odds of being in a particular category or a higher category.
- Advantages: Accounts for the ordered nature of the outcome variable. More powerful than methods ignoring ordinality.
- Disadvantages: Assumptions need to be checked (e.g., proportional odds assumption).
5. Non-parametric Methods:
For smaller datasets or when assumptions of other methods are violated, non-parametric techniques, such as rank-based regression, offer a robust alternative.
- Advantages: Less sensitive to violations of assumptions.
- Disadvantages: Less statistically powerful than parametric methods, may yield less precise estimates.
Choosing the Right Method
The selection of the appropriate method hinges on several factors:
- Nature of the ordinal variable: The number of categories, the spacing between categories, and the distribution of responses within the categories.
- Research question: The specific hypotheses being tested and the type of information required from the analysis.
- Dataset characteristics: The size of the sample, the presence of outliers, and the distribution of other variables in the model.
Interpreting Results and Reporting Findings
Regardless of the chosen method, meticulous interpretation of the results is crucial. Focus on the magnitude and statistical significance of the coefficients, paying attention to potential interactions and multicollinearity. Clearly articulate the chosen method and its limitations in the research report.
Conclusion
Incorporating ordinal measures in multiple regression necessitates careful consideration of the available methods. While simple approaches like dummy coding are readily available, methods like ordinal regression often provide more robust and informative analyses when dealing with ordinal predictors or outcomes. Selecting the appropriate technique and thoroughly interpreting the results are paramount to obtaining valid and meaningful conclusions from your regression analysis. Remember to always assess the assumptions of your chosen model and consider alternative methods if those assumptions are violated.
Latest Posts
Latest Posts
-
How To Reference Algorithm In Latex
May 23, 2025
-
Si There A Space Between Dr Ant The Name
May 23, 2025
-
Why Does My Toilet Run Randomly
May 23, 2025
-
No Matching Host Key Type Found
May 23, 2025
-
How To Finish Newly Installed Windows
May 23, 2025
Related Post
Thank you for visiting our website which covers about Using Ordinal Measures In Multiple Regression . We hope the information provided has been useful to you. Feel free to contact us if you have any questions or need further assistance. See you next time and don't miss to bookmark.